Kore.ai Sentiment Analysis
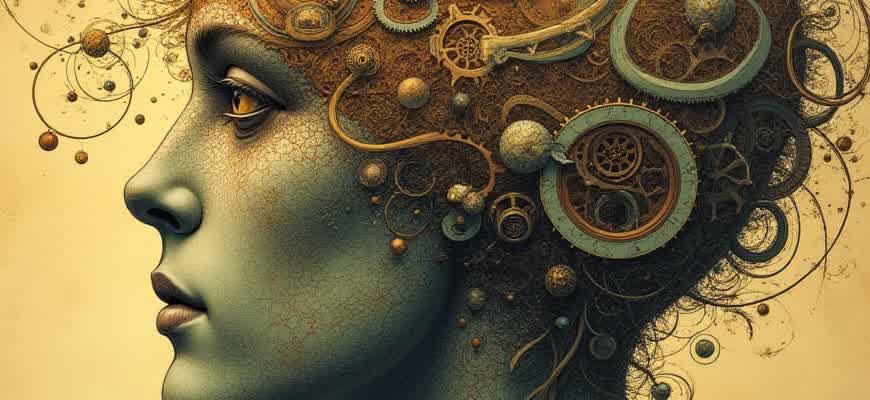
Kore.ai provides a robust sentiment analysis feature that helps businesses gauge user emotions in real-time. This technology leverages natural language processing (NLP) algorithms to detect and classify the sentiment behind user messages. It plays a crucial role in understanding customer interactions and improving service quality.
The system is capable of categorizing sentiments into different emotional states, which helps in making data-driven decisions. Sentiment analysis can be applied across various communication channels, ensuring that businesses can capture user feedback efficiently. Here are some key features:
- Real-time sentiment detection
- Multilingual support
- Integration with customer service platforms
"By analyzing sentiment, Kore.ai transforms raw user data into actionable insights, enabling businesses to respond proactively."
Sentiment analysis can be configured to classify user inputs into categories such as positive, negative, or neutral, providing a clear picture of customer satisfaction. Below is a sample breakdown of sentiment categories:
Sentiment | Percentage of Total Interactions |
---|---|
Positive | 45% |
Negative | 30% |
Neutral | 25% |
This level of analysis allows businesses to monitor and improve customer experience based on real-time feedback.
Sentiment Analysis with Kore.ai: A Practical Guide
Sentiment analysis in natural language processing (NLP) is essential for understanding user emotions and opinions from their text data. Kore.ai provides a robust framework for integrating sentiment analysis into your chatbot or virtual assistant. By using advanced machine learning techniques, Kore.ai helps identify positive, negative, or neutral sentiments in user interactions, which is crucial for improving customer engagement and support systems.
This guide walks you through setting up sentiment analysis using Kore.ai's platform. The steps below will help you configure the system, interpret sentiment results, and leverage them to enhance user experience. It is important to ensure that your chatbots are trained to detect nuances in user language, making interactions more meaningful and contextually aware.
Setting Up Sentiment Analysis in Kore.ai
Follow these key steps to enable sentiment analysis for your chatbot:
- Enable Sentiment Analysis Module: First, ensure that the sentiment analysis module is activated within your Kore.ai workspace.
- Define Intent and Entity Types: Tailor the sentiment analysis by categorizing intents and defining entities that are relevant to your business use case.
- Configure Sentiment Detection: Use the platform's settings to adjust the sensitivity of sentiment detection based on user feedback and interaction context.
- Test and Refine: Perform test runs to observe how the system processes different types of user input and refine the configuration accordingly.
Interpreting Sentiment Analysis Results
Once sentiment analysis is configured, the next step is understanding the output. Kore.ai provides several ways to assess and interpret sentiment from user input:
- Positive Sentiment: Indicates satisfaction or approval. Often found in messages containing positive feedback or gratitude.
- Negative Sentiment: Shows dissatisfaction, frustration, or complaints. It is essential for identifying potential issues or areas for improvement.
- Neutral Sentiment: Represents neutral or informational communication without strong emotional cues.
Example of Sentiment Output
User Input | Sentiment | Confidence Score |
---|---|---|
Great service, I’m happy with my purchase! | Positive | 98% |
I'm really upset with the delay in shipping. | Negative | 95% |
Can you help me track my order? | Neutral | 85% |
Effective sentiment analysis can dramatically improve user experience by proactively addressing issues, enhancing positive interactions, and tailoring responses accordingly.
Customizing Sentiment Models for Specific Industry Needs
When implementing sentiment analysis for specific industries, it's essential to tailor the models to understand the unique language and context of each field. A generic sentiment model may fail to capture the nuances that are critical in specialized sectors like healthcare, finance, or retail. Therefore, adjusting the model to reflect industry-specific vocabulary, customer concerns, and typical interactions ensures that the analysis is accurate and actionable.
Industries often use specific jargon or deal with unique customer emotions that a general sentiment model may not interpret correctly. Customizing sentiment models requires deep knowledge of the domain and collaboration between data scientists and industry experts to refine the model's understanding. This process helps businesses leverage the full potential of sentiment analysis tools for decision-making and customer insights.
Approaches for Customization
- Incorporating Industry-Specific Vocabulary: Adjusting the model to recognize specialized terms and expressions improves its understanding of user sentiment. For example, medical terminology in healthcare or financial jargon in the banking sector requires model adaptation.
- Contextual Sentiment Understanding: Sentiment analysis models can be enhanced by integrating industry-specific contextual cues. In customer service for retail, recognizing frustration over stock shortages is different from the same sentiment in the context of medical services.
- Feedback Loops: Regularly updating the model based on new data and evolving industry trends ensures the model remains relevant and continues to perform effectively in real-world scenarios.
Example of Customization in Different Industries
Industry | Customization Focus | Outcome |
---|---|---|
Healthcare | Recognizing patient sentiment towards treatment options, doctors, and hospital experiences | Improved patient satisfaction analysis and better healthcare delivery |
Retail | Understanding emotions related to product quality, delivery, and customer service | Optimized customer experience and targeted marketing strategies |
Finance | Detecting customer feelings about financial products, trust in banks, and economic conditions | More accurate sentiment reporting for market analysis and risk assessment |
Customizing sentiment models for specific industries ensures that businesses are not only gathering accurate data but also translating that data into actionable insights that directly align with industry goals and customer expectations.
Real-Time Tracking of Customer Sentiment with Kore.ai
In today’s competitive environment, businesses need to stay ahead of customer needs and concerns in real time. Kore.ai provides an advanced sentiment analysis solution that allows businesses to monitor customer feedback and interactions continuously. This capability ensures that companies can react promptly to negative sentiments, enhancing customer satisfaction and loyalty.
With Kore.ai’s sentiment analysis, businesses can gain actionable insights into customer emotions, preferences, and overall satisfaction. By integrating this tool into their customer service platforms, organizations can immediately track and address any emerging issues, providing an enhanced customer experience.
How Kore.ai Analyzes Customer Sentiment
The platform uses natural language processing (NLP) and machine learning algorithms to evaluate the tone and sentiment of customer interactions. Here are the key features of Kore.ai's real-time monitoring system:
- Continuous Sentiment Tracking: It automatically tracks and analyzes each customer interaction, regardless of the channel, in real time.
- Instant Alerts: When negative sentiment is detected, businesses are notified instantly to address the issue before it escalates.
- Sentiment Trends: The system highlights emerging trends in customer sentiment, allowing businesses to adjust their strategies accordingly.
- Detailed Analytics: Provides in-depth analytics on customer sentiment, identifying patterns and areas for improvement.
How It Works
- Customer Interaction: Customers engage with the business through various channels (e.g., chat, voice, emails).
- Sentiment Detection: Kore.ai’s algorithms analyze the content of these interactions, detecting underlying emotions such as joy, frustration, or disappointment.
- Real-Time Insights: The sentiment data is processed in real time, providing businesses with up-to-date feedback on customer emotions.
- Actionable Response: Based on the sentiment analysis, businesses can modify their approach, improve service quality, or address concerns promptly.
Benefits of Real-Time Sentiment Monitoring
The integration of real-time sentiment tracking into a business's customer service framework provides several key advantages:
Benefit | Description |
---|---|
Proactive Issue Resolution | By identifying negative sentiments early, businesses can address problems before they escalate, improving customer retention. |
Improved Customer Experience | Monitoring sentiment ensures businesses respond to customer needs with empathy, enhancing overall satisfaction. |
Data-Driven Decisions | Real-time insights enable businesses to make informed decisions based on customer emotions and trends. |
"Real-time sentiment monitoring empowers businesses to take immediate action, ensuring customer concerns are addressed as they arise."
Leveraging Sentiment Insights for Enhanced Customer Support
Sentiment analysis is an effective tool for improving customer support by offering valuable insights into how customers feel about products, services, or interactions. By analyzing customer feedback in real-time, support teams can understand the emotional tone behind each inquiry, allowing for more tailored responses and efficient issue resolution. This enables organizations to detect negative sentiment early and act swiftly to prevent dissatisfaction from escalating.
With the integration of sentiment analysis, companies can enhance their customer service by prioritizing cases based on urgency, identifying common pain points, and providing more personalized experiences. By continuously analyzing customer interactions, support teams can gain deeper insights into customer preferences, leading to improved service quality and better customer retention rates.
Key Benefits of Sentiment Insights in Customer Support
- Early Detection of Customer Frustration: Identifying negative sentiment allows teams to address issues before they escalate into major problems.
- Improved Response Personalization: Understanding customer emotions leads to more tailored, empathetic responses, enhancing the overall support experience.
- Optimized Resource Allocation: Prioritize cases with high negative sentiment, ensuring that critical issues are handled first.
- Real-time Feedback Loop: Continuous sentiment analysis enables a proactive approach, leading to faster issue resolution and increased customer satisfaction.
Steps to Implement Sentiment Insights Effectively
- Integrate Sentiment Analysis Tools: Incorporate sentiment detection systems into your customer support platform to analyze incoming messages in real-time.
- Train Support Teams: Equip customer service representatives with the knowledge to interpret sentiment data and respond appropriately.
- Use Data to Drive Improvements: Regularly analyze sentiment trends to identify areas of improvement in products or services.
- Measure Success: Track customer satisfaction and resolution time metrics to assess the impact of sentiment-driven changes.
By using sentiment insights effectively, companies can not only enhance their customer service quality but also foster stronger relationships with customers through timely and meaningful interactions.
Sentiment Analysis Impact: A Case Study
Metric | Before Sentiment Analysis | After Sentiment Analysis |
---|---|---|
Average Response Time | 12 hours | 4 hours |
Customer Satisfaction Score | 70% | 85% |
Escalation Rate | 25% | 10% |
Optimizing Marketing Campaigns with Sentiment Insights from Kore.ai
In today’s competitive market, understanding customer emotions and sentiments is crucial to crafting effective marketing campaigns. By leveraging sentiment data from platforms like Kore.ai, businesses can gain deep insights into how customers feel about their products or services, allowing them to fine-tune their marketing strategies for maximum engagement. The ability to analyze sentiment in real-time gives marketers a distinct advantage in responding quickly to shifts in customer perception and behavior.
Utilizing sentiment data allows companies to identify trends, predict customer responses, and optimize messaging. By recognizing positive, negative, and neutral sentiment, marketing teams can tailor their approaches to specific audience segments and enhance customer interaction. With the power of Kore.ai's sentiment analysis, businesses can refine targeting efforts and create campaigns that resonate more effectively with their audience.
Key Steps for Campaign Optimization Using Sentiment Data
- Sentiment Tracking: Continuously monitor customer sentiments across different platforms (social media, surveys, customer feedback) to stay updated on audience reactions.
- Audience Segmentation: Divide audiences based on sentiment scores to tailor personalized campaigns that cater to their emotional state.
- Real-time Adjustments: Modify campaign elements (messaging, visuals, offers) based on sentiment shifts during the campaign’s lifecycle.
Practical Applications in Marketing
Effective sentiment analysis can help optimize key areas of a marketing campaign:
- Content Creation: Create content that speaks to the emotions of your audience, whether it’s addressing concerns or reinforcing positive feelings.
- Customer Engagement: Respond to customer feedback in a way that shows understanding and empathy, fostering stronger relationships.
- Brand Positioning: Align brand messages with the prevailing sentiment of your target demographic to enhance relevance and brand loyalty.
Sentiment Data vs. Traditional Metrics
Sentiment Data | Traditional Metrics |
---|---|
Real-time emotional insights for immediate adjustments in strategy. | Performance indicators like CTR and conversions, typically measured post-campaign. |
Predictive analysis of customer reactions before launch. | Historical data based on past behaviors. |
Detailed audience sentiment breakdown that helps in creating personalized marketing efforts. | General metrics that don’t reveal customer emotional drivers. |
Incorporating sentiment analysis into marketing campaigns not only enhances customer engagement but also allows for greater adaptability and personalization, ultimately leading to better outcomes.
Analyzing Feedback Data: Transforming Sentiment into Actionable Insights
Feedback analysis is a crucial aspect of understanding customer experiences, especially when utilizing sentiment analysis. With the ability to gauge customer emotions through text, companies can uncover critical patterns that would otherwise go unnoticed. The key is to transform raw sentiment data into actionable insights that drive decision-making, product improvements, and service enhancements.
By integrating sentiment analysis with advanced analytics platforms like Kore.ai, businesses can better understand what their customers feel and why they feel that way. This deeper insight allows for more informed actions based on actual customer sentiment, rather than just surface-level feedback.
Key Steps in Transforming Sentiment Data into Insights
- Data Collection: Gather feedback from multiple sources such as surveys, social media, customer support interactions, and reviews.
- Sentiment Categorization: Classify feedback into positive, neutral, and negative categories, and further break it down into sub-categories (e.g., satisfaction with product, service quality, etc.).
- Trend Identification: Identify recurring themes or sentiments across different feedback channels.
- Actionable Recommendations: Generate recommendations based on analyzed sentiment, highlighting areas for improvement or opportunity.
Transforming Insights into Action
- Prioritize Issues: Focus on issues that have the highest impact on customer satisfaction, and address those with the most urgency.
- Product or Service Enhancement: Use the feedback to improve features, adjust marketing strategies, or enhance customer support systems.
- Real-Time Adjustments: Implement changes in real-time based on ongoing sentiment analysis, ensuring that customer concerns are addressed promptly.
Example of Sentiment Analysis in Action
Feedback Category | Sentiment | Action |
---|---|---|
Product Quality | Negative | Investigate quality control processes, release a product update |
Customer Support | Positive | Continue using current support training, possibly expand staff |
Price | Neutral | Reevaluate pricing strategy, offer discounts for loyal customers |
Important: Sentiment analysis is only valuable if organizations act upon the insights gained. Simply collecting feedback without a clear action plan can lead to missed opportunities.