Sentiment Analysis Ediscovery
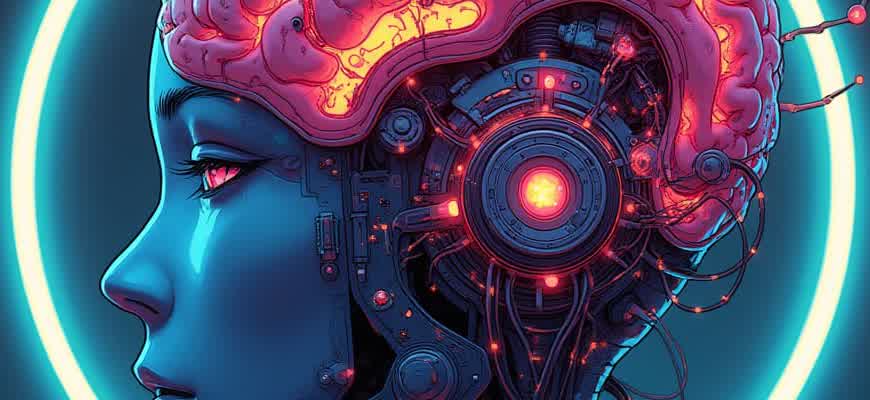
Sentiment analysis has become an essential tool in the field of electronic discovery (ediscovery), particularly when dealing with large volumes of unstructured data. This process helps identify the emotional tone behind written communications, which can be critical for assessing the intent or relevance of certain documents in legal investigations. By analyzing the sentiment of emails, social media posts, and other forms of communication, professionals can quickly flag key pieces of evidence that may not be immediately obvious through traditional search methods.
Applications of Sentiment Analysis in Ediscovery:
- Identification of crucial documents in legal cases
- Enhancement of compliance audits by filtering out irrelevant content
- Streamlining the review process by prioritizing high-impact messages
- Providing insights into parties' attitudes and emotional responses during litigation
Key Benefits:
Sentiment analysis in ediscovery can significantly reduce the time spent on document review, highlighting documents that exhibit emotional extremes or critical content related to the case.
Sentiment Analysis vs. Traditional Keyword Search:
Aspect | Sentiment Analysis | Keyword Search |
---|---|---|
Accuracy | Captures nuances like sarcasm or tone | Relies on exact match terms |
Efficiency | Reduces irrelevant data volume | Can generate large amounts of false positives |
Contextual Relevance | Considers emotional intent | Focuses on specific word matches |
How Sentiment Analysis Optimizes Ediscovery Workflows
Sentiment analysis plays a crucial role in enhancing eDiscovery processes by automatically evaluating the emotional tone of communication within large data sets. In legal cases, where understanding the context of documents and communications is paramount, sentiment analysis helps identify key emotional nuances that can impact the outcome of a case. By detecting the mood behind emails, social media posts, or corporate messages, it enables legal teams to prioritize and assess documents that might otherwise be overlooked.
This technology streamlines document review, ensuring that the most relevant and emotionally charged communications are highlighted early in the discovery process. This allows legal teams to focus on critical information while reducing time spent on irrelevant data, ultimately saving both time and costs.
Key Benefits of Sentiment Analysis in Ediscovery
- Prioritization of Documents: By identifying the emotional tone, sentiment analysis enables legal professionals to quickly focus on documents that may carry higher legal relevance or risk.
- Efficient Data Filtering: It helps sift through vast quantities of data, marking emotionally significant content that requires closer scrutiny.
- Improved Context Understanding: Legal teams gain a better understanding of the context behind the communications, improving the accuracy of their assessments and decisions.
How Sentiment Analysis Works in Ediscovery
- Data Collection: The first step involves gathering the relevant data–such as emails, text messages, and social media posts–within the scope of the case.
- Text Processing: Sentiment analysis algorithms break down the language used in the documents, identifying key terms and phrases that may indicate emotion.
- Emotion Categorization: The system categorizes emotions like anger, joy, fear, or sadness, providing a quantitative score of sentiment strength in each document.
- Results Integration: The analysis results are integrated into the review workflow, guiding the review team to focus on high-priority documents.
"Sentiment analysis empowers legal teams to uncover hidden emotional dynamics in communication, providing a deeper understanding of the facts at hand."
Example of Sentiment Analysis in Action
Document Type | Emotional Tone | Legal Relevance |
---|---|---|
Anger | High | |
Text Message | Joy | Low |
Social Media Post | Fear | Moderate |
Key Advantages of Incorporating Sentiment Analysis in Legal Investigations
Sentiment analysis plays a critical role in modern legal investigations, offering deeper insights into the emotional context of documents and communications. By analyzing tone, intent, and emotional cues, legal teams can quickly assess the relevance and potential impact of evidence. The ability to gauge sentiments embedded in emails, text messages, and other digital content significantly enhances the process of discovering pertinent information.
Beyond simply identifying keywords or phrases, sentiment analysis enables the identification of subtle emotional shifts within the data. This can be particularly valuable in e-discovery, where large volumes of data are reviewed. By integrating sentiment analysis, legal professionals can refine their investigations and focus on the most promising or problematic content more efficiently.
Key Benefits
- Improved Efficiency: By automatically detecting emotional content, sentiment analysis narrows down the most relevant documents, saving significant time and effort in document review.
- Enhanced Contextual Understanding: Analyzing emotions adds layers of context, helping legal teams interpret communications more accurately, especially in complex cases involving interpersonal dynamics.
- Risk Identification: Negative sentiments in communications may indicate potential legal risks or areas of conflict, enabling quicker mitigation strategies and more informed decision-making.
- Better Case Strategy: Sentiment analysis can guide the development of case strategies by revealing underlying emotional patterns that may be central to legal arguments or settlement negotiations.
Applications in Legal Workflows
- Litigation Support: Analyzing witness testimonies, email correspondence, and depositions to identify emotional tone and intent.
- Compliance Monitoring: Assessing communications to identify potentially harmful or non-compliant behavior, aiding in regulatory adherence.
- Internal Investigations: Sentiment analysis helps detect internal conflicts or inappropriate behavior by assessing the emotional content of employee communications.
Example of Sentiment Analysis in Action
Document Type | Sentiment | Potential Impact |
---|---|---|
Email from Employee | Negative | Indicates dissatisfaction, possible misconduct |
Customer Complaint | Angry | Possible breach of contract or service failure |
Management Memo | Neutral | No immediate concerns, but subject to closer review |
Note: Sentiment analysis helps prioritize the review of documents that have high emotional significance, ensuring that legal teams focus on key pieces of evidence with greater potential impact.
Automating Document Review with Sentiment Analysis Algorithms
In the context of eDiscovery, the process of reviewing large volumes of documents can be highly resource-intensive. Integrating sentiment analysis algorithms into the document review workflow has emerged as a way to streamline this process, enabling faster and more accurate identification of critical information. These algorithms analyze the underlying tone, emotions, and intentions behind the text, helping legal professionals prioritize documents based on the sentiment conveyed.
By using sentiment analysis, organizations can not only reduce the amount of manual effort involved but also improve the precision of their reviews. This approach offers several advantages, including identifying key statements, detecting biased language, and flagging documents that may require closer inspection due to their emotional content. As a result, legal teams are empowered to focus their resources on documents that matter the most to the case at hand.
Key Benefits of Using Sentiment Analysis in Document Review
- Increased Efficiency: Automated sentiment detection accelerates the review process, reducing the time spent by legal teams on document analysis.
- Improved Accuracy: By identifying emotional undertones, sentiment analysis helps to accurately assess the relevance of documents, reducing human error.
- Bias Detection: It aids in the recognition of biased language or emotional manipulation, which can be crucial for case outcomes.
- Prioritization: Documents with extreme sentiment can be flagged, allowing for faster prioritization of critical information.
Process of Integrating Sentiment Analysis Algorithms
- Data Preparation: Initial collection and categorization of documents based on type and relevance to the case.
- Sentiment Classification: Sentiment analysis algorithms are applied to the documents to classify them into categories such as positive, negative, or neutral.
- Review and Flagging: Documents with strong sentiment (positive or negative) are flagged for closer inspection by legal professionals.
- Feedback Loop: Continuous refinement of the algorithm based on user feedback and new data to improve its accuracy over time.
"Sentiment analysis provides a powerful tool for enhancing document review workflows, enabling faster decision-making and improving overall case management."
Challenges and Considerations
Challenges | Considerations |
---|---|
Inaccurate Sentiment Detection | Ensure algorithms are trained with diverse data sets to improve accuracy across different types of language. |
Contextual Misunderstanding | Sentiment analysis tools may misinterpret sarcasm or irony, leading to potential misclassifications. |
Integration Complexity | Incorporating sentiment analysis into existing eDiscovery platforms may require significant system integration efforts. |
Identifying Emotional Bias in Corporate Communications During Ediscovery
During the process of eDiscovery, organizations often sift through vast amounts of digital data to locate relevant information for legal cases. However, one critical challenge is recognizing the emotional undertones present in corporate communications. Emotional bias can subtly influence decision-making, messaging, and even legal outcomes. As companies increasingly rely on email correspondence, internal chats, and other forms of communication, understanding how emotional cues manifest in such content becomes essential for accurate analysis and fair legal practices.
Recognizing emotional bias in these communications is crucial for assessing the true intent behind corporate decisions, especially when evaluating tone, urgency, and sentiment. Emotional bias could cloud the interpretation of legal documents or key communication exchanges, potentially skewing the understanding of a case. Therefore, identifying and mitigating this bias becomes a key objective for teams working on eDiscovery projects.
Key Indicators of Emotional Bias in Corporate Communications
- Excessive use of emotional language: Terms like "outraged", "frustrating", or "exciting" can indicate strong emotional involvement.
- Overuse of exclamation marks: This often signifies heightened emotions, particularly in urgent or reactive communications.
- Defensive or aggressive tone: Phrases such as "you don’t understand" or "we were right to act this way" can suggest bias in the conversation.
- Context of urgency: Emails or messages that contain a high degree of urgency may reveal an emotional response to a particular situation, affecting decision-making.
Approaches for Detecting Emotional Bias in Communications
- Sentiment Analysis Tools: Implementing AI-driven tools to assess the emotional content of emails or documents helps to identify underlying sentiments.
- Natural Language Processing (NLP): NLP techniques can be used to dissect communication patterns, revealing emotions that might not be immediately obvious.
- Human Review: Having legal professionals with emotional intelligence conduct a secondary review of flagged communications can provide further clarity on intent and tone.
"Emotional bias in corporate emails and messages can distort the factual context of communications, leading to misinterpretation of intent, especially in legal proceedings."
Example of Emotional Bias in Corporate Communication
Communication | Emotional Bias Indicators |
---|---|
"We absolutely cannot afford to lose this client. We need to act fast and show them how much we care!" | Urgency, overuse of "cannot afford," emotional appeal ("show them how much we care") |
"I can’t believe the team missed this detail again, we’ve been clear about the expectations!" | Defensiveness, frustration, blame-shifting |
Optimizing Legal Case Strategy Using Sentiment Insights from Ediscovery
In modern legal practice, the use of sentiment analysis within the framework of ediscovery has become an invaluable tool for shaping case strategy. By analyzing the tone, emotion, and underlying sentiment in electronic communications, legal teams can gain insights into the mindset of key individuals, identify potential biases, and anticipate opposing arguments. Sentiment insights can transform raw data into actionable intelligence, making it possible to spot inconsistencies or weaknesses in the case early on.
Integrating sentiment analysis with traditional discovery processes adds a layer of depth, moving beyond document review into predictive analysis. Attorneys can identify hidden emotions or intentions in emails, messages, and other communications that may influence case outcomes. The following strategies highlight how sentiment insights can optimize case management and decision-making in litigation.
Key Benefits of Sentiment Insights in Legal Strategy
- Early Case Evaluation: Sentiment analysis reveals emotional trends in documents, helping identify which arguments might resonate with judges or juries.
- Informed Deposition Planning: By understanding the emotional state and stance of opposing witnesses, legal teams can refine questioning tactics and approach during depositions.
- Discovery of Undisclosed Motives: Hidden sentiments within emails or messages can expose underlying motives that may not be immediately obvious through direct evidence.
Practical Applications of Sentiment Analysis in Ediscovery
- Communication Pattern Identification: Analyzing a series of emails to detect shifts in tone or emotion can reveal critical turning points in a case.
- Litigation Risk Assessment: Sentiment trends in corporate communications may indicate potential risks, allowing legal teams to develop more effective risk mitigation strategies.
- Strategy Alignment: Sentiment analysis ensures the legal strategy aligns with the emotional and psychological aspects of the case, enhancing overall preparation.
Example of Sentiment Analysis Impact
Situation | Sentiment Insight | Strategic Action |
---|---|---|
Opposing counsel's emails show increasing frustration. | High levels of negative sentiment and aggression. | Prepare for a more confrontational trial approach and anticipate aggressive cross-examination. |
Internal communication shows reluctance to disclose critical information. | Emotional tension and secrecy detected. | Use this insight to anticipate challenges in discovery and prepare stronger counterarguments. |
Sentiment insights allow attorneys to be proactive, not reactive, enhancing their ability to forecast the opposition’s moves and gain the upper hand in litigation.
How Sentiment Analysis Reduces Manual Effort in Document Classification
In the context of eDiscovery, manual document classification can be an extremely time-consuming task. Traditionally, legal professionals must manually review vast amounts of data to identify relevant information, which often leads to inefficiencies and human errors. However, sentiment analysis technology has significantly streamlined this process by automatically categorizing documents based on their emotional tone and context. This not only accelerates the workflow but also improves the accuracy of identifying relevant documents, reducing the need for extensive manual intervention.
By leveraging sentiment analysis, organizations can quickly process large datasets and automatically classify documents into specific categories based on their sentiment. This approach minimizes the need for human reviewers to painstakingly examine each document, leading to substantial time savings. The reduction in manual effort also helps in cutting down labor costs, allowing legal teams to focus on more complex tasks that require human expertise.
Key Benefits of Sentiment Analysis in Document Classification
- Time Efficiency: Automated classification speeds up the review process, saving significant time compared to manual methods.
- Accuracy Improvement: Sentiment analysis ensures more consistent and precise document categorization.
- Cost Reduction: Less reliance on manual labor results in lower operational costs for eDiscovery teams.
"Sentiment analysis tools can categorize documents not just by content but by the underlying emotional tone, reducing ambiguity and enhancing relevance in legal proceedings."
Comparison of Manual vs. Sentiment-Based Classification
Aspect | Manual Classification | Sentiment-Based Classification |
---|---|---|
Time Required | Hours or days per document | Minutes per document |
Accuracy | Prone to human error | Consistent and reliable |
Scalability | Limited by human capacity | Highly scalable for large datasets |
In conclusion, the integration of sentiment analysis into the document classification process greatly enhances efficiency, accuracy, and scalability. As a result, organizations can handle larger volumes of data more effectively, ultimately leading to faster and more cost-effective eDiscovery procedures.
Detecting and Addressing Negative Sentiment in Social Media for Legal Cases
In legal cases, especially those involving reputational damage or defamation, it is crucial to analyze social media content for any expressions of negative sentiment. Negative comments, posts, or discussions can impact the outcome of a case, as they may reveal crucial evidence or influence public perception. Identifying these sentiments effectively requires advanced text analysis methods to assess the emotional tone of the content and distinguish between harmless opinions and potentially harmful statements.
Legal teams often rely on sentiment analysis tools to sift through vast amounts of social media data. These tools help detect negativity, categorize posts, and evaluate their relevance to the case. It is important to use sophisticated algorithms that go beyond simple keyword searches to understand the context in which negative emotions are expressed, ensuring that the legal team is equipped with accurate and actionable insights.
Approaches to Detecting Negative Sentiment
- Using Natural Language Processing (NLP) to evaluate emotional tone.
- Sentiment scoring models to measure positivity, neutrality, and negativity.
- Contextual analysis to avoid misinterpretations of sarcasm or irony.
Steps for Addressing Negative Content
- Gathering relevant data using sentiment analysis tools.
- Classifying content based on severity and potential impact on the case.
- Filtering out irrelevant or trivial information to focus on key evidence.
- Reporting findings to the legal team, with recommendations for action.
Key Considerations for Legal Cases
Consideration | Explanation |
---|---|
Legal Implications | Understanding how negative content can be used as evidence or defense in court. |
Public Perception | Addressing the potential influence of social media sentiment on jury pools or public opinion. |
Data Privacy | Ensuring that social media data is collected and analyzed in compliance with privacy laws. |
Effective sentiment analysis not only helps in identifying negative content but also in determining its significance in the legal context. It's important to balance the precision of algorithms with the understanding of the case's broader implications.