Ai Driven Data Analytics
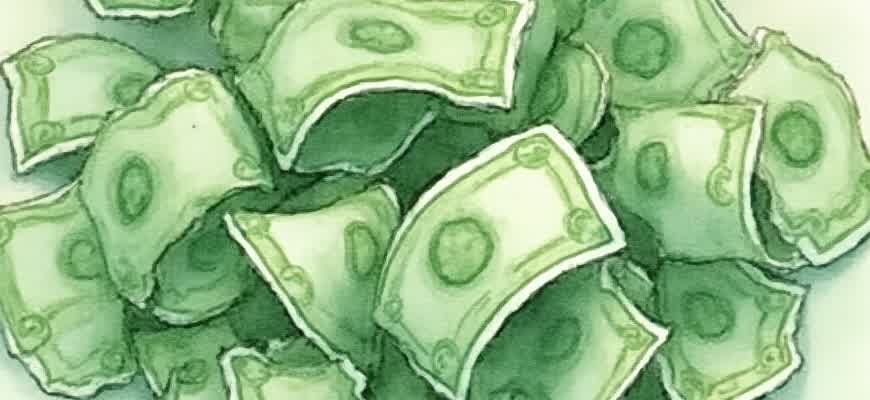
Artificial Intelligence has revolutionized the way businesses approach data analysis, enabling deeper insights and more accurate predictions. Traditional methods of analyzing data often fall short in dealing with the sheer volume and complexity of modern datasets. AI-driven systems, however, enhance this process by using advanced algorithms to process and interpret data at a much faster rate.
Key Features of AI-Powered Data Analytics:
- Speed and Efficiency: AI models can analyze vast datasets in real-time, far outpacing human capabilities.
- Pattern Recognition: Machine learning algorithms can identify hidden patterns in data that might otherwise go unnoticed.
- Predictive Analytics: AI systems can predict trends and outcomes based on historical data, improving decision-making accuracy.
"AI-driven data analytics is no longer a luxury for large enterprises; it's becoming a standard tool for gaining a competitive edge in virtually every industry."
Applications of AI in Data Analysis:
- Fraud detection and risk management in financial sectors
- Customer behavior analysis for personalized marketing campaigns
- Predictive maintenance in manufacturing industries
Technology | Benefit |
---|---|
Machine Learning | Improves decision-making through data-driven insights. |
Natural Language Processing | Enables the analysis of unstructured text data from sources like customer reviews. |
AI-Powered Data Insights
AI-driven analytics is revolutionizing the way businesses extract value from data. By leveraging machine learning algorithms and advanced computational models, AI systems are able to identify patterns and correlations in large datasets that would be nearly impossible for human analysts to uncover. This leads to more precise decision-making and faster, data-driven actions. The shift towards AI-based analytics provides companies with a competitive edge by enhancing both predictive accuracy and operational efficiency.
In contrast to traditional analytics methods, AI can automatically adapt to new data, improving over time as more data becomes available. This dynamic ability allows for real-time insights and a deeper understanding of market trends, customer behaviors, and operational bottlenecks. Companies that successfully integrate AI-driven data analytics into their workflow are positioned to better anticipate future needs, optimize resources, and maximize profitability.
Key Benefits of AI-Driven Analytics
- Real-time Decision Making: Instant access to insights for quick and informed actions.
- Predictive Capabilities: AI models forecast future trends based on historical data.
- Data-Driven Personalization: AI enhances customer experience through tailored recommendations.
- Cost Efficiency: Automates repetitive tasks, reducing human resource needs and errors.
AI Techniques in Data Analysis
- Machine Learning (ML): Helps uncover patterns through algorithms that learn from data.
- Natural Language Processing (NLP): Extracts meaning from textual data like customer feedback or social media posts.
- Neural Networks: Mimics the human brain to detect complex relationships in data.
- Deep Learning: A subset of ML that uses multi-layered neural networks for more accurate analysis.
Real-World Application: Retail Industry
AI Tool | Use Case | Benefit |
---|---|---|
Customer Segmentation | Identifying distinct customer groups based on behavior and preferences. | Enables personalized marketing and better inventory management. |
Demand Forecasting | Predicting product demand using historical sales data. | Helps optimize stock levels, reducing waste and ensuring product availability. |
"AI-driven analytics empowers organizations to make data-centric decisions faster and with greater accuracy, offering a level of insight that was once unattainable."
How AI-Powered Analytics Enhances Business Decision Making
Artificial intelligence-driven data analytics transforms how businesses approach decision-making by offering actionable insights derived from vast amounts of data. By leveraging machine learning algorithms and advanced statistical models, AI can quickly identify trends, patterns, and correlations that might otherwise go unnoticed. This capability allows companies to make faster, more accurate decisions based on evidence rather than intuition.
AI technologies are not limited to simple data analysis; they also enable predictive analytics, where businesses can foresee future trends and outcomes. By incorporating these advanced analytics into their operations, companies can significantly enhance their strategic planning, marketing efforts, and customer service initiatives. Below are key ways AI-powered data analytics is transforming decision-making processes.
Key Benefits of AI-Driven Decision Making
- Increased Accuracy: AI systems process large datasets efficiently, reducing human error and providing more reliable outcomes.
- Faster Insights: Real-time data processing allows for immediate responses to emerging trends and challenges, enhancing agility.
- Predictive Capabilities: Machine learning models forecast future events, helping businesses proactively plan and mitigate risks.
How AI Improves Specific Business Functions
- Marketing: AI-driven tools analyze customer behavior, preferences, and interactions to deliver personalized marketing campaigns that boost conversion rates.
- Finance: AI models assess financial trends and market conditions to inform investment strategies, minimize risks, and optimize portfolio performance.
- Operations: Machine learning algorithms identify inefficiencies in supply chains and logistics, enabling cost reduction and process optimization.
"AI-powered analytics not only helps businesses react to market shifts but also positions them to lead by anticipating changes before they happen."
Example: AI-Driven Insights in Action
Business Area | AI Application | Outcome |
---|---|---|
Retail | Customer behavior analysis | Increased sales through personalized product recommendations |
Finance | Risk prediction models | Improved investment decisions and reduced financial exposure |
Manufacturing | Predictive maintenance | Lowered downtime and extended equipment life cycle |
Key AI Techniques Transforming Data Analysis: Machine Learning & NLP
Artificial Intelligence (AI) has significantly impacted the way organizations analyze and interpret data. Among the key techniques driving this transformation are Machine Learning (ML) and Natural Language Processing (NLP). These methodologies allow for more accurate, efficient, and automated data insights, helping businesses stay competitive in an increasingly data-driven world. Both ML and NLP harness vast datasets to uncover patterns, make predictions, and enhance decision-making processes in real-time.
Machine Learning, with its ability to process and learn from large volumes of data, is particularly valuable for predictive analytics and anomaly detection. On the other hand, Natural Language Processing allows AI systems to understand, interpret, and generate human language, offering powerful tools for text analysis, sentiment analysis, and automated reporting. The combination of these technologies is revolutionizing how companies extract actionable insights from complex datasets.
Machine Learning (ML) in Data Analytics
Machine Learning algorithms are essential for extracting insights from structured and unstructured data by identifying patterns, making predictions, and automating repetitive tasks. Some of the most common ML techniques applied in data analysis include:
- Supervised Learning: Models are trained on labeled datasets to predict outcomes based on input features.
- Unsupervised Learning: Algorithms identify hidden patterns or groupings in data without labeled outcomes.
- Reinforcement Learning: Systems learn through trial and error, maximizing rewards in decision-making processes.
Machine learning techniques are often used in fraud detection, predictive maintenance, and customer segmentation, among other applications.
Natural Language Processing (NLP) in Data Analysis
Natural Language Processing enables AI systems to process and analyze textual data, which is often unstructured. It transforms raw language data into meaningful insights. Key applications of NLP include:
- Sentiment Analysis: Identifying emotional tone from text, valuable for brand monitoring and customer feedback analysis.
- Text Classification: Organizing text into predefined categories, such as spam detection or topic categorization.
- Named Entity Recognition (NER): Extracting entities such as dates, places, and organizations from text.
By combining ML and NLP, organizations can derive insights not just from numeric data, but also from vast amounts of textual data, such as customer reviews, social media interactions, and support tickets. This holistic approach enhances decision-making and enriches the overall data analysis process.
Technique | Application | Benefit |
---|---|---|
Machine Learning | Predictive Analytics, Anomaly Detection | Improves accuracy and automates data-driven decision-making |
Natural Language Processing | Text Mining, Sentiment Analysis | Extracts meaningful insights from unstructured data |
Streamlining Data Integration with AI: Reducing Complexity
Data integration involves combining data from various sources into a unified view, a process that often involves complex steps like cleaning, transformation, and validation. AI can significantly simplify this process by automating repetitive tasks and enabling real-time processing of large datasets. As data becomes increasingly fragmented across different platforms, AI can reduce the overhead typically associated with manual integration methods, leading to faster and more accurate results.
Machine learning algorithms, especially those focused on natural language processing and pattern recognition, can identify relationships between disparate data sources. By automating the identification and mapping of relevant data, AI reduces the need for manual intervention and minimizes human error. This approach streamlines workflows, lowers operational costs, and increases efficiency across organizations.
Key Benefits of AI in Data Integration
- Speed and Scalability: AI-powered systems can process and integrate vast datasets at unprecedented speeds, reducing the time needed for data aggregation.
- Data Accuracy: AI algorithms can identify anomalies and inconsistencies in data, ensuring that only accurate information is integrated into the system.
- Real-time Processing: AI allows for continuous, real-time integration of new data, enhancing decision-making processes.
AI Tools for Simplifying Data Integration
- Data Wrangling Tools: These tools use AI to automate the extraction, transformation, and loading (ETL) of data from different sources into a standardized format.
- Data Mapping Algorithms: AI can automatically map data fields across different sources, reducing manual mapping efforts and ensuring consistent data structure.
- Data Validation Systems: AI systems can cross-check and validate data integrity across multiple platforms, identifying and correcting errors in real-time.
AI-driven integration solutions are not just a way to handle data more effectively; they redefine the approach to managing data complexity. By minimizing human oversight and automating key functions, these tools create smarter workflows that are easier to maintain and scale.
Comparing Traditional vs. AI-Driven Data Integration
Traditional Integration | AI-Driven Integration |
---|---|
Manual data cleaning and mapping | Automated anomaly detection and mapping |
Slow processing times | Real-time, high-speed integration |
High risk of human error | Minimized human involvement with high accuracy |
AI-Driven Predictive Analytics: Anticipating Market Shifts and Consumer Actions
AI-powered predictive analytics is transforming how businesses forecast market behavior and anticipate consumer trends. By analyzing vast amounts of historical data and recognizing patterns, AI can provide actionable insights into future developments. These technologies leverage machine learning models to predict potential outcomes and behaviors, offering organizations the ability to stay ahead of the curve and make informed decisions based on data-driven projections.
Predictive models utilize advanced algorithms to identify hidden correlations and trends that may not be immediately apparent. These insights are then applied to predict future events, enabling businesses to adapt their strategies proactively. In various industries, such as retail, finance, and healthcare, AI-based predictions help optimize resources, mitigate risks, and enhance customer experience by predicting demand, financial shifts, or health outcomes.
Key Applications of AI-Driven Forecasting
- Market Trend Analysis: AI can analyze historical data to identify shifts in consumer preferences, helping businesses stay ahead of changing demands.
- Customer Behavior Prediction: By examining past interactions, AI predicts future customer actions, enabling personalized marketing strategies.
- Financial Forecasting: Predictive analytics can assess economic indicators and historical financial data to anticipate market movements and investment opportunities.
Process of AI-Based Predictive Modeling
- Data Collection: Gather historical and real-time data relevant to the industry or specific business processes.
- Model Training: Use machine learning algorithms to train models based on the collected data.
- Pattern Recognition: AI detects hidden patterns and correlations within the data that could indicate future trends.
- Prediction Generation: The model generates forecasts based on detected patterns, with varying degrees of confidence.
- Continuous Learning: As new data is acquired, the model adapts and improves, refining its predictions over time.
Example of AI-Based Predictive Analytics in Action
Industry | Use Case | AI Application |
---|---|---|
Retail | Demand Forecasting | AI predicts which products will be in demand based on seasonal trends and consumer behavior. |
Finance | Risk Assessment | AI analyzes financial transactions to detect potential risks or fraudulent activity. |
Healthcare | Patient Outcome Prediction | AI predicts patient recovery rates or risks based on historical health data and demographics. |
AI-driven predictive analytics is not just about predicting the future, but about using those predictions to make smarter, data-backed decisions that improve business outcomes.
Optimizing Customer Segmentation through AI-Driven Insights
AI-powered analytics have revolutionized the way businesses understand and engage with their customers. By leveraging vast amounts of data, AI tools can uncover intricate patterns that traditional segmentation methods may overlook. These insights enable businesses to create more precise and effective customer segments, ultimately driving personalized marketing strategies and improving customer retention.
One of the most powerful aspects of AI in customer segmentation is its ability to process and analyze diverse data sources, such as behavioral data, transaction history, and social media activity. With advanced machine learning algorithms, businesses can dynamically adjust their segments in real-time, ensuring that marketing efforts remain relevant and impactful.
Key Strategies for AI-Optimized Segmentation
- Behavioral Analytics: AI can track and predict consumer behavior, identifying specific actions and preferences that define a segment.
- Cluster Analysis: Machine learning algorithms group customers based on shared characteristics, allowing businesses to identify niche segments with high potential.
- Predictive Modeling: AI forecasts future customer behavior based on historical data, helping businesses anticipate needs and tailor their offerings accordingly.
Benefits of AI-Driven Segmentation
AI enables businesses to uncover hidden opportunities by examining data more deeply than traditional methods. By identifying more granular customer segments, companies can refine their targeting strategies, increase ROI, and enhance customer satisfaction.
- Improved Accuracy: AI algorithms reduce human bias, ensuring that customer segments are defined with precision.
- Dynamic Segmentation: AI adjusts segments in real-time, responding to shifts in consumer behavior and market trends.
- Cost Efficiency: By targeting the most relevant segments, businesses reduce marketing spend while improving conversion rates.
Example of AI-Optimized Segmentation
Customer Segment | Behavioral Traits | Marketing Strategy |
---|---|---|
Tech-Savvy Millennials | Frequent online shoppers, early adopters of new technology | Offer exclusive online deals, promote new gadgets |
Price-Sensitive Shoppers | Look for discounts, sensitive to price fluctuations | Provide discount codes, run limited-time promotions |
Loyal Customers | Repeat buyers, high lifetime value | Offer loyalty programs, personalized recommendations |
Real-Time Data Processing with AI: Benefits and Use Cases
AI-powered real-time data analysis offers organizations the ability to make swift decisions based on live data streams. By processing incoming information immediately, businesses can adjust operations, detect anomalies, and optimize processes without delay. This capability is particularly useful in industries where time-sensitive decisions are crucial, such as finance, healthcare, and e-commerce.
Integrating AI into real-time data processing systems enhances the ability to handle vast volumes of data with minimal human intervention. It provides a significant advantage by improving operational efficiency and enabling predictive insights. The system learns from historical data and current inputs, making adjustments and predictions with remarkable speed.
Key Benefits of AI for Real-Time Data Processing
- Faster Decision Making: AI analyzes data as it arrives, providing immediate insights and allowing businesses to respond quickly.
- Improved Accuracy: AI models identify patterns and trends in real-time, offering precise predictions that reduce human error.
- Cost Reduction: Automation of data processing reduces the need for manual oversight, leading to significant cost savings.
- Scalability: AI systems can scale to handle increasing amounts of data without compromising performance.
Real-World Applications of AI in Real-Time Data Processing
- Fraud Detection in Finance: AI systems monitor transactions in real-time, identifying unusual patterns and preventing fraudulent activities.
- Predictive Maintenance in Manufacturing: AI processes sensor data from machines to predict when maintenance is needed, preventing costly breakdowns.
- Personalized Marketing in E-Commerce: Real-time customer behavior analysis allows companies to offer personalized recommendations and targeted promotions.
"AI in real-time analytics doesn't just improve decision-making speed; it also creates smarter, more adaptive systems that evolve with the data they process."
Challenges in Implementing Real-Time AI Analytics
Challenge | Description |
---|---|
Data Volume | The sheer amount of data generated in real-time can overwhelm traditional systems without AI's ability to process it efficiently. |
Integration Complexity | Integrating AI into existing systems and workflows can be complex, requiring significant investment and time. |
Data Privacy | Real-time data collection can raise privacy concerns, requiring strict adherence to regulations like GDPR. |
Securing Data Privacy and Compliance in AI Analytics Platforms
In the era of artificial intelligence, data privacy and regulatory compliance are central to the trustworthiness and functionality of AI-powered analytics platforms. With AI's increasing role in data-driven decision-making, organizations must ensure that sensitive information is securely handled, while also meeting global standards such as GDPR, HIPAA, and other regional regulations. The integration of AI technologies into analytics workflows demands robust mechanisms to protect privacy and guarantee compliance throughout data processing stages.
To safeguard data privacy and meet legal requirements, AI platforms must implement a comprehensive strategy that covers data encryption, access control, and audit trails. Furthermore, organizations should prioritize transparency and provide mechanisms for individuals to exercise their rights over personal data. Ensuring compliance is not just a technical challenge, but also a governance and ethical responsibility.
Key Approaches to Data Security and Compliance
- Data Encryption: Ensuring all sensitive data is encrypted both in transit and at rest.
- Access Control: Implementing role-based access and strict authentication measures to limit exposure.
- Data Minimization: Collecting only the essential data necessary for analytics purposes.
- Audit Trails: Creating detailed logs of all data access and processing actions for accountability.
- Compliance Monitoring: Continuously monitoring regulatory updates to adjust practices and stay compliant.
Compliance Requirements for AI Analytics Platforms
- General Data Protection Regulation (GDPR): AI platforms must implement processes to protect personal data and ensure individuals' rights to access, rectify, and delete their data.
- Health Insurance Portability and Accountability Act (HIPAA): For healthcare-related analytics, platforms must comply with HIPAA regulations, ensuring that health information is processed securely.
- California Consumer Privacy Act (CCPA): Ensuring that personal data of California residents is handled according to specific privacy requirements.
Important: Non-compliance with data protection regulations can result in severe penalties, ranging from financial fines to reputational damage.
AI Data Compliance in Practice
Regulation | Focus | Compliance Measures |
---|---|---|
GDPR | Protection of personal data | Data subject rights, encryption, data minimization |
HIPAA | Protection of health information | Secure access controls, audit trails, encryption |
CCPA | Consumer privacy rights | Opt-out mechanisms, data access requests |