Ai Based Lead Scoring
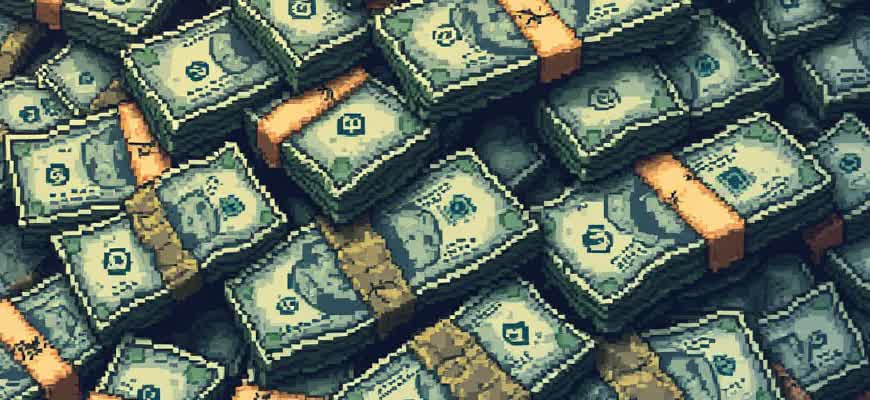
AI-based lead scoring leverages machine learning algorithms to evaluate and rank leads based on their likelihood to convert into customers. Unlike traditional methods, this approach uses data-driven insights to predict the potential of each lead, optimizing sales efforts and boosting conversion rates.
Key Benefits of AI in Lead Scoring:
- Improved accuracy in predicting conversions
- Automated and real-time lead evaluation
- Better prioritization of high-value leads
- Continuous learning and adaptation
How AI Enhances Lead Scoring:
- Data Collection: AI tools analyze historical data, such as past interactions and demographics, to build a lead profile.
- Pattern Recognition: By recognizing patterns in past successful conversions, AI systems score leads based on factors that lead to sales.
- Lead Prioritization: The AI system ranks leads based on their conversion potential, allowing sales teams to focus on the most promising prospects.
"AI-based lead scoring significantly reduces the time spent on unqualified leads, allowing sales teams to focus on prospects with the highest chance of conversion."
Lead Attribute | Impact on Scoring |
---|---|
Engagement Level | High interaction levels signal greater interest, increasing the lead score. |
Demographic Data | Specific characteristics like industry or job title influence the likelihood of conversion. |
Past Purchases | Previous transactions can indicate a higher probability of future purchases, impacting the score positively. |
AI-Powered Lead Scoring
AI-based lead scoring involves the use of machine learning models and data-driven techniques to evaluate the potential of leads in real time. By analyzing historical interactions, behaviors, and demographic data, AI tools can predict which leads are more likely to convert, streamlining the sales process. This approach ensures that sales teams focus on high-value prospects, increasing efficiency and overall conversion rates.
Unlike traditional lead scoring methods that rely on manual scoring or simple algorithms, AI systems dynamically adjust to changing patterns in lead behavior and market trends. These systems are continuously learning from new data, enhancing their predictive capabilities over time. AI models can process vast amounts of information, offering a more comprehensive and accurate assessment of a lead’s quality.
Key Components of AI-Based Lead Scoring
- Behavioral Analysis: AI algorithms track user behavior across various touchpoints, identifying patterns that signal higher intent.
- Predictive Modeling: Machine learning techniques predict future outcomes based on historical data, improving lead prioritization.
- Data Integration: AI models integrate data from multiple sources, ensuring a 360-degree view of each lead.
- Real-Time Adjustments: AI systems continuously adapt to new data, adjusting lead scores to reflect current trends and interactions.
How AI Scoring Works
- Data Collection: AI collects data from lead interactions such as website visits, social media engagement, email responses, and more.
- Data Processing: The system processes this data to identify key features that correlate with successful conversions.
- Lead Scoring: The AI assigns a score to each lead, with higher scores indicating greater likelihood of conversion.
- Continuous Learning: AI models refine their scoring algorithms based on new interactions and outcomes, improving over time.
"AI-based lead scoring not only saves time but also empowers sales teams to focus on the most promising prospects, increasing revenue potential."
Sample Lead Score Table
Lead Name | Lead Score | Status |
---|---|---|
John Doe | 85 | High Priority |
Jane Smith | 65 | Medium Priority |
Tom Brown | 45 | Low Priority |
How AI Enhances Accuracy in Lead Scoring Models
AI-driven lead scoring models have revolutionized the way businesses assess potential customers by using advanced data analysis techniques. Traditional methods of scoring leads often relied on static criteria and manual input, but with AI, the process becomes dynamic and highly adaptable. By analyzing large datasets, machine learning algorithms can identify patterns that predict which leads are most likely to convert into paying customers. This ability to continuously learn from new data makes AI-based models far more accurate than their manual counterparts.
AI improves lead scoring accuracy by automating the scoring process and integrating various data points that humans might overlook. These models can incorporate behavioral data, engagement patterns, and demographic details in real-time, ensuring that lead scores reflect up-to-date information. As a result, businesses can focus on leads that have the highest likelihood of conversion, optimizing their sales and marketing efforts.
Key Benefits of AI in Lead Scoring
- Real-Time Adaptability: AI models can quickly adjust to new trends or shifts in customer behavior, providing up-to-the-minute lead scores.
- Data-Driven Insights: By leveraging massive datasets, AI algorithms uncover hidden correlations between lead characteristics and conversion rates.
- Personalized Lead Scoring: AI enables tailored scoring models based on a company’s specific business needs and customer profiles.
How AI Improves Scoring Accuracy
- Advanced Pattern Recognition: AI systems analyze historical data to identify specific patterns and behaviors that are strong indicators of conversion probability.
- Predictive Analytics: Using historical lead data, AI predicts future behavior, allowing businesses to focus on high-potential leads.
- Continuous Learning: Machine learning algorithms continuously improve by processing new data, which allows the system to refine its scoring over time.
Example Comparison: Traditional vs. AI Lead Scoring
Criteria | Traditional Scoring | AI-Based Scoring |
---|---|---|
Data Sources | Static demographic data | Real-time behavioral, demographic, and engagement data |
Adaptability | Manual adjustments | Automatic adaptation to new trends |
Prediction Accuracy | Limited to predefined criteria | Highly accurate with predictive modeling |
"AI-based lead scoring models can continuously refine their accuracy by incorporating vast amounts of real-time data, ensuring businesses focus on leads with the highest conversion potential."
Understanding Key Data Inputs for AI Lead Scoring Systems
AI-driven lead scoring systems rely on various data points to assess and prioritize prospects, ensuring that sales teams focus their efforts on leads with the highest potential. Understanding the key inputs into these systems is essential for optimizing the process. These data inputs typically consist of both historical and behavioral data, which are then analyzed using machine learning algorithms to predict the likelihood of conversion.
Effective lead scoring systems integrate multiple sources of data to produce an accurate lead score. These inputs include demographic information, engagement levels, and past interactions with the brand. AI models use this data to identify patterns that indicate which leads are most likely to convert into customers.
Key Data Inputs in Lead Scoring
- Demographic Data: Includes lead's job title, company size, industry, location, etc. This helps assess the fit between the lead and your product or service.
- Behavioral Data: Tracks actions such as email opens, website visits, content downloads, and form submissions. Behavioral data reflects a lead's engagement with your brand.
- Engagement History: Data points such as past interactions with sales representatives, previous purchases, or inquiries provide insight into the lead's intent and readiness to convert.
- Social Media Activity: Social signals like LinkedIn profile updates, Twitter mentions, or engagement with company posts can help assess lead interest and activity.
Factors to Consider
- Lead Source: Identifying the origin of the lead (e.g., organic search, paid ads, referrals) helps determine their quality and likelihood of conversion.
- Engagement Frequency: Leads who engage frequently with your content or communications are more likely to be interested in purchasing.
- Lead Demographic Fit: A lead that matches your ideal customer profile (ICP) is more likely to convert than one that does not.
Accurate lead scoring depends heavily on the quality and richness of the data inputted into the AI model. Poor data quality can lead to inaccurate predictions and wasted sales efforts.
Data Types in Detail
Data Type | Description | Examples |
---|---|---|
Demographic | Details about the lead's personal or professional profile. | Job title, company size, industry, location |
Behavioral | Actions taken by the lead that show interest or intent. | Website visits, email opens, content downloads |
Engagement History | Past interactions with the brand, indicating level of interest. | Past purchases, form submissions, customer service requests |
Enhancing Lead Conversion through AI-Driven Algorithms
Artificial intelligence (AI) offers powerful tools to optimize lead conversion by predicting and assessing the likelihood of a prospect becoming a paying customer. By analyzing historical data and customer behaviors, AI algorithms can generate insights that are otherwise difficult to identify using traditional methods. This allows businesses to focus their efforts on leads with the highest potential, improving both efficiency and conversion rates.
AI models, especially those based on machine learning, continuously learn and improve as they process more data. These models consider various factors such as engagement patterns, previous interactions, demographic information, and purchasing behavior to score leads. As a result, businesses can effectively prioritize leads that are more likely to convert, reducing time spent on unqualified prospects.
Key Benefits of AI in Lead Conversion
- Improved Lead Scoring: AI can dynamically adjust lead scores based on real-time data, identifying the most promising leads for immediate follow-up.
- Better Customer Insights: AI-driven models provide detailed insights into customer behavior, preferences, and needs, enabling more personalized communication.
- Higher Efficiency: Automated lead nurturing ensures that no prospect is left unattended, allowing sales teams to focus on leads that have a high probability of conversion.
Steps to Leverage AI for Effective Lead Conversion
- Data Collection: Gather comprehensive data about leads, including demographic information, interaction history, and purchasing behaviors.
- Model Training: Use machine learning algorithms to analyze the data and train the AI model to predict conversion likelihood.
- Lead Scoring: Apply AI-based models to assign lead scores, identifying high-potential leads.
- Personalized Outreach: Utilize AI insights to tailor communication strategies for each lead, increasing engagement and conversion chances.
AI algorithms help sales teams focus on what matters: the leads that are most likely to convert. By understanding customer behavior and predicting conversion probability, AI significantly improves the efficiency of sales efforts.
Comparing Traditional vs AI-Driven Lead Scoring
Aspect | Traditional Scoring | AI-Driven Scoring |
---|---|---|
Lead Scoring Method | Manual, based on predefined criteria | Dynamic, continuously updated based on data |
Data Utilization | Limited to basic demographic data | Incorporates behavioral, demographic, and historical interaction data |
Accuracy | May overlook key insights, prone to biases | Highly accurate, adjusts to new data trends |
Efficiency | Time-consuming, requires constant manual updates | Automated, real-time adjustments |
AI Tools to Integrate with Your Existing Lead Management System
Integrating AI tools into your current lead management infrastructure can significantly enhance your ability to identify and prioritize high-value prospects. These tools leverage machine learning and predictive analytics to provide accurate scoring, allowing sales teams to focus on leads with the highest conversion potential. Additionally, AI-driven lead scoring can evolve over time, continuously improving as more data becomes available and patterns are recognized. This seamless integration ensures that your existing system benefits from enhanced automation and more insightful data-driven decisions.
To maximize the value of AI-based lead scoring, it’s essential to choose the right AI solutions that work smoothly with your CRM and lead management system. Many tools are designed to complement your workflow without requiring a complete system overhaul. Whether you're working with Salesforce, HubSpot, or other platforms, AI integration can be customized to suit your needs and drive better results across the sales funnel.
Key AI Tools for Integration
- Predictive Analytics Tools: These tools use historical data to predict future behaviors and identify which leads are most likely to convert.
- Natural Language Processing (NLP): NLP tools can analyze communication from leads (emails, chat messages) and provide insights into their intent and urgency.
- Lead Scoring Algorithms: AI-powered algorithms can calculate a dynamic score for each lead, adjusting in real-time based on interactions and activities.
- Customer Data Platforms (CDPs): AI-driven CDPs aggregate data from various sources to provide a unified, actionable lead profile for your sales team.
Benefits of AI Integration with Lead Management Systems
- Improved Lead Prioritization: AI enables more accurate lead ranking by analyzing complex datasets and identifying trends that would be difficult for human teams to spot.
- Time and Resource Optimization: With AI handling lead scoring, your sales team can focus on high-priority leads, minimizing time spent on less promising prospects.
- Increased Conversion Rates: AI tools can identify hidden opportunities, ultimately improving conversion rates and ROI by ensuring efforts are concentrated on the best leads.
Popular AI Tools to Integrate
Tool | Features | Best for |
---|---|---|
HubSpot AI | Lead scoring, predictive analytics, email tracking | Small to mid-sized businesses |
Salesforce Einstein | Advanced lead scoring, predictive insights, workflow automation | Enterprises with large sales teams |
Infer | Lead scoring, data enrichment, predictive analytics | Businesses seeking data-driven lead scoring |
Tip: Choose AI tools that can be easily integrated with your CRM to avoid disruptions in your current workflow. Smooth integration ensures that your lead management system remains effective while benefiting from AI-powered enhancements.
How Machine Learning Enhances Lead Scoring Over Time
Machine learning brings a powerful advantage to lead scoring by continuously adapting and improving its predictions. Unlike traditional methods that rely on static criteria, ML algorithms learn from historical data and refine their models over time, which leads to more accurate assessments of leads. This iterative learning process helps identify patterns and behaviors that may not be immediately obvious to human analysts. As the model receives more data, its accuracy increases, providing better insights into which leads are most likely to convert.
One of the key benefits of using machine learning for lead scoring is its ability to process large amounts of data quickly and efficiently. By analyzing customer behavior, demographic information, and engagement history, ML models can prioritize leads based on a variety of factors. Over time, as new data is incorporated, the system becomes more adept at distinguishing between high-value and low-value prospects, thus optimizing the sales process.
Continuous Improvement through Data Learning
- Pattern Recognition: ML identifies subtle patterns that may not be apparent through manual analysis.
- Dynamic Adjustments: The model adjusts lead scores as new data comes in, improving over time.
- Predictive Accuracy: Algorithms become more precise in forecasting the likelihood of conversion based on historical outcomes.
How Data Changes Lead Scoring
As the model ingests more data, it refines the way it calculates lead value. This is typically achieved through an iterative process where the system tests different algorithms and parameters to determine the most effective approach for scoring leads.
“Machine learning continuously adapts to emerging trends, allowing businesses to stay ahead of changes in customer behavior.”
Example: Improved Lead Scoring Process
Stage | Data Insights | Lead Scoring Adjustment |
---|---|---|
Initial Data | Basic customer demographics and behavior. | Leads are scored based on simple rules and predefined characteristics. |
Ongoing Data Integration | Engagement patterns and social signals. | Scores adapt to reflect engagement intensity and historical conversion rates. |
Advanced Data Analysis | Advanced insights like predictive analytics, intent signals, and product usage data. | Lead scores are fine-tuned with higher accuracy, identifying leads likely to convert in the near future. |
Optimizing Lead Scoring Models for Different Market Segments
Effective lead scoring is essential for businesses to prioritize potential customers and allocate resources efficiently. When applied across different market segments, scoring models must be adjusted to reflect the unique characteristics and behaviors of each segment. Customizing these models ensures that organizations can predict lead potential with greater accuracy, increasing conversion rates and overall sales effectiveness.
To optimize lead scoring for diverse segments, businesses must first identify key factors specific to each group, such as purchasing behavior, industry trends, and demographic information. Once these factors are determined, the lead scoring system can be fine-tuned to evaluate leads based on their relevance to each segment. This tailored approach helps businesses avoid a one-size-fits-all model, which may not effectively capture the nuances of distinct market groups.
Key Strategies for Segment-Specific Lead Scoring
- Data Segmentation: Group leads based on shared characteristics (e.g., industry, company size, geographic location) to tailor scoring models accordingly.
- Behavior Analysis: Understand specific actions or signals that indicate lead interest within each segment, such as content engagement or product trial usage.
- Lead Qualification Adjustments: Adapt qualification criteria to reflect varying expectations across segments. For example, high-value leads in one market may require more in-depth qualification than in another.
Examples of Segment-Specific Adjustments
Market Segment | Key Lead Scoring Factor | Adjustment to Model |
---|---|---|
Small Businesses | Price Sensitivity | Increase weight on budget-related signals and cost-conscious behavior. |
Enterprise Clients | Decision-Making Process | Increase weight on multi-step engagement signals, such as involvement from different departments. |
Tech Startups | Innovation Readiness | Adjust scoring based on the lead’s openness to new technologies and innovative solutions. |
Important Consideration: It's essential to continually refine scoring models as market dynamics evolve. Ongoing analysis of lead conversion patterns within each segment ensures that the scoring model remains relevant and effective.
Common Pitfalls in AI Lead Scoring and How to Avoid Them
AI-driven lead scoring models are highly effective for enhancing sales strategies, but their implementation is often fraught with potential pitfalls that can compromise the accuracy and reliability of predictions. Understanding these challenges and taking preventive measures can help businesses fully leverage AI in lead qualification processes.
One of the most significant issues is the reliance on biased data, which can lead to skewed results and inaccurate scoring. The quality of data is essential, and any inconsistencies or historical biases can negatively affect the performance of AI models. Additionally, improper feature selection and lack of continuous monitoring are common factors that contribute to suboptimal outcomes in AI lead scoring systems.
Key Challenges and Solutions
- Data Quality Issues: Using incomplete or outdated data can lead to incorrect lead scoring. Always ensure that the data used for training AI models is clean, relevant, and up to date.
- Model Overfitting: If the model is too complex, it might perform well on training data but fail to generalize to real-world scenarios. To prevent this, use simpler models and apply cross-validation techniques to test performance across different data sets.
- Ignoring Domain Expertise: AI models should be seen as complementary to human judgment, not a replacement. Ensure that domain experts are involved in defining the parameters and interpreting the results.
- Failure to Monitor Performance: AI models require regular updates to remain accurate over time. Continuous monitoring of model performance and making adjustments based on changes in lead behavior and market conditions are crucial.
How to Overcome These Challenges
- Data Preprocessing: Invest in robust data cleaning and normalization practices to ensure high-quality inputs into the AI system.
- Regular Model Evaluation: Continuously assess model performance against real outcomes, adjusting algorithms as necessary to maintain accuracy.
- Hybrid Approach: Combine AI-based scoring with human insights for better results, especially in complex scenarios where human intuition is necessary.
- Transparent Feedback Loops: Create feedback mechanisms where sales teams can provide insights into AI scoring to further refine and improve the models.
"AI is a powerful tool for lead scoring, but it requires continuous human oversight and regular recalibration to ensure its effectiveness."
Best Practices for Successful AI Lead Scoring
Best Practice | Description |
---|---|
Data Cleanliness | Ensure that all data fed into the AI model is accurate, complete, and free from biases to prevent inaccurate scoring. |
Cross-Validation | Regularly test models with diverse data sets to prevent overfitting and ensure they generalize well across different conditions. |
Domain Collaboration | Involve experts in defining the lead scoring criteria and in reviewing the AI model's output to ensure alignment with business objectives. |
Continuous Monitoring | Regularly monitor model performance and update the algorithm based on new insights and changing market dynamics. |