When Should We Use a Within-subjects T-test
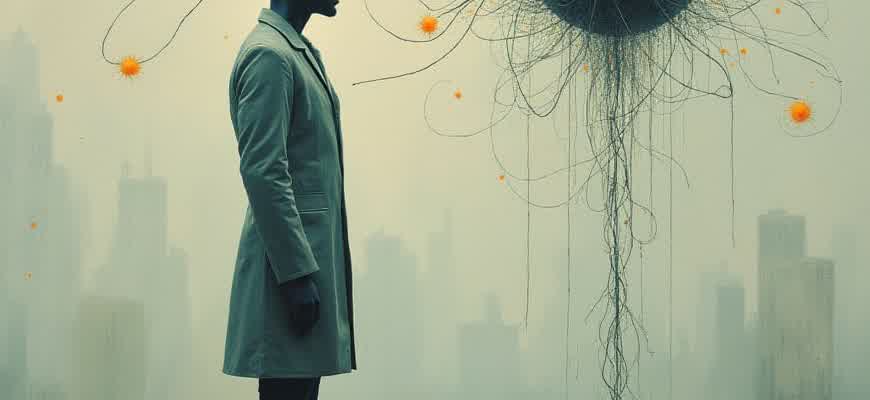
A within-subjects t-test is a statistical method employed to compare the means of two related groups or conditions. It is typically used when the same participants are exposed to multiple conditions or measurements over time. This design eliminates the variability between subjects, which can enhance the sensitivity of the test and lead to more reliable results.
Here are some situations when a within-subjects t-test is appropriate:
- Repeated measures on the same group: When each participant is measured under different conditions or at different times.
- Comparing performance across time: When you assess a group’s behavior at different points, for example, before and after an intervention.
- Control for between-subjects variability: Since each subject serves as their own control, the method minimizes the effect of individual differences.
One key advantage is that this approach requires fewer participants than a between-subjects t-test, as it uses the same subjects for all conditions. However, it is important to account for potential carryover effects or practice effects that may influence results. These can introduce bias if not properly controlled for in the study design.
Note: Always ensure that the assumptions for conducting a within-subjects t-test, such as normality of the differences between paired observations, are met before proceeding with the analysis.
When to Choose a Within-Subjects Design Over a Between-Subjects Design
In experimental research, the decision between using a within-subjects or between-subjects design is critical for ensuring the validity and power of the study. A within-subjects design, where the same participants are exposed to all conditions, can be particularly useful when the goal is to reduce the impact of individual differences on the results. However, this design is not always suitable, and certain factors must be considered before making a decision.
There are specific scenarios where a within-subjects design is more appropriate. These involve situations where the researcher wants to measure changes in the same participants under different conditions, and where minimizing variability due to individual differences is essential for drawing conclusions. Below are key factors to help determine when a within-subjects design is the best choice.
When to Opt for a Within-Subjects Design
- Reduction of Variability: If individual differences are likely to create noise in the data, a within-subjects design allows for a direct comparison of the same participants across conditions. This minimizes error variance attributed to inter-participant differences.
- Cost Efficiency: With fewer participants needed, a within-subjects design is often more cost-effective, especially when working with a limited pool of participants.
- Measuring Within-Subject Changes: When the study focuses on how participants’ responses evolve over time or in reaction to different conditions, within-subjects designs are ideal.
- High Sensitivity to Small Effects: This design is often more sensitive to detecting small effects because each participant acts as their own control group.
When to Avoid a Within-Subjects Design
- Order Effects: If the order in which participants experience conditions might influence their responses (e.g., fatigue, practice effects), a within-subjects design may introduce bias unless counterbalancing techniques are carefully employed.
- Carryover Effects: If the effects of one condition persist and influence responses to subsequent conditions, a within-subjects design may not be appropriate unless proper washout periods or randomization are used.
- Ethical Concerns: In some cases, asking participants to undergo multiple conditions may be too demanding or could lead to ethical concerns, such as overburdening participants or exposing them to repeated risks.
"The decision between within-subjects and between-subjects designs should be guided by a clear understanding of the research question, the nature of the variables, and practical considerations such as the availability of participants and resources."
Comparing Within-Subjects and Between-Subjects Designs
Factor | Within-Subjects Design | Between-Subjects Design |
---|---|---|
Number of Participants | Fewer participants needed | More participants required |
Variability | Reduces variability due to individual differences | More variability due to individual differences |
Order Effects | Risk of order effects (fatigue, learning) | No order effects, as different participants are used for each condition |
Suitability | When measuring change within participants | When random assignment to groups is essential |
How to Ensure the Assumptions of a Within-subjects T-test Are Met
A within-subjects t-test, also known as a paired sample t-test, compares the means of two related groups. This method is useful when measurements are taken from the same participants under different conditions. However, in order for the results to be valid, several assumptions need to be satisfied. Ensuring these assumptions are met is crucial for the reliability of the test and the interpretation of the results.
There are three key assumptions to consider when using a within-subjects t-test: normality of the differences between conditions, independence of the observations, and the absence of extreme outliers. Let’s explore how to check and meet each assumption.
1. Normality of Differences
One of the primary assumptions is that the differences between paired scores follow a normal distribution. If this assumption is violated, the results of the t-test might be misleading. Here's how you can assess and address this assumption:
- Visual inspection: Create a histogram or Q-Q plot of the differences between conditions. This can provide a visual indication of whether the data is approximately normally distributed.
- Shapiro-Wilk test: This statistical test can be used to formally assess the normality of the differences. If the p-value is greater than 0.05, you can assume normality. Otherwise, consider transforming the data.
- Transformation of data: If normality is not present, consider applying transformations like log or square root to the data to achieve normality.
Tip: It’s important to note that the assumption of normality becomes less critical as sample size increases, due to the Central Limit Theorem.
2. Independence of Observations
The second assumption is that the observations are independent, meaning that each participant’s scores should not influence another's. This assumption is generally met if the data collection process is carefully designed. To ensure this:
- Random sampling: Ensure that participants are randomly assigned to different conditions to avoid systematic biases.
- Clear separation between conditions: If participants are exposed to multiple conditions, make sure that they are not influenced by previous exposures or experiences.
3. Absence of Outliers
Extreme values can distort the results of the t-test. Outliers in the differences between conditions are particularly problematic, as they can significantly skew the results. To check for and address outliers:
- Boxplot analysis: Create a boxplot of the differences between the paired conditions. Outliers will be displayed as individual points outside the "whiskers" of the boxplot.
- Remove or adjust outliers: If identified, consider removing outliers or using statistical methods like winsorizing to limit their impact.
Summary Table
Assumption | How to Ensure It |
---|---|
Normality of Differences | Use visual methods like histograms and Q-Q plots, or apply the Shapiro-Wilk test. Consider transforming the data if necessary. |
Independence of Observations | Ensure random sampling and avoid overlap between conditions that could influence participant behavior. |
Absence of Outliers | Use boxplots to identify outliers. Consider removing or adjusting extreme values as necessary. |
Analyzing the Impact of Repeated Measurements on Statistical Power
Repeated measures designs, in which the same participants are tested multiple times under different conditions, have a profound effect on statistical power. One of the key advantages of such designs is the reduction in the variability introduced by between-subject differences, leading to more accurate detection of treatment effects. When measurements are repeated within the same individual, the influence of random error is minimized, thus enhancing the ability to detect meaningful differences.
However, repeated measurements also come with their own set of challenges, particularly concerning the assumptions of independence. If not properly addressed, correlated errors across repeated measurements can undermine the effectiveness of statistical tests. This article explores how repeated measures can influence the statistical power of within-subjects t-tests, emphasizing both the advantages and potential pitfalls of this design.
Key Factors Influencing Statistical Power
- Reduction of Between-Subject Variability: The use of the same participants across conditions reduces the variability associated with individual differences, increasing the test’s sensitivity to detecting treatment effects.
- Increased Statistical Power: By minimizing error variance through repeated testing, within-subject designs typically result in higher statistical power compared to between-subjects designs of equivalent size.
- Correlation Between Measurements: The assumption that repeated measures are independent can be violated if there is a high correlation between measurements, which may distort the results and reduce power.
Challenges with Repeated Measures Designs
- Practice or Fatigue Effects: Participants may experience changes in performance due to learning (practice effects) or tiredness (fatigue effects), which could skew the results and reduce the power of the analysis.
- Time-Related Confounding: If there is a time-related factor affecting performance, such as a change in environmental conditions, these factors need to be controlled to prevent them from masking treatment effects.
- Complexity of Data Analysis: Properly accounting for the correlation between repeated measurements requires specialized statistical techniques, such as mixed-effects models or appropriate adjustments in the t-test, to maintain the validity of the analysis.
Practical Example
Condition | Mean Score | Standard Deviation | Measurement Correlation |
---|---|---|---|
Pre-Treatment | 65 | 10 | 1.0 |
Post-Treatment | 75 | 12 | 0.8 |
By accounting for within-subject correlations, researchers can more accurately estimate treatment effects, improving the power of statistical tests while minimizing potential bias from extraneous variables.
How to Handle Practice and Fatigue Effects in Within-subjects Studies
In a within-subjects design, each participant is exposed to all conditions of the experiment, which increases the risk of confounding variables like practice and fatigue effects. These two factors can significantly distort the results, making it harder to distinguish between the true effects of the experimental manipulation and those arising from the participants' experience or exhaustion over time. Understanding how to address these issues is crucial for ensuring valid results.
Practice effects occur when participants' performance improves simply because they become more familiar with the task. On the other hand, fatigue effects can cause a decline in performance as participants grow tired throughout the experiment. Both of these factors can bias data, leading to misleading conclusions if not properly controlled. Here are some strategies to mitigate these influences:
- Counterbalancing: Randomly varying the order of conditions for each participant ensures that practice and fatigue effects are distributed evenly across all conditions. This method helps prevent one condition from always being influenced by prior experience or tiredness.
- Breaks: Incorporating breaks throughout the experiment can alleviate fatigue and maintain participant focus. Short, frequent rest periods allow participants to recover and maintain consistent performance across conditions.
- Training sessions: Providing participants with a practice session before the actual experiment can help them get accustomed to the task. This minimizes the impact of practice effects during the experiment itself.
- Extended Study Duration: When possible, lengthening the study duration across multiple sessions can reduce fatigue effects. This also allows participants to return to the task after a period of rest.
Key Points to Remember
Practice and fatigue effects are critical concerns in within-subjects studies. Both can compromise the internal validity of the experiment if not properly managed.
Factor | Recommended Mitigation |
---|---|
Practice Effects | Counterbalancing, training sessions |
Fatigue Effects | Breaks, extended study duration |
Calculating the Degrees of Freedom for a Within-subjects T-test
In statistical analysis, calculating the degrees of freedom (df) for a within-subjects t-test is essential for determining the critical value of t. The degrees of freedom help assess the variability in the differences between the conditions or measurements within the same subjects. This calculation is crucial because it affects the shape of the t-distribution and, ultimately, the statistical significance of the results.
In a within-subjects design, each participant is exposed to all experimental conditions. This means that the same individual’s performance is measured under different conditions, leading to paired observations. To calculate the degrees of freedom, we need to focus on the number of pairs of scores, not individual scores themselves.
Steps to Calculate Degrees of Freedom
- Determine the number of participants (n).
- Calculate the number of differences between the paired measurements. For each participant, subtract the score in one condition from the score in the other condition.
- The degrees of freedom are calculated using the formula: df = n - 1, where "n" is the number of participants.
For example, if there are 15 participants in your experiment, the degrees of freedom for the within-subjects t-test would be 15 - 1 = 14.
Important: The degrees of freedom are based on the number of participants, not the number of observations. Each participant contributes one difference, not multiple data points, to the calculation.
Example Calculation
Number of Participants (n) | Degrees of Freedom (df) |
---|---|
10 | 9 |
20 | 19 |
50 | 49 |
This simplified method ensures that the degrees of freedom account for the repeated measures design, leading to more accurate statistical testing.
Interpreting p-values in the Context of Within-subjects Designs
In within-subjects designs, where the same participants are exposed to different conditions, the interpretation of p-values requires careful consideration. Since each participant serves as their own control, the variance between individuals is reduced, and the statistical power of the test is often increased. However, the p-value interpretation in this context is nuanced due to the dependence of observations within each participant. It is essential to consider the effects of these dependencies to avoid misinterpreting the results.
The p-value, representing the probability of obtaining results at least as extreme as those observed, given that the null hypothesis is true, plays a crucial role in decision-making. In the case of within-subjects designs, a significant p-value typically indicates that the difference between conditions is unlikely to have occurred by chance. However, interpreting this p-value requires understanding the underlying statistical assumptions, such as the normality of differences between conditions and the absence of systematic biases.
Key Considerations in p-value Interpretation
- Dependency of measurements: The p-value must account for the correlation between repeated measures from the same participants. This is typically managed by using paired t-tests or other methods that adjust for within-subject variance.
- Effect size: A small p-value alone does not imply a large effect. It is important to also consider the magnitude of the effect through measures such as Cohen's d or partial eta-squared.
- Multiple comparisons: In studies with multiple conditions, the risk of Type I errors increases. Adjustments to the p-value (e.g., Bonferroni correction) are often needed to control for this risk.
Practical Example
Condition | Mean Difference | p-value |
---|---|---|
Condition 1 vs. Condition 2 | 2.5 | 0.04 |
Condition 2 vs. Condition 3 | 1.0 | 0.23 |
When interpreting the p-value from a within-subjects design, the dependence between measurements must be taken into account. A significant p-value indicates a likely true effect, but further statistical analysis is needed to fully assess the magnitude and implications of the findings.
Common Pitfalls When Using a Within-subjects T-test and How to Avoid Them
Conducting a within-subjects t-test can provide valuable insights when studying changes within the same group of participants. However, there are several common mistakes researchers often make when applying this statistical method. Understanding these pitfalls and learning how to avoid them is crucial for accurate results and valid conclusions. Below are key challenges and recommendations for overcoming them.
One major issue arises when researchers ignore the assumption of sphericity, which is the condition that the variances of the differences between all possible pairs of conditions should be equal. Violation of this assumption can lead to inaccurate p-values and misleading conclusions. Another common mistake is failing to properly account for carryover effects, where earlier conditions affect the outcomes of later ones. Proper counterbalancing and randomization can help mitigate such effects.
Key Pitfalls and Strategies for Avoidance
- Ignoring Sphericity Assumption: Ensure that the variances of differences are consistent across conditions. If violated, use a correction like the Greenhouse-Geisser adjustment to modify p-values.
- Carryover Effects: Randomize the order of conditions to minimize the influence of previous conditions on later ones.
- Small Sample Sizes: Insufficient sample sizes can lead to low power and unreliable results. Aim for a sufficiently large sample to detect meaningful effects.
- Misinterpreting Significant Results: Always check the effect size along with p-values to assess the practical significance of the findings.
Important Considerations
Remember: A within-subjects design reduces variability due to individual differences, but it introduces the potential for order and carryover effects, which can bias the results if not carefully managed.
Checklist for Best Practices
- Check for sphericity before running the test.
- Implement counterbalancing or randomization to control for order effects.
- Ensure a large enough sample size for statistical power.
- Report both p-values and effect sizes to convey the true significance of findings.
Example: Comparing Reaction Times Across Two Conditions
Condition | Mean Reaction Time (ms) | Standard Deviation |
---|---|---|
Condition 1 | 450 | 20 |
Condition 2 | 470 | 22 |
How to Report Results of a Within-Subjects T-Test in Academic and Professional Writing
When presenting the results of a within-subjects t-test in academic or professional writing, it is crucial to follow established reporting standards. These results help researchers draw conclusions about the differences between related groups or conditions. Reporting should be clear, concise, and follow a logical format that allows readers to easily interpret the findings. Typically, results are presented using the t-value, degrees of freedom, p-value, and the means of the compared conditions.
To ensure clarity, the presentation of the test results should include the necessary statistical values and a concise interpretation of their meaning. Below is a structured approach to reporting the outcomes of a within-subjects t-test.
Key Elements to Include in Reporting
- t-value: Indicates the difference between the means relative to the variation within the sample.
- degrees of freedom (df): Typically calculated as the number of pairs minus one.
- p-value: Reflects the probability that the observed results occurred by chance. A value below 0.05 usually indicates statistical significance.
- means of both conditions: The average scores for the two conditions being compared.
- effect size: This helps convey the magnitude of the difference and is commonly reported using Cohen’s d for within-subjects designs.
Example of Reporting Results
Below is a sample presentation of results for a within-subjects t-test:
Measure | Condition 1 Mean | Condition 2 Mean | t-value | p-value | Cohen’s d |
---|---|---|---|---|---|
Reaction Time | 500 ms | 450 ms | 2.45 | 0.03 | 0.65 |
Example of Proper Reporting Format
The results of the within-subjects t-test indicated a significant difference in reaction times between Condition 1 (M = 500 ms) and Condition 2 (M = 450 ms), t(29) = 2.45, p = 0.03, Cohen’s d = 0.65. This suggests that the experimental manipulation had a moderate effect on reaction time.
Remember to report the test statistics in a format that allows the reader to easily evaluate the significance and effect size of the results. This will ensure that your conclusions are supported by the data.