How Can Ai Predict Customer Churn in Email Marketing
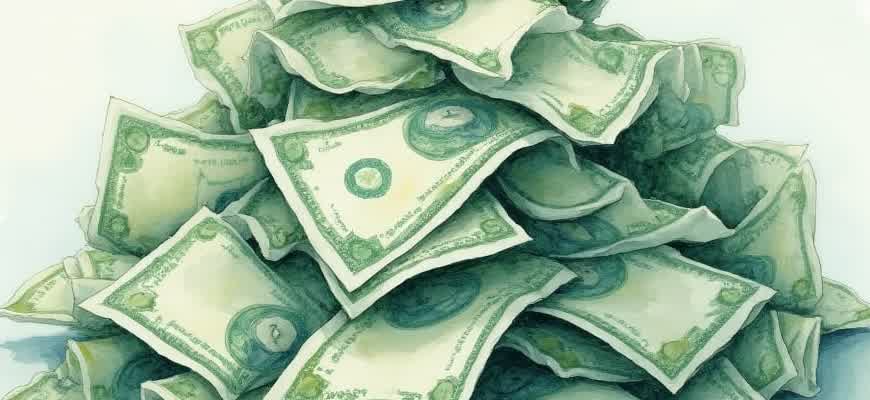
Artificial intelligence has revolutionized the way businesses approach customer retention in email marketing. By analyzing vast amounts of customer data, AI can identify patterns and behaviors that indicate when a subscriber is likely to disengage. This predictive capability allows marketers to take proactive measures, improving engagement rates and reducing churn.
AI models leverage multiple data sources to predict churn, often relying on historical customer interactions, email engagement metrics, and demographic information. These models use machine learning algorithms to recognize patterns in the data, helping marketers identify which subscribers are at risk of unsubscribing or disengaging. The most common factors include:
- Frequency of email opens
- Click-through rates
- Subscription duration
- Product or service interactions
"AI-driven predictions allow for real-time insights, enabling businesses to tailor their communication strategies to retain customers before they churn."
One way to measure and manage churn predictions is by using a predictive analytics dashboard. Here’s an example of how such a dashboard might look:
Customer ID | Engagement Score | Churn Probability (%) | Action Suggested |
---|---|---|---|
1001 | 75 | 35 | Send personalized re-engagement offer |
1002 | 40 | 60 | Initiate win-back campaign |
1003 | 90 | 10 | Continue regular engagement |
How AI Can Forecast Customer Churn in Email Marketing
In the realm of email marketing, customer retention is crucial for long-term success. Artificial intelligence (AI) can be an effective tool for predicting when customers are likely to disengage, allowing marketers to take proactive steps to maintain engagement. AI analyzes vast amounts of data to detect patterns and trends that human marketers may miss, providing valuable insights into potential churn behavior.
AI-driven models use a variety of customer data points, such as email open rates, click-through rates, and previous interactions, to predict future behavior. By applying machine learning algorithms, AI can segment customers based on their likelihood to churn and suggest targeted strategies to retain them. This process reduces the uncertainty surrounding customer retention efforts and boosts the efficiency of email marketing campaigns.
Key Factors for AI to Predict Churn
- Email Engagement: Low open or click rates over time may indicate a higher risk of churn.
- Purchase History: A decline in repeat purchases often correlates with customer disengagement.
- Customer Interaction Patterns: AI can detect sudden changes in behavior, such as a drop in email responses or the frequency of visits to a site.
AI Predictive Models for Churn
AI models rely on several machine learning techniques to predict churn. Some of the most effective approaches include:
- Logistic Regression: A statistical method used to predict the probability of churn based on customer behavior.
- Decision Trees: Models that break down customer data into "if-then" rules to classify users by their churn risk.
- Random Forests: An ensemble of decision trees that increases prediction accuracy by combining multiple models.
“AI provides a data-driven approach to predicting churn, enabling marketers to tailor campaigns that re-engage customers before it's too late.”
Example of AI Churn Prediction Model
Metric | Threshold | Risk of Churn |
---|---|---|
Email Open Rate | Below 20% | High |
Purchase Frequency | Less than once per month | Moderate |
Last Interaction Date | More than 90 days ago | Very High |
Identifying the Key Indicators of Customer Churn in Email Campaigns
Understanding when a customer is likely to disengage from email marketing campaigns is crucial for retaining a loyal customer base. By identifying early signs of churn, marketers can take proactive steps to prevent it. Customer churn often manifests through behavioral shifts, which can be tracked and analyzed through email engagement metrics. These metrics provide insights into a customer’s interest, satisfaction, and likelihood of unsubscribing or ignoring future emails.
To effectively predict churn, it’s important to monitor certain customer behaviors and interactions with email campaigns. These behaviors are often subtle but highly indicative of a customer’s intentions. By analyzing these patterns, marketers can determine the right time to intervene with personalized content, incentives, or re-engagement strategies.
Key Behavioral Indicators to Track
- Open Rate Decline: A noticeable decrease in the percentage of emails opened over time may signal waning interest or engagement. Low open rates often precede higher rates of unsubscription.
- Click-Through Rate (CTR) Drop: A decline in the number of clicks within emails indicates reduced interaction and could be an early sign of churn. If a customer is no longer clicking on call-to-action links, they might be losing interest.
- Unsubscribes: An increase in the number of unsubscriptions is a clear signal of disengagement and potential churn. Tracking unsubscribes by email type or campaign can help identify specific content that drives customers away.
- Frequency of Email Skimming: Customers who open emails but do not interact with the content may be losing interest. If this behavior is consistent over time, it suggests the likelihood of churn.
Customer Behavior Patterns to Watch
- Frequency of Interaction: Customers who frequently engage with your emails are more likely to remain loyal. A drop in interaction frequency is a warning sign that they may soon disengage.
- Time of Engagement: The time it takes for a customer to engage with emails can be revealing. Longer response times or delayed engagement can indicate reduced priority given to your communications.
- Response to Incentives: If customers are no longer responding to special offers or incentives, it may reflect a diminished interest in the product or service.
"Tracking these key indicators helps marketers identify patterns that suggest a customer is likely to churn, enabling timely and targeted interventions."
Summary of Key Indicators
Behavioral Indicator | Sign of Potential Churn |
---|---|
Declining Open Rates | Decreasing engagement or interest in emails |
Drop in Click-Through Rates | Reduction in interaction with email content |
Increased Unsubscribes | Direct signal of disengagement |
Slow Response Times | Indicates reduced priority given to emails |
How Machine Learning Models Analyze Subscriber Behavior
Machine learning models use advanced techniques to analyze subscriber activity in email campaigns, identifying patterns that can predict churn. These models focus on a wide range of behavioral data, such as email open rates, click-through rates, time spent on content, and the frequency of interactions. By processing this data, they uncover hidden correlations that can indicate whether a subscriber is likely to disengage or remain loyal to the brand.
These models are trained to distinguish between typical and atypical behavior by examining factors like how often a subscriber interacts with emails, the types of content they engage with, and their overall email engagement history. The analysis of this data helps to pinpoint subscribers who are at risk of unsubscribing or becoming inactive, allowing businesses to take proactive measures.
Key Data Points Analyzed by Machine Learning Models
- Email Open Rate: A decrease in open rates often signals disengagement.
- Click-through Rate (CTR): Measures how many recipients click links within emails.
- Time Spent on Email Content: Shorter engagement times may indicate a loss of interest.
- Unsubscribe Requests: A direct indicator of churn.
- Frequency of Interaction: Less frequent interaction can signify a decline in interest.
Steps in Behavior Analysis Using Machine Learning
- Data Collection: Collecting historical data of subscriber interactions with emails.
- Feature Engineering: Identifying key features that are indicative of subscriber behavior (e.g., frequency of clicks, engagement patterns).
- Model Training: Training machine learning models using past data to recognize patterns of churn.
- Prediction: Making predictions based on new data, identifying subscribers who are most likely to churn.
- Actionable Insights: Providing businesses with recommendations to prevent churn, such as personalized content or re-engagement campaigns.
Machine learning models can detect subtle shifts in user behavior that may not be immediately visible through traditional analysis methods, providing a more accurate prediction of subscriber churn.
Example: Predicting Subscriber Churn
Behavioral Factor | Risk Level |
---|---|
Email Open Rate Decrease | High |
Click-through Rate Below Average | Moderate |
Unsubscribe Requests | Very High |
Frequent Skipping of Promotions | Moderate |
Using AI to Identify Subscribers Likely to Unsubscribe
Artificial intelligence can significantly enhance email marketing campaigns by predicting subscriber behavior, including the likelihood of unsubscribing. By analyzing large sets of data, AI can pinpoint early indicators of churn, enabling marketers to target high-risk subscribers with tailored retention strategies. Machine learning algorithms, for example, can identify patterns in user engagement, helping to segment audiences based on their likelihood of unsubscribing.
AI-driven segmentation allows for the creation of highly personalized campaigns aimed at re-engaging users who show signs of disengagement. By tracking metrics such as open rates, click-through rates, and the frequency of email interactions, AI tools can detect subtle shifts in subscriber activity. This segmentation empowers marketers to take timely actions to prevent churn, such as sending special offers or content that appeals to the specific interests of these at-risk subscribers.
AI Segmentation Process
- Engagement Drop Detection: AI can monitor engagement metrics, detecting sudden decreases in open and click rates.
- Predictive Modeling: Using historical data, AI can predict future unsubscribe behavior, identifying users who are more likely to leave.
- Personalized Content Recommendations: AI can recommend content or products based on past behavior, helping to re-engage users who show signs of disengagement.
AI does not just react to current behavior; it anticipates future actions, providing marketers with the ability to proactively manage retention efforts.
Segmentation Criteria
- Frequency of Interaction: Low frequency may indicate a risk of unsubscribing.
- Recent Purchases or Actions: Lack of recent activity could signal a disconnection with the brand.
- Time Since Last Email Open: A longer gap could mean a diminishing interest in future communications.
- Previous Unsubscribes: Users who have unsubscribed before might be more prone to doing it again.
Risk Level Table
Risk Level | Criteria | Action |
---|---|---|
High | Low engagement + long time since last open | Send re-engagement offer with personalized content |
Medium | Moderate engagement + slight decrease in activity | Introduce targeted incentives or updates to maintain interest |
Low | Consistent engagement + recent interaction | Continue with regular communications, ensuring value is clear |
Predicting Customer Churn Through Engagement Metrics in Emails
Email marketing is one of the most effective ways to engage with customers. However, understanding when a customer might disengage can be challenging. By analyzing engagement metrics in email campaigns, businesses can forecast potential churn. Key metrics such as open rates, click-through rates (CTR), and unsubscribe rates provide a window into how well customers are interacting with content. These indicators can help to identify early signs of disinterest and reduce the risk of losing valuable clients.
Effective prediction of churn based on engagement requires not just monitoring individual actions, but understanding patterns over time. For instance, a sudden drop in open rates or a slow decline in click-through rates might signal a customer’s shift in interest. With the help of AI and machine learning algorithms, these behaviors can be analyzed and used to predict whether a customer is likely to stop engaging with email campaigns, thus allowing businesses to take proactive steps in re-engaging or retaining them.
Key Engagement Metrics to Track
- Open Rates: A decrease in open rates suggests that recipients are losing interest in the subject matter or frequency of emails.
- Click-Through Rates (CTR): Low or declining CTR can indicate that customers are no longer engaging with the content in emails.
- Unsubscribe Rates: An increase in unsubscribes is a clear sign that customers are opting out of email communications, which can signal potential churn.
- Bounce Rates: Higher bounce rates may indicate poor list hygiene or a lack of relevance to the audience, possibly leading to disengagement.
How to Use AI to Predict Churn
AI models can process vast amounts of engagement data to detect patterns that are not immediately apparent. By segmenting customers based on their engagement behaviors, businesses can create predictive models to identify high-risk customers. For example:
- Behavioral Analysis: Machine learning algorithms analyze individual interactions, such as email opens, link clicks, and time spent reading emails, to assess overall engagement.
- Historical Data Comparison: By comparing current behavior to past trends, AI can predict which customers are likely to churn based on significant changes in engagement patterns.
- Customer Segmentation: AI can cluster customers into different segments based on their engagement levels, helping businesses target high-risk customers with tailored retention strategies.
Impact of Engagement Metrics on Churn Prediction
Metric | Churn Indicator | Actionable Insight |
---|---|---|
Open Rate Drop | Customer loses interest | Test new subject lines or send less frequent emails |
Decline in CTR | Customer disengagement | Personalize content or offer exclusive deals |
Increased Unsubscribes | Customer dissatisfaction | Survey customers to understand their reasons |
"By analyzing email engagement metrics, businesses can predict which customers are likely to churn, allowing them to take proactive steps to retain them before it’s too late."
Building a Churn Prediction Model with Historical Data
Creating a churn prediction model starts with gathering and analyzing historical customer data, which serves as the foundation for identifying patterns and behaviors that contribute to customer attrition. This data typically includes customer interactions, purchase history, email engagement metrics, and other relevant touchpoints. By understanding these elements, businesses can determine which factors are most strongly correlated with churn, helping to prioritize actions for retention.
Once the data is collected, the next step is to prepare it for modeling. Cleaning and preprocessing the data is crucial, as raw datasets often contain missing values, inconsistencies, or irrelevant features. The goal is to create a dataset that can accurately reflect customer behavior and trends that predict potential churn events.
Key Steps in Building the Model
- Data Collection: Gather historical data, including email interactions, purchase frequency, and engagement metrics.
- Feature Engineering: Identify key features that may influence churn, such as frequency of email opens, click-through rates, or previous purchase patterns.
- Data Preprocessing: Clean the data by handling missing values, normalizing numerical data, and encoding categorical variables.
- Model Selection: Choose an appropriate machine learning model, such as logistic regression, decision trees, or random forests, to classify churn likelihood.
- Training and Testing: Split the data into training and test sets to evaluate model performance and avoid overfitting.
Model Evaluation Metrics
After the model is trained, its performance needs to be assessed. Some common evaluation metrics include:
Metric | Description |
---|---|
Accuracy | Percentage of correctly predicted churn events. |
Precision | Proportion of true positive churn predictions out of all predicted churns. |
Recall | Proportion of actual churn events identified correctly by the model. |
F1-Score | The harmonic mean of precision and recall, balancing both metrics. |
Effective churn prediction not only relies on historical data but also on the continuous monitoring and adjustment of the model to account for evolving customer behavior.
Integrating AI Predictions into Automated Email Campaigns
AI-powered insights can enhance the effectiveness of email campaigns by anticipating customer churn. By identifying patterns and behaviors that predict disengagement, AI can enable marketers to create targeted, personalized messages that prevent churn before it occurs. Automated email systems can leverage these predictions, sending tailored content based on individual customer tendencies, engagement history, and predicted behavior. This ensures that the emails are relevant and timely, increasing the chances of retaining at-risk customers.
By integrating AI predictions into automation, businesses can optimize their campaigns with minimal manual effort. The AI models analyze data such as email open rates, click-through rates, and purchase history to determine which users are likely to churn. Once these users are identified, the system automatically triggers specific campaigns designed to re-engage them and boost retention rates.
Key Benefits of AI Integration in Email Automation
- Targeted Engagement: AI identifies customers who are at risk of disengagement and triggers personalized re-engagement emails.
- Optimized Campaign Timing: AI analyzes customer behavior to determine the best time to send an email for maximum impact.
- Increased Efficiency: Automation ensures that emails are sent without manual intervention, saving time while delivering relevant content.
Steps to Integrate AI Predictions into Email Marketing
- Data Collection: Gather customer data, including past interactions, purchase history, and behavioral trends.
- Train AI Models: Use the data to train machine learning models that predict customer churn and engagement likelihood.
- Set Triggers: Define email triggers based on the predictions made by AI, such as offering discounts to at-risk customers.
- Automate Campaigns: Integrate the AI-driven insights into email automation platforms for real-time campaign execution.
“AI models continuously learn from customer interactions, improving over time to provide increasingly accurate churn predictions.”
Example of AI-Powered Email Workflow
Step | Action |
---|---|
1 | Identify at-risk customers using churn prediction algorithms. |
2 | Trigger personalized re-engagement emails, such as exclusive offers or content. |
3 | Measure the success of each campaign, adjusting AI models for better predictions. |
Measuring the Impact of AI Predictions on Subscriber Retention
AI-driven insights offer a powerful tool in understanding customer behavior, particularly when applied to email marketing. By predicting churn, marketers can develop strategies that prevent subscriber attrition and increase retention rates. The accuracy of AI predictions plays a key role in tailoring engagement tactics, improving campaign effectiveness, and enhancing overall customer loyalty.
One way to measure the success of these AI-based predictions is by analyzing retention metrics before and after implementing AI-powered insights. By closely monitoring key performance indicators (KPIs), marketers can assess whether predictive models are effectively guiding their retention strategies.
Key Metrics to Evaluate AI Impact on Retention
- Retention Rate: A primary indicator of subscriber loyalty, showing the percentage of subscribers who remain engaged over time.
- Engagement Levels: Tracking click-through rates (CTR) and open rates of emails to determine how well subscribers are interacting with content after predictions are applied.
- Unsubscribe Rate: A decrease in this rate suggests that the AI-driven strategies are helping to keep subscribers engaged and satisfied.
Comparison of Retention Rates Before and After AI Integration
Metric | Before AI Integration | After AI Integration |
---|---|---|
Retention Rate | 70% | 80% |
Engagement Rate | 45% | 55% |
Unsubscribe Rate | 5% | 3% |
"By analyzing the change in key retention metrics, marketers can assess the tangible benefits of AI-based churn predictions on email campaigns."
Overall, AI-driven predictions contribute to more precise targeting and personalized content, leading to stronger subscriber retention. The real value of these technologies lies in their ability to adapt and improve marketing efforts based on real-time data, fostering long-term loyalty among customers.
Overcoming Challenges in AI-Driven Churn Prediction for Email Marketing
Predicting customer churn in email marketing using artificial intelligence involves several hurdles that can impact the accuracy and effectiveness of predictions. One key challenge is the quality of data used for training AI models. Inaccurate or incomplete data can lead to skewed predictions, resulting in a failure to properly identify potential churners. Furthermore, customer behaviors change over time, requiring constant model updates to stay relevant. Thus, continuous data monitoring and retraining of the AI systems are essential for maintaining precision in churn prediction.
Another significant challenge lies in the complexity of human behavior, which AI must interpret and predict accurately. Email marketing campaigns must account for a variety of factors like customer preferences, frequency of interaction, and engagement level. AI models need to understand these variables and adapt to shifts in customer behavior to prevent churn. However, training AI systems to effectively handle such nuanced and dynamic data often requires advanced algorithms and specialized expertise.
Key Obstacles to Overcome
- Data Quality and Completeness: Missing or incorrect customer data can severely hinder the AI's ability to make reliable predictions.
- Model Overfitting: If the AI model is too finely tuned to historical data, it may not generalize well to new customers, resulting in inaccurate churn predictions.
- Customer Behavior Dynamics: The constantly changing nature of customer engagement can complicate long-term predictions and require frequent model adjustments.
Approaches to Tackle These Challenges
- Regular Data Audits: Continuously verify and clean customer data to ensure high-quality input for AI models.
- Incremental Learning: Use techniques that allow AI models to adapt to new data without forgetting previously learned patterns.
- Behavioral Segmentation: Implement segmentation strategies to better understand diverse customer groups and tailor predictive models accordingly.
"AI models must continuously evolve alongside changes in customer behavior to maintain accurate churn predictions. Without constant adaptation, predictions can quickly become outdated."
Data-Driven Solutions
Solution | Description |
---|---|
Predictive Analytics | Use machine learning models to forecast customer behavior based on historical interactions and trends. |
Customer Feedback Integration | Incorporate customer survey data and feedback to gain deeper insights into the reasons behind churn. |