Ai Driven Lead Generation Tools
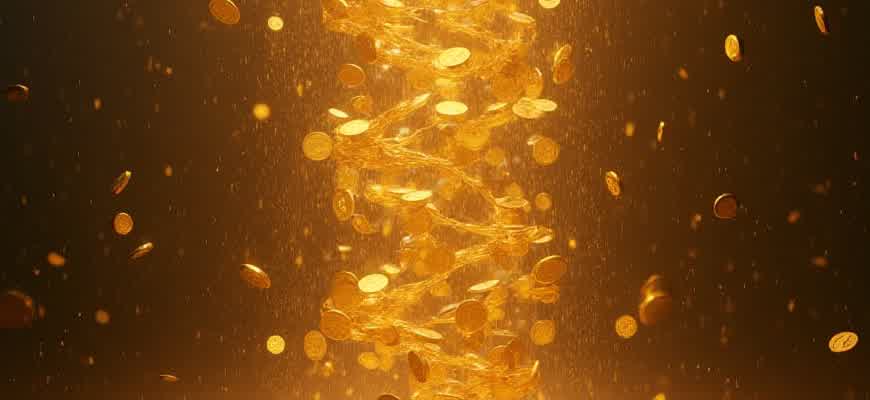
In recent years, businesses have increasingly relied on artificial intelligence (AI) to streamline and enhance the lead generation process. By leveraging advanced algorithms, AI-powered platforms can automate key aspects of lead discovery, nurturing, and conversion, drastically improving efficiency and accuracy. These tools help marketers identify high-quality prospects, predict their behavior, and personalize outreach efforts, ultimately driving higher engagement rates.
Key Benefits of AI in Lead Generation:
- Data-Driven Insights: AI tools analyze large datasets to identify trends and patterns, making lead identification more targeted and effective.
- Automation of Outreach: Automated messages and follow-ups save time while ensuring timely responses to potential clients.
- Improved Conversion Rates: With AI’s ability to predict buyer intent, businesses can better prioritize leads that are most likely to convert.
Common Types of AI-Driven Lead Generation Tools:
- Chatbots: AI-powered chatbots can interact with website visitors, qualifying leads in real-time and gathering necessary information for follow-up.
- Predictive Analytics: These tools use historical data to forecast future buying behavior, enabling marketers to focus on high-value leads.
- Lead Scoring Systems: AI algorithms assign a score to each lead based on various engagement factors, helping teams prioritize follow-ups more effectively.
"AI-driven lead generation tools empower businesses to not only automate tedious tasks but also enhance their lead quality, leading to a more efficient and profitable sales process."
AI-Driven Lead Acquisition Tools
AI-based solutions have revolutionized the way businesses capture and engage potential clients. By utilizing advanced algorithms, these tools can automatically identify and qualify leads, improving both the efficiency and effectiveness of marketing campaigns. Rather than relying on traditional methods, businesses now leverage machine learning and predictive analytics to uncover prospects who are more likely to convert.
From automating outreach to segmenting target audiences with precision, AI-driven tools provide a highly scalable approach to lead generation. These platforms gather data from a variety of sources, process it, and deliver actionable insights that can significantly reduce manual effort and improve sales outcomes.
Key Features of AI-Enhanced Lead Generation
- Lead Scoring: AI tools assess the likelihood of a prospect converting, allowing sales teams to prioritize high-value leads.
- Automated Outreach: Using natural language processing (NLP), AI can craft personalized messages and engage leads without human intervention.
- Data Integration: These systems pull from multiple data sources (social media, CRM, etc.) to build a comprehensive lead profile.
"AI-driven lead generation not only streamlines processes but also ensures that the leads generated are the most promising, which leads to higher conversion rates and shorter sales cycles."
AI Tools in Lead Generation: How They Work
- Data Collection: AI tools begin by gathering data from various online platforms and interactions with prospects.
- Predictive Analytics: These platforms use algorithms to analyze trends and predict which leads are most likely to convert based on historical data.
- Automated Follow-ups: With machine learning, AI can determine the best time and method to follow up with leads, increasing the chance of engagement.
Comparison of Popular AI Lead Generation Tools
Tool | Key Feature | Best For |
---|---|---|
HubSpot | AI-powered email and CRM integration | Small to medium businesses |
Leadsquared | AI-based lead scoring and behavioral insights | Enterprises and growth-stage companies |
Clearbit | Real-time lead enrichment with AI | Marketing teams needing rich data profiles |
How AI Optimizes Lead Scoring for Sales Teams
AI-powered tools have revolutionized the process of lead scoring by analyzing vast amounts of data to predict the likelihood of a lead converting into a paying customer. Traditional lead scoring methods often rely on basic demographics or past interactions, which can be limited in their predictive power. With the help of machine learning algorithms, these systems can now evaluate behavioral patterns, engagement levels, and other key metrics to provide sales teams with a much more accurate and actionable lead score.
By leveraging AI, sales teams can focus on high-value prospects, ensuring their efforts are directed where they are most likely to yield results. Machine learning models learn continuously from new data, which helps in adjusting lead scores based on changing market conditions and customer behaviors. This dynamic approach makes lead scoring more adaptive and effective over time.
AI Techniques for Lead Scoring Optimization
- Behavioral Analysis: AI evaluates how potential leads interact with your website, emails, and social media, identifying the actions that are most likely to indicate purchase intent.
- Predictive Analytics: By analyzing historical data, AI can predict future outcomes, assigning scores based on which leads are most likely to convert.
- Real-time Data Processing: AI systems can process incoming data in real time, allowing sales teams to prioritize leads as soon as new signals or interactions are detected.
Advantages for Sales Teams
- Increased Efficiency: Automated scoring reduces the time spent on manual lead qualification, allowing sales reps to focus on high-potential leads.
- Higher Conversion Rates: By targeting the most qualified leads, sales teams can increase their chances of converting leads into customers.
- Continuous Improvement: AI models improve over time as they process more data, leading to progressively better lead scoring and targeting accuracy.
"AI in lead scoring isn't just about automation–it's about smart prioritization and aligning sales efforts with the most promising opportunities."
Example: Lead Scoring Table
Lead Name | Engagement Score | Predictive Conversion Score | Overall Lead Score |
---|---|---|---|
Lead 1 | 85% | 90% | 88% |
Lead 2 | 70% | 65% | 68% |
Lead 3 | 50% | 40% | 45% |
Using Predictive Analytics to Identify High-Quality Leads
Predictive analytics can significantly enhance lead generation strategies by forecasting which prospects are most likely to convert into loyal customers. By leveraging data from past interactions, purchasing patterns, and other relevant factors, businesses can predict future behaviors and prioritize leads that show the highest potential for engagement. This method reduces the time spent on unqualified leads, allowing sales teams to focus on those most likely to bring value to the organization.
One of the key benefits of predictive analytics in lead generation is its ability to segment leads based on their likelihood of conversion. This approach uses advanced algorithms to analyze historical data, helping to uncover hidden trends and patterns that are not immediately apparent. By relying on these data-driven insights, companies can improve the efficiency of their marketing efforts and achieve a higher return on investment.
Steps to Leverage Predictive Analytics for High-Quality Leads
- Data Collection: Gather data from various sources, including CRM systems, social media, and past customer interactions.
- Behavior Analysis: Analyze past behaviors to determine patterns that predict future actions.
- Lead Scoring: Assign numerical values to leads based on their likelihood to convert, using factors such as engagement, demographics, and behavior.
- Segmentation: Group leads into categories, such as "high potential," "medium potential," and "low potential," to focus resources on the most promising prospects.
- Continuous Optimization: Regularly update the model to account for new trends and ensure that the lead scoring process remains accurate over time.
Key Metrics for Predictive Lead Scoring
Metric | Impact on Lead Quality |
---|---|
Engagement Level | Indicates the likelihood of continued interaction, crucial for identifying interested leads. |
Purchase History | Helps identify repeat customers or prospects with a higher chance of conversion. |
Demographic Fit | Ensures the lead matches the ideal customer profile, increasing conversion chances. |
"Predictive analytics allows businesses to focus on leads with the highest potential, optimizing marketing efforts and improving sales outcomes."
How Machine Learning Improves Lead Segmentation and Targeting
Machine learning (ML) significantly enhances the way businesses segment their potential customers and target them with tailored approaches. By analyzing vast amounts of data, ML algorithms can identify patterns and preferences that traditional methods might overlook. This allows for more precise grouping of leads, ensuring that marketing efforts are focused on those most likely to convert, which increases efficiency and ROI.
With machine learning, businesses can continuously refine their lead segmentation processes by learning from ongoing interactions. The more data is gathered, the better the models become at predicting lead behavior and categorizing them into segments that reflect their likelihood to engage with specific offers.
Benefits of ML-Driven Lead Segmentation
- Dynamic Segmentation: ML algorithms can adjust segmentation criteria in real-time based on new data, providing more relevant groupings.
- Improved Accuracy: By leveraging predictive models, businesses can reduce human error and misclassification of leads.
- Behavioral Insights: Machine learning models analyze user behavior, which helps in identifying key actions that lead to conversions.
- Personalization: More granular segmentation allows for highly personalized marketing messages, improving engagement rates.
How Machine Learning Improves Targeting
- Real-Time Adjustments: ML models adjust targeting strategies in real time based on interactions, ensuring that marketing efforts remain relevant.
- Predictive Targeting: Algorithms can predict which leads are more likely to engage, allowing businesses to prioritize high-value prospects.
- Data-Driven Decisions: Machine learning removes guesswork, enabling businesses to make informed decisions on which leads to target with greater precision.
Key Takeaways
Machine learning not only helps in segmenting leads but also optimizes targeting strategies, making marketing efforts more efficient and effective.
Example of Lead Segmentation with ML
Lead Attribute | ML Model Outcome | Segmentation Group |
---|---|---|
Previous Purchase Behavior | Likely to Buy Premium Products | High-Value Prospects |
Email Open Rate | Engaged, Interested in Offers | Active Engagers |
Social Media Interactions | Potential Brand Advocates | Influencers |
Automating Lead Engagement with AI-Powered Chatbots and Email Sequences
AI-driven tools are transforming how businesses engage with leads, moving beyond initial contact to nurture long-term relationships. By integrating AI chatbots and email sequences, companies can ensure that leads are continuously engaged with relevant content, timely responses, and personalized experiences. This process helps maintain interest while guiding potential customers through the sales funnel without requiring constant human intervention.
Automation tools, particularly chatbots and email campaigns, are becoming integral for lead nurturing. AI chatbots can provide instant support, answer questions, and offer product recommendations in real-time. Meanwhile, email sequences, powered by AI, can be tailored to a lead’s behavior and interaction history, allowing businesses to deliver highly personalized content over a longer period, improving the chances of conversion.
AI Chatbots for Immediate Engagement
- Instant Response: AI chatbots are available 24/7 to interact with leads, reducing wait times and providing real-time assistance.
- Personalized Conversations: Based on previous interactions, chatbots can suggest relevant products, services, or content.
- Data Collection: Chatbots can gather valuable data on leads, such as preferences, pain points, and purchase intent, helping to refine marketing strategies.
Email Campaign Automation for Nurturing Leads
- Behavior-Based Segmentation: AI tools analyze customer interactions and segment leads based on behavior to send targeted emails.
- Drip Campaigns: AI-powered email sequences send automated, personalized messages over time, helping to build trust and move leads further down the funnel.
- Content Personalization: Emails are dynamically adjusted based on the lead’s activity, ensuring that they receive the most relevant content at the right time.
Key Benefits
Benefit | Impact |
---|---|
Increased Engagement | AI systems ensure timely and relevant communication, maintaining interest and improving lead retention. |
Personalization | AI tools deliver tailored content, fostering deeper relationships and improving conversion rates. |
Efficiency | Automation of repetitive tasks frees up human resources, allowing teams to focus on strategic decisions. |
By combining AI chatbots with email campaigns, businesses can provide a seamless, personalized experience that nurtures leads effectively, increases conversion potential, and reduces manual effort.
AI-Driven Personalization: Tailoring Content to Individual Leads
In today’s competitive landscape, businesses are increasingly turning to AI tools to enhance lead generation efforts. By leveraging machine learning algorithms, companies can personalize their content in real-time, addressing each lead’s unique needs, preferences, and behaviors. This approach not only improves engagement but also increases conversion rates, as prospects feel that the message speaks directly to them.
AI-driven personalization works by analyzing large sets of data to create detailed profiles of potential customers. These profiles are then used to serve customized content, whether through email, landing pages, or advertisements, that resonates with the individual. The process allows for a more relevant and timely connection, ultimately improving the quality of leads and shortening the sales cycle.
How AI Enhances Content Personalization
- Behavioral Tracking: AI systems monitor user interactions, such as website visits and clicks, to create a dynamic profile.
- Contextual Relevance: AI assesses not only past behavior but also the current context of the lead, like location or time of day, to serve the most appropriate message.
- Real-time Adjustments: AI can instantly adjust the content based on real-time data, ensuring leads are always seeing the most relevant offers and information.
Benefits of AI-Driven Personalization
- Increased Engagement: Tailored content speaks directly to leads’ needs, making them more likely to interact.
- Higher Conversion Rates: Personalized messaging leads to higher likelihoods of conversion, as prospects feel understood.
- Improved Customer Retention: Delivering relevant content increases customer satisfaction and encourages repeat business.
Example of Personalization in Action
Lead Profile | Personalized Content |
---|---|
Tech Startup CEO | Case studies highlighting ROI for other tech startups, demo videos, and whitepapers on scaling businesses using AI tools. |
Marketing Director in Retail | Discount offers, new product recommendations, and insights on enhancing customer experience through data-driven strategies. |
"Personalization is not just about addressing a lead by name; it's about delivering the right message at the right time, based on the right data."
Integrating AI-Powered Lead Generation with CRM Systems
AI-driven tools have revolutionized lead generation, offering businesses the ability to identify, qualify, and nurture leads more effectively. By incorporating these tools with customer relationship management (CRM) systems, companies can achieve seamless workflows, enhanced data accuracy, and better-targeted outreach strategies. The integration allows AI to continuously monitor interactions, predict customer behavior, and automate repetitive tasks, freeing up time for more personalized engagements.
Integrating these advanced technologies into CRM platforms not only streamlines data collection but also improves sales cycle efficiency. By syncing AI-generated insights directly with CRM systems, businesses can ensure that their sales teams work with the most up-to-date information, leading to more informed decision-making and stronger customer relationships. Below are some key benefits of this integration:
- Improved Data Accuracy: AI tools automatically update and refine customer data in the CRM, reducing human errors.
- Streamlined Lead Scoring: AI algorithms assess lead quality and assign scores, ensuring sales teams focus on high-potential prospects.
- Enhanced Personalization: By analyzing past interactions and behaviors, AI helps tailor communication strategies for each lead.
"Integrating AI-driven tools with CRM systems allows businesses to merge predictive analytics with customer insights, driving sales efficiency and enhancing customer retention."
Effective integration relies on several technical and strategic elements. Key steps include:
- Data Synchronization: Ensuring AI tools and CRM systems share data in real time, enabling timely actions and decisions.
- Automation of Workflow: AI-powered tools can automate follow-ups and lead nurturing tasks directly through the CRM.
- Customizable Dashboards: CRM platforms can be configured to display AI-generated insights for easy access and actionable decision-making.
Integration Benefit | Impact |
---|---|
AI-Generated Lead Scoring | Better targeting and prioritization of leads |
Predictive Analytics | Proactive sales strategies based on data trends |
Automated Follow-ups | Time savings and consistent engagement with prospects |
How to Track and Measure AI-Generated Leads in Real Time
Tracking and measuring AI-generated leads in real time is crucial for optimizing the performance of automated marketing strategies. With the help of AI, businesses can identify potential leads quickly, but the challenge lies in effectively monitoring and analyzing these leads as they interact with your brand. Real-time tracking enables businesses to understand how AI-generated leads behave, what triggers their engagement, and how to tailor follow-ups based on their actions. Implementing tools that monitor lead activity is essential to ensuring you don’t miss opportunities to convert them into customers.
AI-driven lead generation tools often provide detailed insights into lead behavior, including click-through rates, engagement times, and the channels through which leads come in. By combining these insights with real-time tracking, companies can gauge the quality of leads and adjust strategies instantly. This approach helps identify trends and patterns that enhance the AI algorithms, ensuring that future leads are even more targeted and relevant.
Key Metrics to Monitor for Real-Time Lead Tracking
- Lead Engagement: Track how frequently and for how long leads engage with your content across various platforms.
- Lead Source: Monitor which channels are generating the most qualified leads (e.g., email, social media, organic search).
- Lead Activity: Measure actions like clicking on links, downloading resources, or submitting forms.
- Conversion Rate: Track how many AI-generated leads convert into actual sales or qualified leads.
Tools to Use for Real-Time Lead Measurement
- CRM Software: Modern CRM platforms like Salesforce or HubSpot offer real-time dashboards that provide insights into lead interactions and conversions.
- Marketing Automation Platforms: Tools such as Marketo or ActiveCampaign allow businesses to track and measure lead behavior in real time, including follow-ups and lead scoring.
- AI Analytics Tools: Platforms like Leadspace or Infer use machine learning to enhance lead tracking and real-time analytics.
Tip: Integrating AI-powered lead scoring into your CRM can automate the process of evaluating lead quality in real-time, allowing for immediate adjustments to outreach strategies.
Real-Time Lead Data Analysis Example
Lead Source | Engagement Level | Conversion Rate |
---|---|---|
Email Campaign | High | 12% |
Social Media Ads | Medium | 8% |
Organic Search | Low | 5% |
Reducing Manual Workload: Leveraging AI to Streamline Lead Qualification
Manually qualifying leads is a time-consuming and error-prone task, often leading to inefficient resource allocation and missed opportunities. By utilizing artificial intelligence (AI), businesses can automate much of the lead qualification process, freeing up sales teams to focus on higher-value tasks. AI-driven solutions use advanced algorithms to analyze a vast amount of data quickly and accurately, significantly improving the overall efficiency of lead scoring and prioritization.
AI tools are designed to assess lead behavior, engagement levels, and demographic data, enabling automatic categorization. This allows organizations to focus on leads with the highest potential, reducing the time spent on less promising contacts. As AI technologies continue to evolve, the accuracy and effectiveness of these solutions will only improve, making them an indispensable asset for modern sales teams.
How AI Reduces Manual Effort in Lead Qualification
- Automated Lead Scoring: AI systems analyze customer interactions and assign scores based on predefined criteria, identifying which leads are most likely to convert.
- Behavioral Analysis: AI tools can track user behavior across multiple channels, identifying patterns that predict the likelihood of conversion.
- Data Integration: AI can integrate data from various sources, ensuring that lead qualification is based on a comprehensive view of the lead’s interactions with the business.
Key Benefits of AI in Lead Qualification
- Improved Accuracy: AI reduces human error, ensuring that the leads passed to the sales team are genuinely high-value prospects.
- Faster Decision-Making: Automation speeds up the qualification process, allowing sales teams to engage with the best leads in real-time.
- Resource Optimization: Sales and marketing teams can focus their efforts on qualified leads, improving overall productivity.
AI tools don’t just replace manual tasks; they enhance the lead qualification process, enabling teams to make data-driven decisions that significantly improve conversion rates.
AI Tools in Action
AI Tool | Key Feature | Benefit |
---|---|---|
Lead Scoring Algorithms | Assigns numerical scores to leads based on predefined criteria | Enables automatic prioritization of high-value leads |
Behavioral Tracking | Monitors user interactions across multiple platforms | Improves understanding of lead interests and conversion likelihood |
Data Integration Platforms | Consolidates data from various sources for more accurate qualification | Provides a complete view of each lead, improving qualification accuracy |