Ai Powered Lead Generation Tools
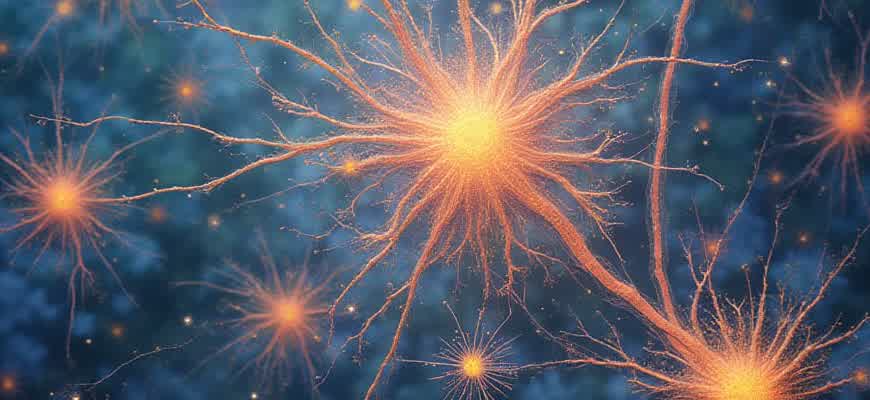
Modern businesses increasingly rely on intelligent systems that automate the identification and qualification of potential customers. These solutions leverage natural language processing, data mining, and behavior prediction to streamline outreach and improve conversion rates.
- Behavioral data analysis to identify high-intent prospects
- Automated segmentation based on industry, role, and activity
- Real-time enrichment from public and proprietary data sources
Note: Machine learning models can detect buying signals across emails, social activity, and CRM updates–reducing manual research time by over 70%.
These tools also provide structured workflows to prioritize outreach and maximize ROI from sales efforts. The automation of lead scoring and pipeline management allows teams to focus on meaningful interactions rather than data entry or filtering.
- Capture website visitors through AI-driven chat interfaces
- Score leads using predictive algorithms based on historical win data
- Trigger personalized campaigns aligned with lead behavior
Feature | Impact |
---|---|
Automated Intent Detection | Identifies high-conversion prospects faster |
Smart CRM Integration | Updates records and reduces manual input |
Intelligent Automation in Lead Identification and Engagement
Modern businesses increasingly rely on advanced software that leverages machine learning to discover, evaluate, and prioritize potential clients. These systems analyze behavior, demographics, and digital footprints to deliver highly relevant prospects, reducing manual effort and human error in the sales funnel.
Unlike traditional tools, these platforms not only collect contact information but also assess user intent and purchasing likelihood through real-time data processing. This allows sales teams to focus only on high-value opportunities and tailor their outreach with precision.
Key Capabilities of Smart Prospecting Solutions
- Automatic profiling based on social activity and website behavior
- Scoring algorithms that predict conversion probability
- Dynamic segmentation of audience lists for personalized campaigns
- Trigger-based messaging sequences initiated by prospect actions
Note: Tools with deep learning capabilities can identify patterns invisible to human analysts, uncovering hidden revenue streams and emerging market niches.
- Input lead sources (e.g., LinkedIn, website visits, email interactions)
- System applies intent analysis and behavioral scoring
- Qualified leads are enriched with firmographic and psychographic data
- Automated outreach campaigns are launched
Feature | Description | Business Impact |
---|---|---|
AI-Driven Scoring | Ranks leads by predicted purchase intent | Reduces time wasted on cold leads |
Behavior Tracking | Monitors interactions across channels | Enhances message timing and relevance |
Content Personalization | Tailors messaging based on user segments | Boosts engagement and response rates |
How to Automate Cold Outreach with AI Lead Generation Tools
Modern business development teams increasingly rely on machine-driven processes to scale their outreach. With the integration of intelligent prospecting platforms, it’s now possible to eliminate manual lead research and personalize outreach at scale. These systems gather, filter, and prioritize contact data based on real-time company insights, job roles, and engagement signals.
Instead of sending generic emails to massive lists, AI-enhanced platforms allow you to craft data-driven messages tailored to each prospect's profile. These tools can analyze digital footprints, identify decision-makers, and initiate outreach sequences without human input, increasing conversion rates and reducing wasted effort.
Steps to Streamline Outreach Using Automated Systems
- Integrate a CRM or contact database with an AI-driven prospecting engine.
- Define filters: target roles, industries, funding rounds, technologies used.
- Use AI to generate personalized email content and sequence templates.
- Set triggers for follow-ups based on recipient behavior (opens, clicks, replies).
- Monitor performance metrics and retrain AI prompts to improve response rates.
Tip: Use intent-based data to prioritize outreach. AI tools can detect purchase signals like recent job postings or new funding rounds.
- Lead scoring models highlight high-probability prospects first.
- Automated A/B testing tools adjust messaging in real time.
- Natural language engines rewrite emails for tone, length, and readability.
Feature | Benefit |
---|---|
Automated Email Sequences | Ensures consistent follow-ups without manual input |
Dynamic Data Enrichment | Keeps prospect info up-to-date for higher accuracy |
Behavior-Based Triggers | Increases engagement by responding to real actions |
Using AI to Score Leads Based on Buyer Intent Signals
Modern B2B and B2C marketing increasingly relies on artificial intelligence to assess how likely a prospect is to make a purchase. Instead of manually evaluating behavior data, AI systems analyze diverse intent signals such as site activity, email interactions, and social media engagement. These insights allow businesses to prioritize high-potential leads with greater precision.
Advanced algorithms detect nuanced behavioral patterns that indicate purchasing interest. For instance, frequent visits to product pages, downloading case studies, or extended dwell times on pricing sections are strong predictors of sales-readiness. AI-driven models assign scores dynamically based on such behaviors, constantly learning from new data to improve accuracy.
Key Intent Triggers Monitored by AI
- Repeated visits to high-value pages (pricing, features, demos)
- Clicks on CTAs within targeted email campaigns
- Engagement with competitor comparison tools
- Search terms used on internal site search engines
- Mentions or comments on branded social content
Insight: Leads that open more than three product-related emails within 72 hours have a 40% higher conversion rate.
Behavior | AI Score Impact | Conversion Correlation |
---|---|---|
Visited Pricing Page | +25 | High |
Downloaded Whitepaper | +15 | Medium |
No Activity for 14 Days | -30 | Low |
- Aggregate multi-channel behavioral data
- Apply predictive scoring algorithms
- Prioritize sales outreach based on ranking
Integrating Intelligent Prospecting Systems with CRM Platforms
Modern customer databases become significantly more powerful when automated prospecting systems are connected to them. Linking these systems allows for real-time enrichment of lead profiles, automated qualification, and seamless handoff between marketing and sales teams. Instead of manually importing contact data or filtering low-quality leads, intelligent engines pre-process and score incoming prospects before they even enter the pipeline.
To enable full synchronization, integration must address both data accuracy and process automation. AI systems extract behavioral signals, firmographics, and engagement metrics, which are then mapped to specific fields inside the CRM. This ensures that account managers always work with the most relevant and updated information, directly aligned with their workflow.
Steps to Connect Intelligent Lead Engines with CRM Platforms
- Define required data points: contact info, company size, intent signals.
- Use APIs or pre-built connectors to establish real-time data flow.
- Map AI-generated lead scores to CRM fields (e.g., "Lead Quality Index").
- Set up automation rules for routing leads to the right sales reps.
Tip: Always validate data mapping with a test group before full deployment to prevent misclassification of high-value opportunities.
- Reduces manual entry by 70% or more
- Accelerates follow-up with auto-assigned lead owners
- Improves forecasting accuracy with AI-enhanced metrics
AI Function | Mapped CRM Field | Benefit |
---|---|---|
Engagement Scoring | Lead Priority | Speeds up response time |
Firmographic Analysis | Account Tier | Targets high-value segments |
Intent Detection | Next Action | Personalizes outreach |
AI-Based Audience Segmentation for Precise Targeting
As a result, outreach strategies become more aligned with real user needs. Campaigns driven by these intelligent segmentation tools show higher open rates, reduced bounce rates, and significantly improved conversion metrics due to the fine-tuned alignment between message and recipient.
Key Benefits of Predictive Segmentation
- Behavior-based targeting: Segments formed from on-site activity, session duration, and frequency of interaction.
- Real-time adjustments: Dynamic audience groups that update as new data flows in.
- Personalization at scale: Tailored content and offers based on segment-specific characteristics.
AI-driven segmentation enables marketing teams to shift from generic campaigns to precision-driven interactions, maximizing every touchpoint's impact.
- Input customer interaction data from CRM, analytics, and ad platforms.
- Train models to detect high-propensity clusters using engagement metrics.
- Export refined audiences to campaign tools for hyper-targeted delivery.
Segmentation Type | Data Used | Use Case |
---|---|---|
Behavioral Clustering | Clickstreams, time on page | Identify high-intent leads |
Predictive Scoring | Historical conversion patterns | Prioritize outreach efforts |
Churn Risk Segmentation | Inactivity, support tickets | Trigger retention campaigns |
Leveraging Natural Language Processing to Qualify Leads
Natural Language Processing (NLP) enables businesses to assess potential clients based on the content and context of their communication. By analyzing conversations, emails, or form submissions, NLP systems can detect intent, urgency, and relevance, helping sales teams focus on high-probability opportunities.
Instead of relying solely on demographic or behavioral data, NLP evaluates textual input to rank prospects. This approach allows for the automatic detection of buying signals, pain points, or budget mentions, all extracted from the lead’s own language.
How Text-Based Insights Improve Lead Prioritization
- Intent Recognition: Identifies phrases indicating purchase readiness (e.g., “we’re looking to switch providers”).
- Urgency Detection: Flags time-sensitive expressions (e.g., “need a solution this month”).
- Fit Analysis: Matches keywords against ideal customer profiles.
NLP-driven qualification reduces time spent on irrelevant leads by up to 40%, enabling faster conversions.
- Integrate NLP tools with CRM to auto-flag qualified leads.
- Train models on existing successful conversations to improve detection accuracy.
- Set thresholds for language patterns that define sales readiness.
Lead Attribute | Example NLP Indicator | Action Triggered |
---|---|---|
High urgency | "Need this ASAP" | Route to senior rep |
Budget readiness | "We’ve allocated funds" | Send pricing deck |
Competitor comparison | "Comparing to X" | Initiate objection handling |
Training AI Models with Historical Lead Data
When building AI models for lead generation, using historical data is crucial to training algorithms to recognize patterns and make accurate predictions. By analyzing past interactions, conversion rates, and demographic information, AI models can better understand the characteristics of high-quality leads. This process involves feeding the AI with a diverse dataset that spans various types of leads and customer behaviors, allowing the system to fine-tune its decision-making capabilities.
Historical lead data typically includes information such as lead source, engagement levels, time-to-conversion, and the specific actions that led to successful outcomes. With this data, machine learning models can identify key factors that differentiate successful leads from less promising ones. The model can then apply these insights to future leads, improving conversion rates over time.
Key Elements of Historical Lead Data
- Lead source: Where the lead originated from (e.g., social media, email campaigns).
- Lead behavior: Actions taken by the lead, such as website visits or email opens.
- Engagement levels: Frequency and intensity of interaction with the business.
- Conversion metrics: What leads ultimately converted into customers, including timing and touchpoints.
Steps in Training AI with Lead Data
- Data Collection: Gather a comprehensive dataset of past leads, including both successful and unsuccessful outcomes.
- Data Preprocessing: Clean and normalize the data to ensure consistency and accuracy in model training.
- Feature Engineering: Extract meaningful features such as lead source, actions taken, and interaction patterns.
- Model Selection and Training: Choose the appropriate machine learning model and train it on the preprocessed data.
- Validation and Testing: Validate the model's performance using a separate test set and adjust parameters as needed.
By leveraging historical lead data, businesses can empower their AI models to predict which leads are most likely to convert, enhancing the overall efficiency of lead generation efforts.
Sample Data for AI Training
Lead ID | Lead Source | Engagement Level | Conversion Status |
---|---|---|---|
001 | Email Campaign | High | Converted |
002 | Social Media | Low | Not Converted |
003 | Referral | Medium | Converted |
Comparing AI Tools for Lead Generation in B2B vs B2C
AI-driven tools for lead generation are becoming increasingly essential in modern marketing strategies, but the approach can differ significantly between B2B (business-to-business) and B2C (business-to-consumer) markets. Each strategy requires tailored solutions that cater to the unique nature of these customer bases. Understanding these differences can help businesses choose the right AI tools to improve their lead generation efforts, maximize ROI, and streamline sales processes.
When comparing AI lead generation tools for B2B and B2C, it's important to recognize how customer profiles, purchasing behavior, and sales cycles influence the features of these platforms. In B2B, the focus is often on targeting a smaller, more specific audience with a longer decision-making process, while B2C strategies typically aim for a larger, more diverse audience with quicker purchasing decisions. Below is a comparison of key elements in AI tools for each approach:
B2B vs B2C Lead Generation AI Tools: Key Differences
Feature | B2B | B2C |
---|---|---|
Target Audience | Specific decision-makers within companies | Broad consumer segments |
Sales Cycle | Longer and more complex | Shorter and impulse-driven |
Lead Qualification | Requires detailed company data, financials, and specific needs | Focuses on consumer preferences and behaviors |
AI Features | Advanced analytics, account-based targeting, CRM integration | Personalization, behavioral tracking, recommendation engines |
Key Factors to Consider in AI Lead Tools for B2B
- Advanced Analytics: AI tools for B2B use large datasets to identify high-value prospects by analyzing company size, industry, and revenue.
- Account-Based Marketing: Targeting specific accounts with tailored outreach strategies based on the company's profile.
- Lead Scoring: Evaluating the likelihood of converting leads through a combination of behavior and company-level data.
Key Factors to Consider in AI Lead Tools for B2C
- Personalization: AI-driven tools use customer data to create personalized recommendations, offers, and content.
- Behavioral Tracking: Monitoring consumer behavior across channels to optimize lead nurturing strategies.
- Automation: AI tools in B2C environments automate responses and follow-ups, increasing efficiency for handling large volumes of leads.
Important Note: While B2B lead generation focuses more on data analysis and long-term relationship building, B2C tools leverage consumer data to enhance immediate engagement and conversion rates.
Reducing Manual Data Entry with AI Lead Capture
Manual data entry is often a time-consuming and error-prone task, especially when dealing with large volumes of lead information. AI-driven lead capture tools offer a significant improvement by automating the process, reducing human error and freeing up valuable resources for more strategic tasks. These tools can extract, validate, and organize contact details from various sources, allowing businesses to focus on nurturing leads rather than collecting data.
By implementing AI in lead capture, companies can streamline their sales funnels and enhance the accuracy of their lead databases. This is particularly beneficial for industries where timely follow-ups and accurate customer information are crucial. AI tools can seamlessly integrate with CRM systems, ensuring that the data is immediately available for sales and marketing teams to act upon.
How AI Enhances Lead Capture Automation
- Real-time Data Extraction: AI tools can automatically capture lead information from forms, emails, social media profiles, and websites.
- Data Validation: AI verifies the accuracy of contact details by cross-referencing them with public databases, reducing errors and duplicates.
- CRM Integration: AI can sync captured data directly with CRM systems, ensuring that the sales team has instant access to fresh leads.
"AI-powered lead capture systems not only reduce manual work but also enhance the quality of the data being collected, leading to more successful sales conversions."
Key Benefits of AI in Lead Capture
Benefit | Description |
---|---|
Time Savings | Automates data entry, reducing the time spent on manual tasks and increasing efficiency. |
Improved Accuracy | AI tools can reduce human errors by automatically validating and correcting lead data. |
Scalability | AI systems can handle large volumes of lead data, making them suitable for businesses of all sizes. |
- Increased Productivity: Teams can spend less time on data entry and more on converting leads into customers.
- Enhanced Customer Insights: AI systems can analyze lead data to provide actionable insights for better-targeted marketing campaigns.