Analytics and Ai-driven Enterprises Thrive in the Age of With
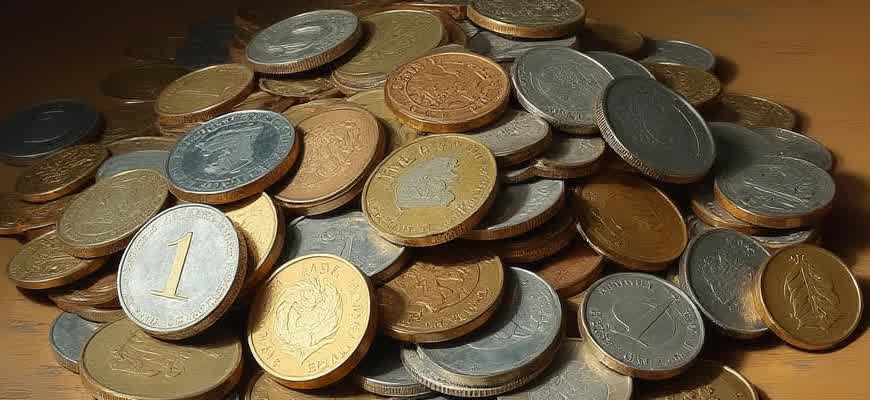
As businesses navigate an increasingly interconnected world, the integration of analytics and AI-driven technologies has become essential for sustainable growth. These tools allow companies to enhance decision-making processes, adapt to rapid changes, and deliver more personalized experiences for customers.
Key drivers of success in the modern enterprise include:
- Real-time data analysis for immediate decision-making
- AI-powered automation to streamline operations
- Advanced predictive capabilities to anticipate market shifts
"Companies that successfully leverage data and artificial intelligence can create a synergy between operational efficiency and customer engagement, driving long-term value."
Understanding the synergy of data and AI within businesses:
- Data Analytics: Provides actionable insights by processing large volumes of structured and unstructured data.
- AI Integration: Enhances business models by offering intelligent automation and machine learning to identify patterns and improve outcomes.
Technology | Benefit |
---|---|
Data Analytics | Improves decision-making and enables predictive analytics for faster responses to market conditions. |
AI Automation | Reduces manual effort, increases efficiency, and ensures consistency across business operations. |
How AI-Driven Analytics Improve Business Decision Making
In today’s competitive landscape, businesses are increasingly relying on AI-driven analytics to gain valuable insights and make data-driven decisions. These advanced technologies enable organizations to process massive amounts of data in real-time, providing decision-makers with accurate forecasts, trends, and actionable recommendations. With AI-powered tools, businesses can not only automate data analysis but also uncover patterns and correlations that would be difficult or impossible to detect manually.
AI-enhanced analytics empower companies to optimize their operations, enhance customer experiences, and identify new growth opportunities. By leveraging machine learning and predictive analytics, businesses can refine their strategies, reduce operational costs, and improve overall performance. These tools allow for faster, more precise decision-making, which is crucial in a fast-paced, data-heavy environment.
Key Benefits of AI-Driven Analytics
- Real-Time Insights: AI systems analyze data instantly, providing decision-makers with up-to-the-minute information.
- Improved Accuracy: AI algorithms can identify trends and anomalies with high precision, reducing human error.
- Cost Efficiency: Automating data analysis reduces the need for manual labor, freeing up resources for other critical tasks.
- Enhanced Forecasting: AI models can predict future outcomes with greater accuracy, helping businesses plan ahead.
How AI-Powered Analytics Help in Decision Making
- Optimizing Marketing Strategies: AI can analyze customer behavior and market trends, allowing businesses to tailor their marketing campaigns for maximum impact.
- Improving Operational Efficiency: AI identifies inefficiencies within processes and suggests actionable improvements to reduce waste and optimize resource use.
- Enhancing Customer Experience: By leveraging AI insights, companies can personalize interactions and offer better services, leading to improved customer loyalty.
- Identifying Market Opportunities: AI analytics can spot emerging trends and new markets, enabling businesses to stay ahead of competitors.
"AI-powered analytics transforms raw data into strategic decisions, helping businesses not only keep pace with change but lead it."
Example of AI in Action
Business Function | AI Application | Outcome |
---|---|---|
Sales | Predictive analytics to identify high-value leads | Higher conversion rates and better targeting of sales efforts |
Operations | AI-driven process optimization | Reduced costs and improved resource allocation |
Customer Support | AI chatbots to provide instant assistance | Improved customer satisfaction and reduced response time |
Automating Routine Data Tasks with AI Tools
In the modern business landscape, data is an essential asset. However, the continuous growth of data has made manual data processing increasingly inefficient and error-prone. By leveraging artificial intelligence (AI), companies can automate repetitive tasks, significantly reducing the time and effort required to manage vast datasets. AI-based solutions excel at handling tasks such as data extraction, transformation, and loading (ETL), allowing businesses to focus on strategic decision-making rather than mundane processes.
The integration of AI-driven tools enables organizations to streamline their workflows and gain deeper insights into their operations. By automating routine data tasks, businesses not only improve efficiency but also enhance data accuracy and consistency. AI can be applied to various stages of data processing, such as data cleaning, classification, and report generation, leading to a more agile and data-driven environment.
Key Benefits of AI Automation
- Increased Efficiency: Automation eliminates the need for manual data handling, speeding up processes and reducing human errors.
- Cost Reduction: AI tools help reduce labor costs associated with manual data entry and processing.
- Improved Data Quality: AI models can detect anomalies, inconsistencies, and errors in data, ensuring high-quality datasets.
- Real-time Insights: AI tools can process data in real-time, allowing businesses to make informed decisions quickly.
Common AI Tools for Data Processing
- Machine Learning Algorithms: These can automatically detect patterns in data, classify it, and even predict future trends.
- Natural Language Processing (NLP): Used for processing and interpreting unstructured text data, such as customer feedback and support tickets.
- Robotic Process Automation (RPA): Automates repetitive tasks such as data entry, validation, and report generation.
- Data Integration Platforms: These AI-powered platforms connect disparate data sources and automate the ETL process.
"By leveraging AI for routine data tasks, businesses can unlock the true potential of their data, turning it from a burden into a strategic asset."
Comparison of AI Tools for Data Processing
Tool | Function | Benefits |
---|---|---|
Machine Learning | Pattern recognition, classification, prediction | Improved decision-making, trend forecasting |
NLP | Text data analysis, sentiment analysis | Insights from unstructured data, improved customer experience |
RPA | Automating repetitive tasks | Cost savings, efficiency improvement |
Data Integration Platforms | Connecting and automating ETL processes | Faster data processing, fewer errors |
Maximizing Revenue through Predictive Analytics and AI Insights
In today’s highly competitive business environment, organizations are increasingly turning to advanced data-driven solutions to drive revenue growth. Predictive analytics and AI-powered insights are transforming how companies approach decision-making, customer engagement, and market trends. These tools enable businesses to anticipate future demands, optimize resource allocation, and enhance overall efficiency, ultimately leading to increased profitability.
By leveraging machine learning algorithms and big data, companies can gain a deeper understanding of customer behavior, identify new revenue opportunities, and streamline operations. The ability to predict customer preferences, forecast sales trends, and optimize pricing strategies provides businesses with a significant advantage over competitors. Below are some key methods to effectively harness these technologies for revenue maximization.
Key Approaches for Revenue Optimization
- Customer Segmentation: AI helps identify distinct customer segments based on past behaviors, enabling targeted marketing efforts and personalized offerings.
- Demand Forecasting: Predictive models help forecast future demand trends, allowing businesses to adjust inventory and production levels accordingly.
- Dynamic Pricing: AI-driven pricing strategies ensure that businesses can adjust their prices based on real-time market conditions, maximizing profit margins.
- Churn Prediction: By analyzing customer data, AI tools can predict when customers are likely to leave, enabling proactive retention strategies.
"Businesses that use predictive analytics to forecast customer needs and behavior are better positioned to make data-driven decisions that directly impact revenue growth."
Examples of AI in Revenue Maximization
- Retail companies use AI to personalize product recommendations and improve customer retention rates.
- Financial institutions leverage AI to offer personalized financial products, increasing cross-sell and up-sell opportunities.
- Manufacturers use predictive maintenance models to reduce downtime, optimize production efficiency, and lower operational costs.
AI Impact on Sales Forecasting
Method | Benefit |
---|---|
Linear Regression Models | Improved accuracy in predicting sales trends based on historical data. |
Time Series Forecasting | Identifies seasonal demand patterns, enabling better inventory management. |
Neural Networks | Detects complex relationships in data, offering highly accurate predictions in dynamic markets. |
Reducing Operational Costs through AI-Driven Workflow Optimization
Enterprises are increasingly leveraging artificial intelligence to streamline business operations and reduce overhead costs. AI-driven tools help businesses optimize workflows by automating repetitive tasks, predicting resource requirements, and providing data-driven insights for process improvements. This leads to a significant reduction in labor and operational expenses, allowing businesses to allocate resources more efficiently and focus on higher-value activities.
Incorporating AI into workflow optimization involves the use of advanced algorithms to analyze data, identify bottlenecks, and automate decision-making. These systems can be implemented in various aspects of business operations, from inventory management to customer service, helping companies to minimize waste, enhance productivity, and ultimately drive profitability.
Key Benefits of AI-Driven Workflow Optimization
- Improved Efficiency: AI tools can automate mundane tasks, reducing human error and speeding up operations.
- Cost Savings: By minimizing resource waste and improving workforce management, companies can lower operational expenses.
- Data-Driven Decision Making: AI analyzes historical data to predict trends and suggest optimal strategies, reducing the need for costly trial-and-error approaches.
“AI enhances workflow efficiency by enabling companies to operate with fewer resources while maintaining high performance and accuracy.”
Examples of AI-Driven Workflow Optimization
- Inventory Management: AI can predict inventory levels and order schedules, reducing stockouts and excess inventory, which saves on storage and logistical costs.
- Customer Support: Chatbots and AI-powered systems can handle routine customer inquiries, freeing up human agents for more complex tasks.
- Predictive Maintenance: AI can monitor machinery and equipment, predicting maintenance needs before failures occur, reducing downtime and repair costs.
AI-Driven Cost Reduction in Numbers
AI Application | Cost Reduction (%) |
---|---|
Inventory Management | 20-30% |
Customer Service Automation | 15-25% |
Predictive Maintenance | 10-20% |
Leveraging Real-Time Analytics to Adapt to Market Changes
In today’s fast-paced business environment, companies must adapt quickly to changing market conditions. Real-time data analysis allows organizations to detect shifts in consumer behavior, economic trends, and competitive dynamics almost instantly. By integrating advanced analytics platforms, enterprises can convert massive data streams into actionable insights, allowing them to respond before potential issues escalate or opportunities pass by.
Companies that use real-time analytics are better positioned to refine strategies on-the-fly, improving decision-making and enhancing agility. Such capabilities are invaluable in sectors like retail, finance, and technology, where delays in responding to market changes can lead to lost revenue or diminished customer trust.
Key Strategies for Adapting to Market Shifts with Real-Time Analytics
- Monitoring Customer Behavior: Real-time analysis of customer interactions, whether online or in-store, allows businesses to adjust marketing campaigns, personalize offers, and provide timely customer support.
- Supply Chain Optimization: Monitoring inventory levels and supplier performance in real-time can help businesses avoid disruptions and maintain product availability.
- Competitive Intelligence: Tracking competitor actions and market sentiment provides organizations with a direct line to necessary adjustments in strategy.
Benefits of Real-Time Analytics in Market Adaptation
- Faster Decision Making: Organizations can make decisions quickly based on live data, minimizing the risk of missed opportunities or slow responses.
- Enhanced Customer Experience: Immediate insights allow businesses to anticipate customer needs and address concerns before they escalate.
- Improved Forecasting: Real-time data enables more accurate forecasting by identifying patterns and trends as they emerge.
Companies that implement real-time analytics not only streamline their operations but also enhance their ability to pivot strategies, ensuring they stay competitive even in volatile markets.
Example of Real-Time Data Utilization
Market Change | Action Taken | Outcome |
---|---|---|
Fluctuating Demand for Product X | Real-time inventory and sales data analysis led to a dynamic pricing adjustment | Increased sales and reduced stockouts |
Competitor Price Cut | Instant monitoring of competitor prices allowed for quick promotional offers | Maintained market share and customer loyalty |
Building Scalable AI Infrastructure for Long-Term Business Growth
To achieve sustainable growth in today's data-driven environment, businesses must create AI infrastructure that is not only robust but also scalable. A scalable AI framework ensures that as a company expands, its artificial intelligence capabilities evolve accordingly. This infrastructure should be designed to handle increasing data volumes and computational needs while maintaining operational efficiency. Leveraging the right tools and technologies is crucial for staying competitive in industries where AI adoption is accelerating rapidly.
Building a scalable AI infrastructure begins with a deep understanding of the company's long-term goals. A well-planned strategy enables organizations to avoid bottlenecks, reduce costs, and optimize AI model performance over time. By investing in scalable cloud solutions, automation, and flexible data pipelines, businesses can ensure that AI initiatives will support long-term success rather than just short-term gains.
Key Elements of Scalable AI Infrastructure
- Data Management: Efficient data storage, retrieval, and preprocessing systems are the backbone of any AI infrastructure. These systems must be able to scale in line with growing datasets and complexity.
- Computational Resources: Cloud computing or hybrid solutions provide the elasticity needed to scale AI workloads, ensuring businesses can access the processing power required at any given time.
- Automation: Automating data pipelines, model training, and deployment allows companies to accelerate AI model iterations and reduces manual intervention.
“A scalable AI infrastructure must prioritize both flexibility and efficiency to accommodate future growth while ensuring optimal performance.”
Steps to Build Scalable AI Infrastructure
- Assess Current Capabilities: Evaluate existing technology stacks, data management systems, and computational resources to identify any limitations that may hinder scalability.
- Adopt Cloud-Based Solutions: Implement cloud platforms like AWS, Azure, or Google Cloud to provide flexible, scalable resources that can grow with the business.
- Invest in Robust Data Pipelines: Develop data pipelines that can efficiently handle both structured and unstructured data while ensuring quick data access for AI models.
- Optimize Machine Learning Models: Ensure that AI models are optimized for scalability, considering factors such as model complexity, deployment frequency, and real-time inference requirements.
Example of Scalable AI Infrastructure Components
Component | Description |
---|---|
Data Storage | Cloud-based storage solutions that allow businesses to scale their data storage as needed. |
Compute Power | On-demand computing resources like GPUs and TPUs for training large AI models efficiently. |
Model Deployment | Continuous integration/continuous delivery (CI/CD) pipelines to streamline AI model deployment at scale. |
Addressing Privacy and Security Challenges in AI-Enabled Analytics
As organizations increasingly leverage AI-powered analytics for decision-making, the challenges surrounding data privacy and security become more pronounced. The integration of large-scale data collection, machine learning models, and predictive analytics introduces risks that can compromise sensitive information. These risks demand comprehensive strategies to protect both the data and the infrastructure supporting AI-driven systems.
To mitigate these risks, businesses must adopt robust privacy policies, implement cutting-edge security protocols, and ensure compliance with evolving regulations such as GDPR or CCPA. This requires a multifaceted approach that spans from data encryption to transparency in AI model development and deployment.
Key Privacy and Security Measures in AI-Driven Analytics
- Data Anonymization: Ensuring that sensitive information is anonymized before being used in AI models to prevent identification of individuals.
- Model Transparency: Maintaining transparency in AI model processes to make it clear how data is processed, analyzed, and used.
- Access Control: Implementing strict user authentication and role-based access to sensitive data to limit exposure.
- End-to-End Encryption: Protecting data both in transit and at rest through encryption techniques to prevent unauthorized access.
"Data security and privacy concerns are not just technical but also ethical issues that companies must address to build trust with their customers."
Security Best Practices for AI Analytics Systems
- Conduct regular security audits of AI infrastructure to identify vulnerabilities.
- Incorporate advanced threat detection systems to monitor potential cyber-attacks.
- Develop incident response plans to address breaches swiftly and efficiently.
- Ensure all AI models undergo rigorous testing for robustness and resistance to adversarial attacks.
Compliance with Regulations
Regulatory frameworks play a crucial role in shaping how organizations manage privacy and security risks in AI analytics. Compliance with regulations such as the General Data Protection Regulation (GDPR) and the California Consumer Privacy Act (CCPA) requires businesses to adopt stringent practices that prioritize user consent, data transparency, and user rights.
Regulation | Key Requirements |
---|---|
GDPR | Requires explicit consent, data minimization, and the right to be forgotten. |
CCPA | Gives consumers the right to know, delete, and opt-out of the sale of personal data. |