Customer Segmentation Best Practices
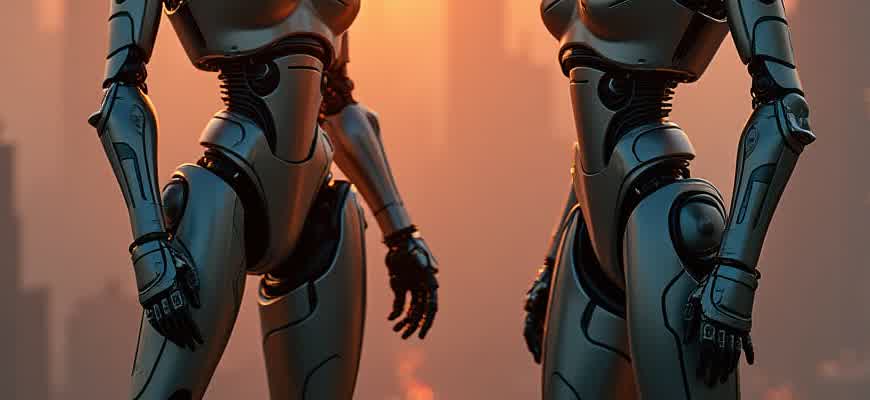
Understanding customer segmentation is key to tailoring marketing efforts and optimizing customer experiences. A well-structured approach allows businesses to identify distinct customer groups, which can then be targeted with personalized strategies. Below are the best practices that can help organizations achieve accurate segmentation and maximize the impact of their marketing campaigns.
1. Define Clear Segmentation Criteria
Before segmenting your customers, it’s important to set clear parameters based on relevant data. Use a mix of the following factors to create actionable segments:
- Demographic Information: Age, gender, income, education level, etc.
- Geographic Data: Location, urban vs. rural, region-specific behaviors.
- Behavioral Patterns: Purchase history, product usage frequency, online engagement.
- Psychographics: Interests, lifestyle, values, personality traits.
2. Leverage Data Analysis Tools
Effective segmentation is often driven by robust data analysis. Tools that can help in identifying meaningful patterns include:
- Data Mining: Helps uncover hidden customer insights from large datasets.
- Predictive Analytics: Uses historical data to forecast future behaviors and trends.
- Customer Surveys: Provides direct input from customers regarding preferences and needs.
"Accurate segmentation can increase marketing efficiency by 20-30% and enhance customer retention rates." – Market Research Insights
3. Monitor and Refine Segmentation Regularly
As customer preferences and behaviors evolve, it’s important to revisit your segmentation strategy periodically. Segmentations that were relevant a year ago may no longer be as effective, requiring regular analysis and adjustments.
Segmentation Type | Example | Use Case |
---|---|---|
Demographic | Age, gender | Targeting based on life stage, such as products for young professionals or retirees. |
Behavioral | Purchase frequency, brand loyalty | Customizing offers for frequent buyers or promoting new products to occasional customers. |
Psychographic | Lifestyle, values | Creating personalized experiences for customers who prioritize sustainability or innovation. |
How to Identify and Prioritize Key Segmentation Variables
Effective customer segmentation starts with identifying the most relevant variables that distinguish different customer groups. These variables should provide insights into customer behavior, needs, and preferences that will drive targeted marketing and product strategies. A well-defined set of variables enables businesses to create personalized experiences and allocate resources efficiently. Prioritizing the right variables is critical to ensuring that segmentation efforts have the desired impact on business outcomes.
Once you have gathered data, it’s important to assess which variables will deliver the most value to your segmentation strategy. Not all variables will be equally useful, and some may offer more actionable insights than others. By evaluating variables based on their impact on customer behavior, business goals, and the ease of implementation, you can focus your efforts on those that will truly influence your marketing and sales outcomes.
Steps to Identify and Rank Key Variables
- Analyze Available Data: Collect data from various sources, including sales, customer interactions, and surveys, to understand customer behavior.
- Map to Business Goals: Ensure the variables align with your objectives, such as increasing sales, enhancing customer retention, or improving customer satisfaction.
- Evaluate Actionability: Prioritize variables that are measurable and can be effectively used in marketing campaigns or product development.
- Test and Refine: Continuously test the selected variables and refine them based on feedback and new data.
Key Variables to Consider
- Customer Behavior: This includes purchase frequency, product preferences, and online activity. It helps identify high-value customers and tailor promotions.
- Demographics: Age, gender, income level, and education can offer insights into broad customer profiles but may not be as dynamic as behavioral data.
- Geographic Location: Understanding where customers are located can help target local promotions or optimize logistics and distribution.
- Customer Value: Lifetime value, purchasing power, and potential for upselling or cross-selling help determine which segments to focus on for revenue growth.
Important: Behavioral data, such as engagement and purchasing history, typically offers more precise insights than demographic data alone, allowing for more targeted marketing efforts.
Example of Prioritizing Segmentation Variables
Variable | Impact on Business | Priority |
---|---|---|
Purchase Frequency | Indicates customer loyalty and potential for repeat business. | High |
Product Preferences | Helps create personalized offers and marketing campaigns. | High |
Demographics | Useful for targeting broad customer segments but less impactful than behavior. | Medium |
Geographic Location | Important for localized promotions, but not as central for global strategies. | Low |
Choosing the Right Data Sources for Accurate Customer Profiles
When creating accurate customer profiles, selecting the most relevant and reliable data sources is critical for obtaining a complete understanding of your customer base. These data sources provide essential insights that allow businesses to segment their customers effectively, target them with personalized messaging, and optimize their marketing strategies. The goal is to utilize data that reflects customers' behaviors, preferences, and purchasing patterns, so you can build actionable and effective profiles.
It’s important to differentiate between primary and secondary data sources. Primary sources provide direct insights from customers, while secondary sources include data collected from external sources, such as market research reports or third-party analytics. Combining both types can enhance the quality and accuracy of the customer segmentation process. Below are some of the key data sources for building detailed customer profiles:
Key Data Sources for Customer Segmentation
- Transactional Data: Purchase history, frequency, and average order value.
- Demographic Data: Age, gender, income, education, and location.
- Behavioral Data: Website interactions, click-through rates, and user journey analysis.
- Psychographic Data: Customer interests, lifestyle choices, and values.
- Social Media Insights: Engagement patterns, sentiment analysis, and online conversations.
Choosing the right data sources is essential for accurate customer segmentation, as inaccurate or incomplete data can lead to poor targeting decisions and wasted marketing efforts.
How to Evaluate Data Sources
- Relevance: Ensure the data aligns with the goals of your segmentation strategy.
- Accuracy: Verify the data's correctness to avoid building misleading customer profiles.
- Timeliness: Use up-to-date data to reflect current customer preferences and behaviors.
- Completeness: Gather as much data as possible to build comprehensive customer profiles.
- Integration: Make sure the data can be easily integrated into your CRM or analytics platforms.
Example of Effective Data Integration
Data Source | Type | Benefit |
---|---|---|
CRM Data | Primary | Provides in-depth customer profiles based on purchase history and interactions. |
Website Analytics | Secondary | Helps track behavior patterns, engagement, and conversion metrics. |
Social Media Insights | Secondary | Offers sentiment analysis and trends based on social interactions. |
Building and Maintaining Segments Based on Behavioral Insights
Effective customer segmentation requires a deep understanding of how users interact with a brand. Behavioral insights provide a clear picture of customer actions, such as browsing patterns, purchase history, and engagement frequency. By leveraging this data, businesses can form more accurate and dynamic customer groups that reflect actual behaviors rather than just demographics.
Building and maintaining these segments involves continuous monitoring and refinement. Behavioral data changes over time, so segmentation must be an ongoing process to ensure the groups remain relevant and actionable. This approach also allows businesses to adapt to shifts in consumer behavior and preferences, offering a personalized experience at every touchpoint.
Key Practices for Segmenting Customers Based on Their Behavior
- Track Actionable Metrics: Identify and monitor customer actions that directly influence business outcomes, such as clicks, purchases, or form submissions.
- Use Behavioral Triggers: Segment customers based on specific behaviors, such as cart abandonment, frequent browsing, or high engagement with promotions.
- Analyze Engagement Levels: Group customers by their interaction frequency and intensity with the brand–whether they are occasional visitors or highly engaged users.
- Monitor Changes Over Time: Regularly update segments as user behavior evolves to maintain relevance and drive better-targeted marketing efforts.
Steps to Build Dynamic Behavioral Segments
- Data Collection: Gather data from multiple touchpoints, including web analytics, transaction logs, and customer interactions.
- Define Segmentation Criteria: Choose the most relevant behavioral attributes to form distinct customer segments.
- Apply Machine Learning Algorithms: Use predictive analytics and clustering techniques to identify patterns and predict future behaviors.
- Refinement and Validation: Continuously test and refine the segments to ensure accuracy, using A/B testing and feedback loops.
Example of Behavioral Segmentation Criteria
Segment | Criteria | Behavioral Characteristics |
---|---|---|
Frequent Shoppers | Multiple purchases in the past 6 months | High transaction volume and repeat purchases |
Engaged Visitors | Regular site visits but no purchases | Frequent page views, clicks, but abandoned checkout |
One-Time Buyers | Single purchase within the last year | Low interaction after purchase, rarely return |
"Behavioral insights help companies focus their efforts on the most promising customer groups, leading to higher conversion rates and more efficient marketing strategies."
Leveraging Demographics for Targeted Marketing Campaigns
Effective use of demographic data allows businesses to tailor marketing campaigns to specific audience segments, increasing the relevance and impact of their messaging. By analyzing characteristics such as age, gender, income, and location, brands can deliver personalized experiences that resonate with distinct consumer groups. Demographic segmentation helps businesses optimize advertising spend and ensure they reach the right customers with the right message at the right time.
Incorporating demographic insights into marketing strategies can enhance customer engagement and improve conversion rates. Rather than broadcasting a generic message to a wide audience, brands can create targeted campaigns that appeal directly to the needs, desires, and behaviors of specific consumer profiles. This approach not only fosters stronger connections with customers but also drives more efficient and effective marketing efforts.
Key Demographic Factors for Campaign Personalization
- Age: Tailor messages that appeal to the interests and life stages of different age groups.
- Gender: Create gender-specific offers or products that speak to individual preferences and needs.
- Income Level: Design pricing strategies or premium product lines targeted at high-income or budget-conscious consumers.
- Geographic Location: Customize campaigns based on regional preferences, cultural influences, or local trends.
By focusing on the demographics of your target market, you ensure that your message resonates with the right people, at the right time, through the right channels.
Demographic Segmentation Examples
- Luxury Brands: Targeting high-income individuals with exclusive offers, premium experiences, or tailored advertisements.
- Fitness Industry: Marketing fitness products to young adults and targeting seniors with health-focused offerings.
- Travel Agencies: Offering tailored vacation packages based on family, solo traveler, or business traveler needs.
Demographics Breakdown Table
Demographic Factor | Strategy |
---|---|
Age | Create age-appropriate content that speaks to the unique interests of each group. |
Gender | Use gender-specific messaging that aligns with consumer preferences and cultural expectations. |
Income | Design premium or value-driven products that meet the financial expectations of different groups. |
Location | Personalize offers based on regional or cultural differences, enhancing relevance for local consumers. |
Using Psychographic Data to Deepen Customer Understanding
Psychographic information provides a deeper insight into customer behavior, focusing on their values, attitudes, and lifestyle choices. By analyzing these factors, businesses can create more precise customer segments that reflect not only what consumers buy but why they buy it. This deeper understanding helps tailor marketing strategies and product offerings in ways that resonate more effectively with various customer groups.
When applied correctly, psychographic data goes beyond basic demographics to reveal emotional triggers, motivations, and purchasing drivers. It allows brands to connect on a more personal level, ensuring that messaging and engagement strategies feel more relevant and authentic to each segment.
Key Areas to Explore in Psychographic Data
- Values and Beliefs: Understanding core beliefs helps in shaping messages that resonate with customers' worldviews.
- Lifestyle Choices: Analyzing how customers spend their time or money can predict their purchasing behavior.
- Personality Traits: Knowing whether customers are adventurous or conservative helps in designing targeted campaigns.
Effective Techniques for Gathering Psychographic Data
- Surveys and Questionnaires: Direct feedback from customers can uncover values, interests, and opinions.
- Social Media Monitoring: Analyzing online behavior and interactions gives insight into preferences and attitudes.
- Customer Interviews: In-depth conversations can provide a clearer understanding of customers' mindset and motivations.
Example of Psychographic Segmentation
Customer Segment | Values | Lifestyle | Buying Motivation |
---|---|---|---|
Eco-Conscious Consumers | Environmental sustainability | Outdoors, minimalistic living | Support brands with eco-friendly products |
Luxury Seekers | Status and exclusivity | High-end fashion, travel | Desire for premium, unique experiences |
Tech Enthusiasts | Innovation and efficiency | Early adoption of gadgets, online gaming | Need for cutting-edge, functional technology |
Psychographic data allows companies to personalize experiences, creating stronger emotional connections that drive loyalty and advocacy.
Optimizing Segmentation with Predictive Analytics
Incorporating predictive analytics into customer segmentation allows businesses to enhance the precision of their targeting efforts. By leveraging historical data and advanced algorithms, companies can predict future behaviors, preferences, and purchasing patterns. This not only improves the relevance of marketing strategies but also fosters more personalized customer experiences. Predictive models, such as regression analysis or machine learning algorithms, analyze vast datasets to identify key patterns that influence customer decisions.
Using this data-driven approach helps organizations to segment their audience more effectively, enabling them to focus on high-potential groups. Predictive analytics can highlight which customers are most likely to convert, renew, or churn, allowing businesses to tailor their messaging and offerings accordingly. This proactive strategy empowers companies to anticipate customer needs and enhance their engagement strategies.
Key Techniques for Optimizing Segmentation
- Behavioral Segmentation: Analyzing past customer behavior to predict future actions.
- RFM Analysis (Recency, Frequency, Monetary): A method that evaluates how recently, how often, and how much a customer has purchased.
- Customer Lifetime Value (CLV) Prediction: Forecasting the potential value of a customer over their entire relationship with the company.
Steps to Implement Predictive Segmentation
- Data Collection: Gather relevant data points from multiple customer touchpoints.
- Data Cleaning: Remove inconsistencies or irrelevant data to ensure the accuracy of predictions.
- Model Selection: Choose an appropriate algorithm (e.g., decision trees, clustering, neural networks) to build the predictive model.
- Model Training: Train the model using historical data to improve prediction accuracy.
- Implementation: Apply the model to segment customers dynamically and adjust marketing efforts based on the predictions.
Important Insights
"Predictive analytics not only identifies existing customer segments but also reveals emerging trends, enabling companies to stay ahead of the curve."
Example of Predictive Segmentation
Segmentation Criteria | Predicted Action |
---|---|
High RFM Score | Offer loyalty programs to retain high-value customers |
Low RFM Score | Send re-engagement campaigns to reduce churn |
High CLV Potential | Target with premium product recommendations |
Testing and Refining Customer Segments for Ongoing Improvement
Regular evaluation of customer segments is essential for ensuring the accuracy and relevance of the data being used for targeting. Over time, markets and customer behaviors evolve, making it necessary to test segmentations frequently and refine them accordingly. By implementing iterative testing processes, businesses can adjust their marketing strategies to meet the needs of their audience more effectively.
The refinement process involves identifying gaps in segmentation, analyzing customer feedback, and leveraging new data sources. Regular adjustments can lead to better customer experiences, increased engagement, and more effective targeting. This ongoing cycle of testing and refining allows companies to stay aligned with customer expectations and improve business outcomes.
Key Steps for Effective Testing and Refining
- Monitor Performance Metrics: Track KPIs such as conversion rates, customer satisfaction, and lifetime value to gauge the effectiveness of each segment.
- Gather Qualitative Data: Conduct surveys or focus groups to understand customer needs and preferences, helping identify potential adjustments to segments.
- Test Hypotheses: Run A/B tests or small-scale trials to see how changes to customer segmentation affect performance.
- Adjust Based on Findings: Use the insights from testing to refine segments, ensuring they align with evolving customer behaviors.
Tools and Techniques for Segment Testing
Technique | Benefit |
---|---|
A/B Testing | Enables direct comparison of different segment approaches to determine the most effective method. |
Customer Feedback | Provides qualitative insights into customer satisfaction and expectations, highlighting areas for improvement. |
Predictive Analytics | Anticipates future customer behavior by analyzing historical data, allowing for more accurate segmentation adjustments. |
"Testing is not a one-time event; it’s an ongoing process that helps refine strategies and optimize customer experiences."