Ai and Personalization
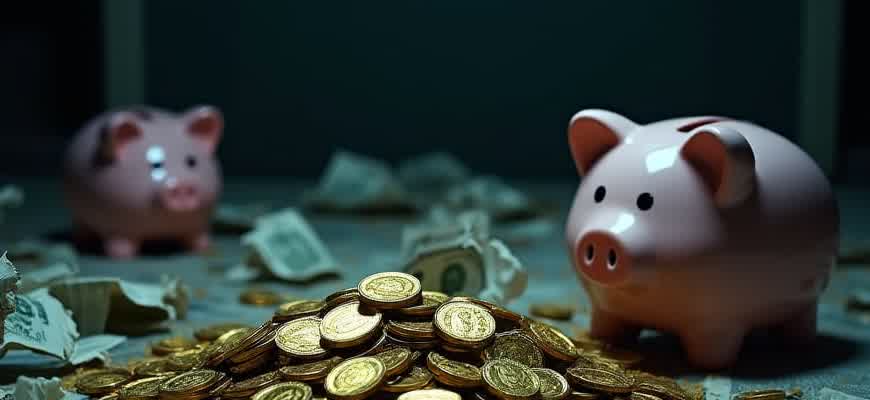
Artificial intelligence (AI) plays a key role in revolutionizing how businesses and services tailor experiences to individuals. With the help of AI, personalization has evolved beyond static recommendations to adaptive, real-time adjustments that enhance user engagement and satisfaction. By analyzing user data, AI systems can predict preferences and customize interactions based on past behavior and context.
Applications of AI in Personalization:
- Retail: AI-driven product recommendations based on browsing and purchasing history.
- Content Platforms: Dynamic content curation based on user interactions and viewing habits.
- Healthcare: Personalized treatment plans using AI to analyze patient history and genetic data.
"The shift from generic services to hyper-personalized experiences is driven by AI's ability to process vast amounts of data and make real-time decisions."
How AI Enhances Personalization:
- Data Collection: AI systems gather user data from various touchpoints, such as websites, apps, and social media platforms.
- Pattern Recognition: AI algorithms detect patterns and correlations that would be impossible for humans to identify manually.
- Real-time Customization: AI continuously adapts to changes in user preferences and behavior, ensuring that the experience remains relevant.
Example of AI-Powered Personalization:
Sector | AI Application | Benefit |
---|---|---|
Retail | Personalized product suggestions | Increased conversion rates and customer loyalty |
Entertainment | Content recommendations based on user history | Improved user engagement and retention |
Healthcare | Personalized treatment options | Better patient outcomes and efficiency in care |
AI and Personalization: A Practical Guide
Personalization has become a critical element for businesses looking to engage with their customers on a deeper level. Leveraging Artificial Intelligence (AI) technologies offers companies the opportunity to tailor experiences, products, and services to the unique preferences of individual users. By using machine learning algorithms and data analytics, businesses can create dynamic and personalized interactions that improve customer satisfaction and drive conversions.
AI can be used to analyze customer behavior, predict future preferences, and automate decision-making processes that were previously manual. This guide explores practical applications of AI in personalizing user experiences and how businesses can implement these technologies effectively to create more meaningful connections with their audience.
Applications of AI for Personalization
AI can be used in a variety of ways to customize user experiences. Here are some of the most common methods:
- Recommendation Systems: AI can analyze user data to suggest relevant products, content, or services, increasing the chances of a successful conversion.
- Dynamic Pricing: By evaluating user behavior and market trends, AI can help adjust prices in real-time to reflect demand and customer willingness to pay.
- Chatbots and Virtual Assistants: AI-driven bots can provide personalized assistance, resolving issues and answering queries based on individual customer history and preferences.
- Email Personalization: AI can craft tailored email campaigns based on user behavior and past interactions, increasing engagement rates.
Steps to Implement AI-Powered Personalization
For businesses looking to integrate AI into their personalization strategy, here is a step-by-step guide:
- Data Collection: Start by gathering accurate and relevant data on customer interactions, preferences, and behaviors.
- Choose the Right AI Tools: Select AI technologies that align with your business needs, such as machine learning algorithms for recommendations or natural language processing (NLP) for chatbots.
- Model Training: Train AI models using the collected data to recognize patterns and predict future behavior.
- Testing and Optimization: Continuously test the AI algorithms and adjust them for improved performance, ensuring the personalization is effective and efficient.
Key Considerations for Effective AI Personalization
Factor | Consideration |
---|---|
Data Privacy | Ensure compliance with data protection regulations, such as GDPR, while collecting and using personal data. |
Customer Trust | Transparency in how data is used can help maintain customer trust and reduce concerns over privacy. |
Scalability | Choose AI solutions that can scale as your business grows and adapt to changing customer needs. |
"AI-powered personalization isn't just about recommending products; it's about understanding and anticipating what customers truly want before they even ask for it."
How AI Enhances Customer Segmentation in Personalization
Customer segmentation plays a vital role in creating personalized experiences for users. With the advancement of AI technologies, businesses now have the ability to segment their customers with greater accuracy and precision. By analyzing vast amounts of data, AI models can uncover patterns and trends that human analysts may overlook, leading to more refined and actionable customer groups.
AI-driven tools employ machine learning algorithms to segment customers based on a variety of behaviors, preferences, and demographic details. This process allows brands to deliver highly relevant content, offers, and recommendations to the right customer at the right time, enhancing the overall user experience.
Key Benefits of AI in Customer Segmentation
- Data-Driven Insights: AI models process large datasets and provide deep insights into customer behavior that would be impossible to identify manually.
- Dynamic Segmentation: Unlike traditional methods, AI allows for real-time segmentation adjustments based on new data, ensuring up-to-date targeting.
- Predictive Analytics: AI can forecast customer actions and preferences, enabling brands to proactively address needs before they arise.
AI-Enhanced Segmentation Techniques
- Clustering: Grouping customers into clusters based on shared attributes, such as buying habits or engagement patterns.
- Behavioral Segmentation: Analyzing user actions, such as purchase history, browsing patterns, and interactions with the brand.
- Predictive Modeling: Using historical data to predict future behaviors and segment customers accordingly.
"AI not only identifies who your customers are but also predicts what they are likely to do next, enabling brands to personalize every touchpoint."
Example of AI in Segmentation: A Comparative Table
Segmentation Method | AI Application | Benefit |
---|---|---|
Traditional Segmentation | Based on basic demographics or geography. | Offers general grouping but lacks depth in behavior analysis. |
AI-Driven Segmentation | Analyzes dynamic behaviors, preferences, and predictive trends. | Highly personalized groups, ensuring tailored experiences and proactive engagement. |
Leveraging Data for Tailored User Experiences with AI
Personalized experiences are becoming a central aspect of modern digital platforms, and artificial intelligence is at the heart of this shift. By utilizing vast amounts of user data, AI systems can generate dynamic, customized interactions that meet the unique needs of each user. The ability to anticipate behaviors, preferences, and actions helps businesses engage with their audiences on a deeper level, creating more relevant and impactful experiences.
Through sophisticated algorithms, AI can analyze individual user data in real-time, adapting the interface, content, and recommendations accordingly. This deep level of personalization is not just about providing more relevant content, but also enhancing user satisfaction, loyalty, and conversion rates. The more accurately AI can predict what a user wants, the better their overall experience will be.
Data-Driven Personalization Strategies
- Behavioral Analysis: AI tracks how users interact with digital platforms, collecting data on their browsing patterns, clicks, and time spent on different sections.
- Contextual Relevance: By understanding the context in which a user is engaging (such as location, device, or time of day), AI can offer more meaningful content.
- Predictive Recommendations: AI anticipates user preferences by analyzing past behavior, making personalized suggestions for products, services, or content.
Key Components of AI-Powered Personalization
- Data Collection: AI systems rely on both structured and unstructured data, including user demographics, online behavior, and historical interactions.
- Machine Learning Models: These models enable the system to detect patterns, learn from new data, and adapt to evolving user needs over time.
- Real-Time Adaptation: AI continuously updates the user experience based on real-time data, ensuring that users always receive the most relevant interactions.
"AI-driven personalization is not just about offering customized content; it’s about building a system that evolves with the user, adapting seamlessly to their preferences."
Example: Personalized Content in E-Commerce
User Activity | AI Response |
---|---|
Recent Purchase: Running Shoes | AI suggests complementary products such as workout apparel and accessories. |
Frequent Browsing of Fitness Equipment | AI recommends new workout gear based on past interest and trending items. |
Abandoned Cart with Items in Sports Category | AI sends personalized follow-up offers or reminders, tailored to the user’s interest in fitness products. |
AI-Driven Content Recommendations: Algorithms at Work
Content recommendation systems have revolutionized the way digital platforms engage with users, providing tailored experiences that align with personal preferences. By leveraging artificial intelligence, these systems analyze vast amounts of user data to predict and deliver content that a user is most likely to find engaging. The algorithms powering these systems employ sophisticated techniques such as collaborative filtering, content-based filtering, and hybrid models to ensure precision in their recommendations.
These AI-driven systems are not only adaptive to user behaviors but also improve over time as they collect more data. The dynamic nature of AI allows the systems to continuously refine their predictions, offering a more personalized experience with each interaction. Below, we explore the core mechanisms behind these algorithms and how they work in real-time to influence content discovery.
Key Mechanisms of AI Content Algorithms
- Collaborative Filtering: This method recommends content based on the behaviors of similar users. For example, if users with similar browsing histories liked a particular video, it will be suggested to you.
- Content-Based Filtering: In this approach, content is recommended based on the attributes of items you've interacted with before, such as keywords, genre, or topic.
- Hybrid Systems: These combine both collaborative and content-based filtering to maximize the accuracy of recommendations.
"By analyzing user behavior and preferences, AI algorithms can make remarkably accurate predictions, creating more engaging and tailored content experiences."
How Algorithms Learn and Adapt
The learning process of AI-driven recommendation systems involves a continuous feedback loop. Algorithms start by analyzing user interactions, such as clicks, views, and time spent on specific content. Over time, the system fine-tunes its predictions based on the accumulated data.
- Data Collection: Every user action is recorded and analyzed.
- Pattern Recognition: Algorithms detect patterns and preferences within the data.
- Recommendation Generation: Based on the recognized patterns, personalized suggestions are provided.
- Feedback Loop: User responses to recommendations are fed back into the system to refine future suggestions.
Types of AI Algorithms in Action
Algorithm Type | Usage | Example |
---|---|---|
Collaborative Filtering | Recommends based on user similarity | Netflix recommending shows based on the preferences of users with similar tastes |
Content-Based Filtering | Recommends based on content features | Spotify suggesting songs from the same genre or artist |
Hybrid Model | Combines both methods for more accurate recommendations | Amazon suggesting products based on both past purchases and user preferences |
Optimizing Product Pricing with AI Personalization Tools
AI-powered personalization tools offer businesses innovative ways to refine product pricing strategies based on customer behavior and preferences. By analyzing vast amounts of data, AI systems can predict how a customer is likely to respond to different price points. These insights allow companies to adjust their pricing dynamically, ensuring they remain competitive while maximizing revenue. The ability to offer personalized prices tailored to individual consumer profiles enhances the customer experience, encouraging more purchases and fostering loyalty.
Moreover, AI-driven pricing models go beyond simple price adjustments. They incorporate factors such as demand elasticity, customer purchase history, and market trends. By continuously learning and adapting, these tools ensure that pricing decisions are informed by real-time data, optimizing profit margins and reducing overpricing or underpricing risks. Below are some of the key advantages of leveraging AI for product pricing optimization.
Key Benefits of AI in Pricing Optimization
- Dynamic Pricing: AI can adjust prices in real-time based on changing market conditions and consumer demand.
- Customer Segmentation: Personalization tools segment customers into groups, tailoring prices based on their unique buying behaviors and preferences.
- Price Sensitivity Analysis: AI models analyze how sensitive different customer segments are to price changes, allowing for more precise pricing strategies.
- Competitor Monitoring: AI can track competitors' pricing, enabling businesses to stay competitive while maintaining profitability.
How AI Personalization Tools Work for Pricing
- Data Collection: AI gathers data from various touchpoints, including previous transactions, browsing behavior, and external factors like seasonality.
- Price Optimization Algorithm: Using machine learning, AI creates predictive models to determine the optimal price for each customer segment.
- Continuous Adjustment: The system continuously monitors the market, consumer behavior, and competitor prices to adjust pricing dynamically.
"AI-powered pricing strategies allow businesses to move away from one-size-fits-all models, instead offering personalized pricing that appeals directly to individual customer preferences."
Example of AI Pricing Model
Customer Segment | Base Price | Personalized Price | Price Sensitivity |
---|---|---|---|
Frequent Shoppers | $100 | $90 | Low |
Price-Sensitive Shoppers | $100 | $80 | High |
Occasional Shoppers | $100 | $95 | Medium |
AI-Driven Real-Time Email Personalization
Artificial intelligence has revolutionized how businesses approach email marketing, allowing for dynamic and highly tailored campaigns. By integrating AI into email systems, companies can offer personalized content based on real-time customer behavior, preferences, and interaction history. This advanced approach ensures that each email resonates with its recipient, enhancing user engagement and increasing conversion rates.
Real-time personalization powered by AI focuses on delivering relevant content when the recipient is most likely to engage. AI systems continuously analyze data such as browsing history, past purchases, and engagement with previous emails to optimize the timing and content of each message. This creates a seamless and customized experience that feels natural to the customer.
How AI Enables Dynamic Email Campaigns
- Real-time Data Integration: AI processes data in real-time, adjusting email content based on user interactions, location, and time of day.
- Behavioral Targeting: AI can segment users by their actions, such as abandoning a cart or clicking on specific links, to tailor email content to those behaviors.
- Adaptive Subject Lines: AI can automatically adjust the subject line for each recipient based on their preferences, increasing open rates.
Benefits of Real-Time Personalization in Email Campaigns:
Benefit | Description |
---|---|
Increased Engagement | Emails are more likely to be opened and interacted with when the content aligns with the recipient's current interests. |
Higher Conversion Rates | Personalized emails that reflect real-time behaviors drive more conversions, whether it's completing a purchase or signing up for an event. |
Enhanced Customer Retention | By delivering relevant content consistently, customers feel valued and are more likely to stay loyal to the brand. |
"Real-time personalization powered by AI helps create a customer experience that feels intuitive, fostering stronger relationships and boosting overall campaign performance."
Building Customized Virtual Assistants for Improved Customer Assistance
In the era of automation, personalized chatbots play a significant role in enhancing the quality of customer service. By leveraging artificial intelligence (AI), these bots are capable of offering tailored interactions that meet the unique needs of each user. With natural language processing (NLP) and machine learning algorithms, these systems can understand customer queries more effectively and provide relevant, context-driven solutions. This approach not only improves response times but also fosters a more engaging and efficient customer experience.
To create truly effective and personalized chatbot systems, several factors need to be considered. These include integrating customer data, employing real-time learning mechanisms, and ensuring that the bot understands and responds appropriately to a diverse range of inquiries. A well-built virtual assistant can adapt to different customer preferences, offering assistance in a way that feels intuitive and human-like, driving customer satisfaction and loyalty.
Key Elements in Building Effective Personalized Chatbots
- Data Integration: Using customer data to personalize responses enhances relevance and accuracy.
- Adaptive Learning: Allowing the bot to improve over time based on user interactions increases efficiency and accuracy.
- Real-time Interaction: AI systems must provide instantaneous responses to ensure smooth communication.
Challenges in Personalizing Virtual Assistants
- Data Privacy: Ensuring that customer information is securely stored and used only with consent.
- Context Understanding: Accurate interpretation of customer queries in diverse contexts remains challenging.
- Maintaining Human-Like Interaction: Balancing AI-driven responses with a natural conversational flow is crucial.
"Personalized chatbots are not just about answering questions; they are about creating a dynamic, tailored experience for each user."
Features of Personalized Chatbots
Feature | Benefit |
---|---|
Natural Language Processing (NLP) | Improves understanding of complex queries, enabling more accurate responses. |
Customer Data Utilization | Allows for personalized recommendations and solutions based on historical interactions. |
24/7 Availability | Ensures constant customer support, increasing convenience and accessibility. |
Leveraging Predictive Analytics for Customized Marketing Strategies
Predictive analytics has become a cornerstone in refining personalized marketing efforts. By analyzing historical data, businesses can predict future customer behaviors, enabling them to craft highly targeted and relevant campaigns. This allows companies to move away from generic marketing tactics and focus on individual preferences, ultimately enhancing customer engagement and driving conversions.
The process of implementing predictive analytics involves integrating sophisticated algorithms and machine learning models that can forecast potential customer actions. These models are trained on vast amounts of data, ranging from customer interactions to browsing patterns, making them effective in anticipating future trends and preferences. The result is the creation of marketing strategies tailored to each customer's specific needs and behaviors.
Key Benefits of Predictive Analytics in Personalized Marketing
- Improved targeting: Marketing efforts are directed towards the most likely customers, increasing the likelihood of engagement and purchase.
- Enhanced customer experience: Personalized content, offers, and recommendations lead to a more satisfying customer journey.
- Efficient resource allocation: Resources are allocated more effectively by focusing on high-potential leads, optimizing marketing spend.
Steps to Implement Predictive Analytics
- Data Collection: Gather data from various touchpoints such as website visits, customer interactions, and social media activities.
- Data Processing: Clean and prepare the data for analysis, ensuring it is accurate and structured.
- Model Development: Use machine learning models to analyze the data and predict future behaviors.
- Campaign Optimization: Implement the insights gained from the predictive models to personalize marketing campaigns and offers.
- Continuous Monitoring: Track the performance of the campaigns and adjust strategies based on ongoing results.
By leveraging predictive analytics, businesses can not only increase the effectiveness of their marketing efforts but also provide customers with a more tailored and relevant experience, fostering long-term loyalty.
Example of Predictive Analytics in Action
Data Source | Analysis Type | Outcome |
---|---|---|
Customer Purchase History | Predict future purchases based on past behavior | Targeted email campaigns with personalized product recommendations |
Website Browsing Data | Identify preferences and interests | Customized landing pages based on individual interests |
Social Media Interaction | Gauge sentiment and engagement levels | Real-time content tailored to current trends and interests |