Ai-powered Lead Scoring
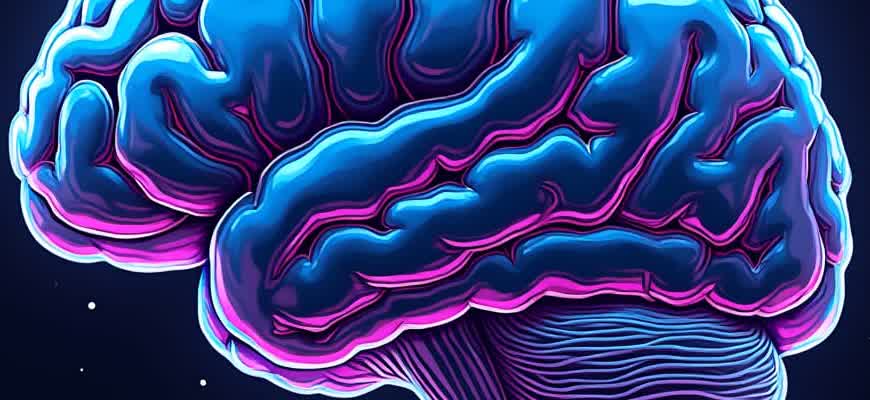
Lead scoring has traditionally been a manual and subjective process. However, with the integration of AI technologies, businesses can now automate and refine their lead evaluation, making it more data-driven and accurate. AI-powered lead scoring models utilize historical data, behavioral insights, and predictive analytics to assess leads' likelihood to convert into customers.
Instead of relying on human intuition or simple demographic data, AI systems analyze complex patterns in large datasets. These models continuously learn and improve their accuracy over time, offering more reliable scoring results. Here are some of the core benefits of AI-driven lead scoring:
- Data-Driven Decisions: AI algorithms assess vast amounts of customer interactions and behaviors to predict the most valuable leads.
- Improved Efficiency: Automation eliminates the need for manual data entry, saving time for sales teams.
- Predictive Capabilities: AI models forecast which leads are most likely to convert based on patterns and trends from previous interactions.
The effectiveness of AI-powered lead scoring is evident when comparing traditional methods with advanced AI approaches. The table below illustrates the key differences:
Criteria | Traditional Lead Scoring | AI-Powered Lead Scoring |
---|---|---|
Data Utilization | Basic demographic and firmographic data | Behavioral, transactional, and engagement data |
Scoring Method | Manual assignment and subjective judgment | Automated, data-driven, and machine learning algorithms |
Accuracy | Often inconsistent and prone to errors | High accuracy with continuous learning from new data |
"AI-powered lead scoring systems bring precision and objectivity to the lead qualification process, enabling sales teams to focus on high-potential opportunities."
AI-Driven Lead Scoring: Practical Insights
AI-powered lead scoring leverages machine learning algorithms to prioritize potential customers based on their likelihood to convert. By analyzing past interactions, behavior patterns, and demographic data, AI can assess leads more accurately and efficiently than traditional methods. This data-driven approach helps sales teams focus their efforts on high-potential prospects, improving conversion rates and overall sales efficiency.
One of the main advantages of using AI for lead scoring is its ability to continuously learn and adapt. Unlike manual systems that rely on static criteria, AI models evolve based on new data, ensuring the scoring remains relevant and accurate. The result is a more streamlined sales process, where the focus is directed at leads with the highest probability of closing, reducing wasted effort and increasing revenue.
How AI Enhances Lead Scoring Accuracy
AI-based lead scoring uses a variety of data points to build a comprehensive profile of each lead. This may include factors like engagement with emails, website behavior, social media interactions, and previous purchase history. The AI system then assigns a score to each lead based on how closely their actions align with those of past successful conversions.
- Behavioral Data: Actions such as downloading content or interacting with emails are weighted to determine lead engagement.
- Demographic Information: Age, industry, job title, and company size help assess a lead's suitability.
- Predictive Analytics: AI models predict the likelihood of conversion based on historical trends and patterns.
Key Benefits of AI-Driven Lead Scoring
AI-powered lead scoring reduces manual effort and allows sales teams to prioritize high-value leads, increasing the chances of successful conversions.
- Increased Precision: AI ensures that only the most promising leads are passed on to sales teams, improving conversion rates.
- Time Savings: Automating the scoring process saves time and resources by eliminating the need for manual evaluation.
- Continuous Improvement: Machine learning models update in real-time, enhancing scoring accuracy based on new data and trends.
Key Metrics in AI Lead Scoring
AI lead scoring is built on several critical data points. Understanding these metrics is essential for maximizing the system's effectiveness:
Metric | Impact |
---|---|
Engagement Level | Higher engagement increases the likelihood of conversion. |
Lead Demographics | Targeting leads within your ideal customer profile boosts conversion potential. |
Behavioral Patterns | Predicts a lead's readiness to purchase based on past actions. |
How AI Improves Lead Scoring Precision for Enhanced Sales Conversion
AI-driven lead scoring leverages advanced algorithms to analyze vast amounts of data quickly, making the process of identifying high-potential prospects much more efficient. Traditional lead scoring models often rely on basic demographic data or manual scoring criteria, which can be inconsistent and prone to human error. By integrating artificial intelligence, companies can predict which leads are most likely to convert with greater accuracy, improving overall sales outcomes.
AI enhances the process by continuously learning from new data inputs, adjusting its algorithms to improve over time. This allows sales teams to focus on the most promising leads, reducing wasted effort and optimizing resource allocation. AI also factors in a wider range of variables, including engagement behaviors, past interactions, and even external signals such as social media activity.
Key Benefits of AI in Lead Scoring
- Data-Driven Insights: AI analyzes both structured and unstructured data, creating a more holistic view of each lead.
- Continuous Learning: AI models adjust and optimize over time, enhancing accuracy based on new data trends.
- Segmentation and Prioritization: AI can segment leads more precisely, allowing sales teams to focus efforts on the most valuable prospects.
How AI Increases Lead Conversion Rates
- Advanced Predictive Analytics: By predicting which leads are most likely to convert, AI allows sales teams to target high-value prospects effectively.
- Behavioral Scoring: AI tracks lead behavior across multiple channels and incorporates this data into scoring models.
- Automated Adjustments: AI constantly refines its models, adjusting lead scores based on new interactions or patterns, ensuring the system evolves with the business needs.
"AI-powered lead scoring not only improves conversion rates but also creates a dynamic sales strategy that adapts as customer behavior evolves."
AI Lead Scoring vs Traditional Lead Scoring
Feature | Traditional Lead Scoring | AI-Powered Lead Scoring |
---|---|---|
Data Types | Demographic, firmographic | Behavioral, contextual, external signals |
Scoring Process | Manual, static | Automated, dynamic, continuously optimized |
Accuracy | Limited, often inconsistent | High, continuously refined based on new data |
Key Factors That AI Analyzes in Lead Scoring Models
AI-driven lead scoring models rely on various data points and behavioral indicators to predict the likelihood of a lead converting into a customer. These models leverage advanced algorithms to assess and prioritize leads based on both explicit and implicit signals. By evaluating a range of factors, AI systems can assign more accurate scores, helping sales teams focus their efforts on high-potential prospects.
The following factors are crucial in AI-powered lead scoring models:
1. Demographic Information
AI systems consider various demographic factors to determine whether a lead fits the ideal customer profile. These data points include:
- Industry - Certain industries may show higher conversion rates based on past data.
- Company Size - Larger organizations may require a different approach than smaller businesses.
- Job Title/Role - Decision-makers or influencers are more valuable leads.
2. Behavioral Signals
AI algorithms analyze how leads interact with your brand across different channels. These behaviors provide deep insights into a lead's intent and readiness to buy:
- Website Visits - Frequent visits to high-conversion pages or product-specific pages increase a lead’s score.
- Content Engagement - Downloading whitepapers, reading blogs, or attending webinars shows interest in your offerings.
- Social Media Interactions - Likes, shares, or comments on social platforms signal engagement levels.
AI models can track and prioritize leads based on real-time activities, making them more dynamic and responsive than traditional scoring methods.
3. Historical Data and Previous Interactions
AI also takes into account past interactions between the lead and your business, including:
Factor | Description |
---|---|
Lead Source | Leads coming from referral programs or inbound channels may score higher due to their demonstrated interest. |
Engagement Recency | Leads who have interacted with your brand recently are often considered higher priority. |
These data points, when analyzed collectively, help AI models make smarter decisions and assist sales teams in identifying the best prospects more efficiently.
Integrating AI-based Lead Scoring into Your Existing CRM System
Integrating artificial intelligence (AI) into your current Customer Relationship Management (CRM) system can significantly enhance lead scoring accuracy. AI algorithms can analyze vast amounts of customer data and predict the likelihood of a lead converting into a paying customer. By integrating AI-based lead scoring, you enable your sales and marketing teams to prioritize high-value prospects, improving efficiency and ultimately boosting conversion rates.
The integration process involves connecting AI tools with the CRM platform in a way that enriches existing lead data. It uses machine learning models to assess behavioral patterns, historical interactions, and engagement levels. As a result, the CRM becomes more intelligent, allowing for better segmentation and targeted outreach.
Key Steps for Successful Integration
- Data Collection: Ensure that all relevant customer data (website visits, email responses, purchase history, etc.) is stored within your CRM.
- Choose the Right AI Tool: Select an AI solution that aligns with your CRM's capabilities and supports custom lead scoring models.
- Model Training: Train the AI model on historical data to develop accurate scoring algorithms tailored to your specific business needs.
- Continuous Monitoring: Regularly update the model with new data to keep the AI's predictions relevant as market conditions change.
Challenges and Considerations
It’s essential to ensure that your team has the necessary skills to interpret AI-generated insights. Integration isn’t just a technical challenge; it requires strategic alignment across departments to get the most out of the new system.
- Data Quality: The effectiveness of AI-based lead scoring relies heavily on the quality and accuracy of the data it processes.
- System Compatibility: Ensure that the AI tool integrates seamlessly with your CRM platform, minimizing technical issues.
- Scalability: As your business grows, the lead scoring system should be flexible enough to scale with your expanding customer base.
Comparison Table: Traditional vs AI-Enhanced Lead Scoring
Factor | Traditional Lead Scoring | AI-Based Lead Scoring |
---|---|---|
Data Processing | Manual input and predefined rules | Automatic processing of large data sets with predictive models |
Accuracy | Limited to preset criteria | Highly accurate, continually improving with new data |
Scalability | Limited to small data sets | Scalable to handle large, dynamic datasets |
Reducing Manual Effort in Lead Qualification with AI Algorithms
In traditional lead qualification processes, sales teams often spend a significant amount of time manually assessing leads based on basic data points. This approach can be both time-consuming and error-prone, leading to missed opportunities or wasted resources. However, AI-powered systems can automate the scoring process by analyzing large datasets, identifying patterns, and predicting the likelihood of a lead converting into a customer with higher accuracy.
By leveraging machine learning models, businesses can streamline the lead qualification process, reducing manual effort and improving efficiency. AI algorithms analyze factors such as engagement history, company size, industry relevance, and behavioral signals to prioritize leads, helping sales teams focus their efforts on high-potential prospects.
Key Benefits of AI in Lead Qualification
- Faster Decision-Making: AI models can instantly score leads based on historical data, allowing for quicker prioritization and follow-up.
- Increased Accuracy: AI systems can identify subtle patterns and trends that might be overlooked by human evaluators, leading to more precise lead qualification.
- Reduced Human Bias: By relying on data-driven insights, AI eliminates subjective biases in the qualification process.
How AI Reduces Manual Effort
- Automated Data Analysis: AI models process vast amounts of customer interaction data in real-time, ranking leads based on pre-defined criteria.
- Behavioral Insights: Machine learning can track user behavior, such as website visits and email interactions, to assess the likelihood of a lead's engagement.
- Continuous Learning: AI systems improve over time by learning from new data, ensuring that the scoring process becomes more refined and accurate with each iteration.
"AI-powered lead scoring allows sales teams to focus on what really matters–engaging with the most promising prospects, rather than manually filtering through hundreds of leads."
AI-Driven Lead Scoring vs. Traditional Methods
Aspect | AI-Driven Lead Scoring | Traditional Methods |
---|---|---|
Data Analysis | Automated, continuous processing of large datasets | Manual review of basic data points |
Speed | Real-time scoring, instant prioritization | Time-consuming, slow to process |
Accuracy | High, based on data-driven insights | Low, prone to human error and bias |
Scalability | Can handle a large volume of leads without additional resources | Requires more human resources as the volume grows |
Real-Time Lead Scoring: How AI Adjusts Lead Prioritization
In the competitive world of sales, ensuring that sales teams focus on high-quality leads is crucial for maximizing conversion rates. AI-driven lead scoring systems offer the ability to dynamically adjust lead prioritization based on real-time data and evolving interactions. These systems analyze a variety of behavioral and demographic signals to continuously refine lead scores, helping teams stay ahead of potential opportunities.
By leveraging machine learning algorithms, these tools can quickly interpret complex patterns in large datasets and make decisions faster than traditional manual scoring. As a result, AI-powered platforms enable businesses to respond to leads with a much higher degree of relevance, often in real time, adjusting their strategies as new data emerges.
How AI Refines Lead Scoring in Real-Time
AI continuously updates its scoring model based on various factors that influence the likelihood of a lead converting. These updates are performed without human intervention, providing a more agile approach to lead management. Here’s how AI refines the process:
- Behavioral Signals: Every interaction a lead has with your website, emails, or social media is tracked and analyzed. AI assesses whether these actions indicate a higher intent to purchase.
- Demographic Information: The system integrates real-time data about a lead’s company, role, or industry to adjust scoring based on the potential fit.
- Engagement Patterns: If a lead consistently engages with your content, AI updates its score to reflect a higher likelihood of conversion.
Key Factors Influencing AI Lead Prioritization
AI algorithms rely on several parameters that evolve as interactions occur. The system continuously analyzes and adapts these factors:
- Lead Activity: Frequency and type of engagement are closely monitored. AI increases priority for leads who demonstrate high levels of interaction.
- Lead Source: The origin of the lead (e.g., paid ads, organic search, referrals) influences the initial score, but this is recalibrated as more data is gathered.
- Lead’s Buying Stage: AI can detect where a lead is in their purchasing journey, prioritizing those who are closer to making a decision.
AI-powered lead scoring not only tracks static information but dynamically shifts priorities based on fresh insights, enabling sales teams to act promptly and efficiently.
Example of AI Lead Scoring in Action
Consider the following table that shows how a lead’s score may change based on real-time interaction with the company’s content:
Lead Activity | Initial Score | Updated Score |
---|---|---|
Downloaded a whitepaper | 45 | 60 |
Attended a webinar | 60 | 80 |
Requested a product demo | 80 | 95 |
Real-time lead scoring can significantly enhance sales team efficiency by highlighting the most promising leads based on up-to-date behavioral data and engagement signals.
Improving Customer Insights with AI-Enhanced Lead Evaluation
Artificial intelligence (AI) has revolutionized the process of lead evaluation, enabling businesses to gain deeper insights into customer behavior and intent. Traditional lead scoring methods often rely on manual input or simple criteria, which can be time-consuming and inaccurate. With AI-driven lead scoring, companies can assess prospects more efficiently by analyzing a wide array of data points and identifying patterns that predict the likelihood of conversion.
By leveraging AI, businesses can segment their audience based on specific behaviors, preferences, and engagement history. This approach leads to more precise and actionable insights that inform sales strategies and marketing campaigns, improving conversion rates and optimizing resource allocation.
Key Benefits of AI-Based Lead Scoring
- Accurate Prediction: AI algorithms analyze past customer interactions to predict future behavior, allowing companies to prioritize leads that are more likely to convert.
- Real-Time Adjustments: AI continuously updates lead scores based on new data, ensuring that businesses have the most up-to-date insights on customer potential.
- Personalization: By analyzing customer preferences, AI enables more tailored communications, increasing engagement and the chances of a successful sale.
AI-Driven Lead Scoring Process
- Data Collection: Gather data from various sources, including website visits, social media interactions, and past purchases.
- Behavior Analysis: AI examines customer actions and engagement levels to assess their readiness to make a purchase.
- Lead Scoring: AI assigns scores to each lead based on the likelihood of conversion, considering both demographic and behavioral factors.
- Continuous Optimization: The AI system learns from new data and refines the scoring model to improve its accuracy over time.
Table: AI vs. Traditional Lead Scoring
Aspect | AI-Based Scoring | Traditional Scoring |
---|---|---|
Data Processing | Real-time, continuous analysis of large data sets | Manual or simple rule-based evaluation |
Accuracy | Highly accurate due to data-driven predictions | Often based on limited or outdated criteria |
Scalability | Handles vast amounts of data efficiently | Becomes less effective as the volume of leads grows |
Important Insight: AI-powered lead scoring enhances decision-making by providing detailed, real-time customer insights, significantly boosting conversion rates and sales efficiency.
Assessing the Effectiveness of AI-Driven Lead Scoring Solutions
When evaluating AI-based lead scoring tools, measuring their return on investment (ROI) is crucial for businesses to understand the value they bring to sales processes. These tools can streamline lead qualification and enhance targeting efforts, but it’s important to have clear metrics to assess whether the investment is delivering measurable benefits. ROI is not only about immediate sales conversions but also the improvement in efficiency, lead quality, and long-term customer relationships. The more granular the data collected, the better the tools can optimize processes and demonstrate tangible outcomes.
To properly gauge ROI, businesses need to track a mix of quantitative and qualitative metrics. While metrics like lead conversion rates and sales cycle length are often emphasized, other factors such as improved lead nurturing effectiveness and reduced cost-per-lead should also be considered. AI-powered tools allow for automation of lead scoring, reducing manual work and improving the accuracy of predictions, which leads to more informed decisions and better resource allocation.
Key Metrics to Measure ROI
- Lead Conversion Rate: The percentage of leads that turn into paying customers after being scored by the AI tool.
- Sales Cycle Length: The time it takes to move a lead through the sales funnel. Shorter cycles often indicate higher-quality leads.
- Lead Quality: The improvement in lead quality as determined by the AI tool’s predictive accuracy.
- Cost per Lead: The reduction in the cost of acquiring a qualified lead, thanks to more precise lead scoring.
ROI Calculation Methods
- Compare the performance of leads before and after the AI tool is implemented, focusing on conversion rates and sales cycle efficiency.
- Analyze the reduction in manual labor hours spent on lead qualification.
- Evaluate long-term customer retention rates, as more accurate lead scoring often results in better-fit customers.
"AI-powered tools not only reduce the time spent on lead qualification but also increase the likelihood of closing sales with more accurate lead predictions."
Example ROI Table
Metric | Before AI Tool | After AI Tool | Change |
---|---|---|---|
Lead Conversion Rate | 15% | 25% | +10% |
Sales Cycle Length | 45 days | 30 days | -15 days |
Cost per Lead | $50 | $35 | -30% |