Ai-powered Email Recommendation Engines
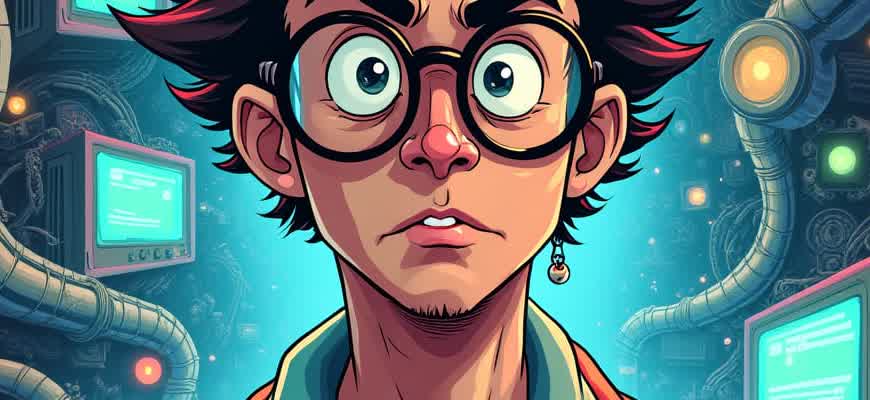
The integration of artificial intelligence into email marketing has revolutionized the way businesses engage with their customers. By utilizing machine learning algorithms, these systems are able to analyze large datasets to tailor email content to individual preferences, enhancing user engagement and conversion rates.
Key Benefits of AI Email Personalization:
- Automated content optimization based on user behavior
- Improved customer segmentation for targeted campaigns
- Real-time adjustments to email content, boosting relevance
Components of AI-Powered Recommendation Engines:
- Data Collection: Gathering customer data from various sources like browsing history, past purchases, and email interactions.
- Behavioral Analysis: Using AI to detect patterns in user behavior and preferences.
- Content Generation: Automatically generating personalized email content based on the analyzed data.
"AI-driven systems continuously evolve by learning from user responses, allowing for real-time refinement of email strategies."
Example of AI-driven Email Content Customization:
User Activity | Email Content Type | Action Taken |
---|---|---|
Viewed product A | Recommendation of similar products | Increased click-through rate by 30% |
Abandoned cart | Discount offer on cart items | Recovered 15% of abandoned sales |
AI-Driven Email Personalization Systems
AI-driven systems for personalizing email content have become essential tools for enhancing user engagement and improving email marketing strategies. These technologies utilize advanced machine learning algorithms to analyze user behavior, preferences, and previous interactions, tailoring email recommendations in a way that maximizes relevance. By leveraging large data sets and real-time inputs, these systems continuously optimize the content delivered to each individual, leading to more effective communication and higher conversion rates.
At the heart of these recommendation engines is the ability to predict what content a user is most likely to engage with based on their past actions. This predictive capability not only boosts the chances of an email being opened but also ensures that the right message is delivered at the right time, enhancing the overall user experience.
Key Features of AI Email Personalization Systems
- Behavioral Analysis: Algorithms track user actions, including clicks, open rates, and past purchases, to understand preferences and predict future behavior.
- Dynamic Content Generation: The system adapts email content on the fly, creating messages that are highly relevant to each recipient.
- Segmentation and Targeting: Machine learning models segment users into precise categories based on their engagement patterns, ensuring more accurate targeting.
- Real-Time Adjustments: AI systems can update email content in real-time based on user interactions and contextual factors like time of day or device used.
How AI Improves Email Recommendations
- Higher Engagement Rates: By tailoring content to individual interests, AI systems increase the likelihood that users will engage with the email.
- Optimized Delivery Times: AI can determine the best time to send emails to each user, increasing the chance of the email being seen and interacted with.
- Continuous Improvement: AI-driven systems learn from each interaction, constantly refining their recommendations to become more accurate over time.
"AI-powered email recommendation engines have transformed marketing strategies by allowing companies to deliver personalized content that resonates with each user, boosting both engagement and customer satisfaction."
Example of an Email Personalization Flow
Stage | AI Process | Outcome |
---|---|---|
Initial Interaction | Collect user behavior data (clicks, opens, time spent) | Personalized email content creation based on preferences |
Ongoing Interaction | Real-time content updates based on new behavior | Higher relevance and engagement for future emails |
Post-Email | Analyze interaction data to refine future recommendations | Continuous optimization of email content and timing |
How AI Algorithms Tailor Email Content Based on User Actions
Email marketing has evolved significantly with the rise of artificial intelligence (AI), allowing businesses to deliver more personalized and relevant content to their subscribers. AI systems analyze user behavior to predict their preferences, enabling the automation of email content customization. These algorithms gather data from user interactions, such as past purchases, click-through rates, and browsing history, to dynamically adjust the content in each email. This creates a more engaging and tailored experience for the recipient, improving open rates and conversions.
AI-driven engines rely on sophisticated models to recognize patterns in user actions. By leveraging these insights, marketers can create content that resonates with individual subscribers. The core idea is to deliver emails with content, offers, and recommendations based on what the user is most likely to engage with, thus increasing the relevance of every interaction.
Behavioral Data Analysis
AI algorithms utilize various behavioral signals to personalize email content, including:
- Browsing activity: Which pages or products the user has viewed recently.
- Past purchase history: Items the user has bought or shown interest in.
- Engagement patterns: Open and click-through rates for previous emails.
- Time of interaction: When users tend to engage with emails (e.g., morning, evening).
Personalization Techniques
Once the AI system analyzes this data, it can employ different strategies to tailor the email content:
- Dynamic Content: Content blocks in the email that change based on individual user data (e.g., product recommendations).
- Subject Line Optimization: Personalizing the subject line based on past interactions or preferences.
- Timing Optimization: Sending emails at the times when users are most likely to engage.
"By understanding user behavior, AI can create hyper-targeted emails that feel less like a marketing message and more like a personalized experience."
Impact on Conversion Rates
Personalized email marketing powered by AI can significantly increase engagement. When content resonates with the recipient, they are more likely to open the email and follow through with a purchase or other desired action. Here's a table showing how different personalization tactics impact key metrics:
Personalization Tactic | Impact on Open Rate | Impact on Conversion Rate |
---|---|---|
Dynamic Product Recommendations | +25% | +15% |
Subject Line Customization | +18% | +10% |
Optimized Send Time | +20% | +12% |
Integrating AI-driven Email Recommendations with Existing Marketing Platforms
Integrating AI-powered email recommendation systems with current marketing platforms can significantly enhance the relevance and personalization of email campaigns. By leveraging machine learning algorithms, these systems analyze customer behavior, preferences, and past interactions to deliver more targeted content. This creates a seamless experience for customers while driving higher engagement rates for marketers. The integration process, however, requires thoughtful consideration of data flow, system compatibility, and user experience to ensure smooth operation within existing marketing ecosystems.
Successful integration hinges on understanding how AI recommendations can complement the existing workflows of marketing platforms. Most email service providers (ESPs) and Customer Relationship Management (CRM) systems already collect vast amounts of user data, making them prime candidates for AI recommendation engine integration. By enhancing these platforms with AI, marketers can optimize their campaigns without a complete overhaul of their current systems, making the transition more cost-effective and efficient.
Key Integration Steps
- Data Synchronization: Ensure smooth data flow between the AI system and the existing platform. This involves syncing customer data, such as purchase history and browsing behavior, with the recommendation engine.
- API Integration: Use APIs to connect the AI recommendation engine to the marketing platform. This allows for real-time personalization and dynamic email content updates.
- Testing & Calibration: Continuously monitor the performance of the AI system within the platform, adjusting recommendation algorithms based on user response and campaign performance.
Challenges to Address
"Integrating AI into an existing marketing framework can be challenging, especially when dealing with siloed data and inconsistent formats. Proper data preparation is essential for accurate AI-driven insights."
- Data Integration: Aligning disparate data sources may require significant effort in cleaning and unifying information.
- System Compatibility: Ensuring that AI recommendations can be seamlessly integrated without disrupting other marketing workflows.
- Real-time Processing: Optimizing the recommendation engine to process and deliver relevant content in real-time without delays.
Benefits of Integration
Benefit | Description |
---|---|
Increased Engagement | Personalized email content based on AI recommendations leads to higher open and click-through rates. |
Enhanced Customer Experience | Customers receive more relevant offers and product suggestions, improving their interaction with the brand. |
Improved ROI | Higher engagement and more precise targeting result in a better return on investment for email campaigns. |
Optimizing Email Open Rates with Machine Learning-Powered Suggestions
Machine learning (ML) has become a powerful tool for enhancing email engagement. By analyzing past interactions and learning user preferences, AI-driven systems can recommend highly personalized email content. This data-driven approach helps marketers deliver tailored messages that resonate with individual recipients, significantly boosting open rates. Key algorithms like natural language processing (NLP) and clustering models are utilized to process vast amounts of user data, enabling the system to predict what will grab the recipient’s attention.
Incorporating machine learning into email campaigns involves several strategies. These include optimizing subject lines, determining the best time to send emails, and suggesting content that aligns with the recipient’s interests. ML algorithms constantly refine their predictions based on continuous feedback, making each email more targeted and impactful. As a result, open rates are likely to improve, reducing the need for broad, generic campaigns.
Key Machine Learning Techniques for Email Optimization
- Personalized Subject Line Generation: ML algorithms analyze past email subject lines that have garnered high engagement and generate new variations based on those insights.
- Send Time Optimization: By examining when a user is most likely to engage with an email, machine learning systems predict the optimal send time, boosting the chance of opening.
- Content Personalization: Machine learning algorithms segment users into clusters based on behavior and preferences, allowing for the suggestion of highly relevant content.
Approaches to Achieve Higher Open Rates
- Behavioral Tracking: Collect data on user actions like previous email opens, clicks, and conversions to fine-tune future recommendations.
- Subject Line Testing: Continuously test subject lines using ML-driven A/B testing to find which ones generate higher open rates.
- Dynamic Content Delivery: Deliver emails with content tailored to specific user interests, powered by machine learning models that predict relevance.
Example: Subject Line Variations and Open Rate Impact
Subject Line | Open Rate |
---|---|
“You won’t want to miss this!” | 25% |
“Exclusive Offer Just for You!” | 32% |
“Limited Time Deal – Act Now!” | 28% |
Machine learning continuously adapts, improving open rates by personalizing subject lines and timing, ultimately increasing engagement with each campaign.
Analyzing Data Patterns for Accurate AI-based Email Personalization
AI-powered email recommendation systems use advanced data analysis to understand user behavior and predict the most relevant content for individual recipients. By examining patterns such as previous purchases, email engagement, and browsing history, these systems can generate personalized email content that aligns with a user’s preferences and interests. This data-driven approach helps ensure that every email sent is relevant, increasing the likelihood of interaction and conversion.
As users continue to engage with content, the AI model adapts, fine-tuning its predictions based on new data. This continuous learning cycle allows the system to stay aligned with shifting user preferences and ensures that email content remains fresh and timely. By leveraging real-time data, the system can dynamically adjust email strategies to maintain high engagement and meet the evolving needs of the user.
Essential Data Elements for Personalization
To ensure highly personalized emails, AI systems rely on several key data points:
- Past Engagement: Understanding past email opens, click-through rates, and interactions provides valuable insights into user preferences.
- Demographic Information: User attributes such as age, gender, and location help in segmenting and targeting the right content.
- Behavioral Data: Analyzing browsing and purchasing behaviors helps predict future interests and suggest relevant products or content.
Approaches to Identifying Behavioral Patterns
AI systems utilize a variety of strategies to detect and predict user behavior patterns:
- Collaborative Filtering: This method identifies users with similar behaviors and recommends content based on what others in the same group have engaged with.
- Content-Based Filtering: Content-based filtering suggests items similar to those the user has already interacted with, making recommendations more relevant.
- Hybrid Models: Combining collaborative and content-based filtering techniques can improve recommendation accuracy by considering both individual and group behavior patterns.
Key Trends for Optimizing Email Content
Identifying important data patterns helps fine-tune email strategies for better engagement:
Behavioral Insight | Impact on Personalization |
---|---|
Engagement Time | Sending emails when users are most likely to open them increases the chances of higher interaction rates. |
Interaction History | Recommending content based on a user’s previous interactions or purchases increases relevance and engagement. |
Engagement Frequency | Adjusting the frequency of emails based on user engagement helps prevent saturation and keeps content fresh. |
"Personalization is most effective when it takes into account not only what the user likes but also when and how often they prefer to interact."
Setting Up Automated Workflows for AI Email Recommendations
Integrating AI-powered email recommendation systems into your workflow involves creating automated processes that can deliver personalized content to users based on their preferences and behavior. These systems rely on machine learning algorithms that continuously learn from user interactions to optimize the content of email campaigns. By automating the process, companies can reduce manual effort while improving the relevance and effectiveness of their email communications.
To implement such a system, it's essential to design workflows that are both efficient and flexible. The following steps outline the necessary components to set up automated workflows for email recommendations powered by AI:
Key Steps for Workflow Automation
- Data Collection: Gather data from various touchpoints, such as website visits, user purchases, and email interactions. This data is crucial for feeding the recommendation engine.
- Segmentation: Automatically segment users based on behaviors and preferences. This allows the AI system to tailor recommendations to each segment.
- Recommendation Engine: Integrate the AI engine that processes the data and generates personalized recommendations. The engine uses predictive algorithms to suggest content based on user behavior and preferences.
- Email Trigger: Define automated email triggers based on user actions, such as abandoned cart emails, product recommendations after a purchase, or special offers based on browsing history.
- Optimization: Continuously monitor the results of the email campaigns and optimize the recommendations based on performance data.
Automated Workflow Example
- Gather data from user activity across your platform.
- Segment users into categories based on past interactions.
- Generate personalized product recommendations using an AI engine.
- Trigger email campaigns when users meet predefined criteria (e.g., cart abandonment, specific interests).
- Track user responses and update the recommendation engine accordingly to improve future suggestions.
"By automating recommendation workflows, businesses can not only save time but also enhance user engagement by delivering highly relevant content to their audience."
Workflow Automation Best Practices
Best Practice | Description |
---|---|
Personalization | Ensure each email is tailored to individual user preferences and behavior. |
Real-Time Data | Use real-time data to adapt recommendations as user behavior changes. |
Regular Optimization | Regularly review and adjust your workflows based on campaign performance and user feedback. |
Maximizing Engagement: Using AI to Tailor Email Subject Lines and Content
AI-driven email optimization strategies are rapidly transforming how businesses engage with their audience. By analyzing user behavior, preferences, and past interactions, AI can craft personalized subject lines and email content that increase open rates and conversions. The ability of AI to test and iterate these elements ensures that every email sent is as relevant as possible to its recipient.
Utilizing AI tools to modify subject lines and content based on user insights is crucial for maximizing engagement. These tools assess various data points, such as browsing history and purchasing patterns, to create highly targeted messages. Over time, the AI adapts to the changing preferences of users, ensuring the content remains fresh and aligned with their interests.
Personalization of Email Subject Lines
- Behavior-based customization: AI analyzes users' interaction history to create subject lines that resonate with their interests.
- Time-sensitive offers: AI can tailor subject lines to reflect urgency, prompting recipients to act quickly.
- Testing and optimization: A/B testing powered by AI allows for real-time adjustments to subject lines based on engagement metrics.
AI-Driven Content Personalization
- Dynamic content insertion: Emails can be dynamically altered to display personalized product recommendations, content, or offers.
- Segmentation: AI segments email lists based on user behavior, ensuring that content is targeted to specific groups.
- Language preferences: AI adjusts the tone and language of the email to align with the recipient's preferences and past interactions.
AI empowers marketers to send highly relevant messages, increasing the likelihood of recipient engagement and reducing unsubscribe rates.
Table: AI-Driven Subject Line Testing Results
Subject Line | Open Rate (%) | Click-Through Rate (%) |
---|---|---|
Exclusive Offer Just for You! | 45 | 12 |
Don't Miss Out on This Limited-Time Deal | 40 | 10 |
Your Personalized Recommendations Inside | 50 | 15 |
Monitoring and Adjusting AI Recommendations for Ongoing Campaign Success
When implementing AI-driven systems for email campaign optimization, continuous monitoring is critical for sustained effectiveness. The goal is not only to identify performance issues but also to ensure that the recommendations align with the evolving needs of the audience. Regular tracking of key metrics can provide insights into the performance and help refine AI algorithms. This iterative process allows marketers to adapt to changing consumer behaviors, leading to better engagement rates and overall campaign success.
Adjustment is a vital part of ensuring that AI recommendations remain relevant. As data accumulates and new trends emerge, AI models must be fine-tuned. This includes adjusting parameters such as content suggestions, timing, and targeting strategies. The constant evaluation and modification of these factors can significantly improve the precision of recommendations, making campaigns more efficient and effective over time.
Key Metrics to Track
- Open Rates: Indicates how well the subject lines and content resonate with the audience.
- Click-through Rates (CTR): Measures the effectiveness of the call-to-action in email content.
- Conversion Rates: Tracks how many recipients complete the desired action.
- Unsubscribe Rates: Signals if the email content or frequency is not aligned with audience expectations.
- Engagement Time: Shows how much time recipients spend interacting with the email.
Steps for Ongoing Adjustment
- Data Collection: Gather data from email campaigns, analyzing user engagement and feedback.
- AI Algorithm Refinement: Update AI models based on new data to improve recommendation accuracy.
- Personalization Adjustments: Tailor content, timing, and delivery based on the evolving preferences of the target audience.
- Testing: A/B test various changes to determine the impact on key metrics.
- Optimization: Implement successful changes across the entire campaign.
Important Note: Regular fine-tuning of AI algorithms based on live feedback is essential to ensure continued campaign relevance and maximize ROI.
AI Recommendation Adjustments Table
Adjustment Type | Impact on Campaign |
---|---|
Subject Line Updates | Increases open rates by capturing user interest. |
Content Personalization | Boosts engagement by delivering relevant offers. |
Timing Optimization | Improves delivery windows for higher engagement. |