Analytics Engagement Model
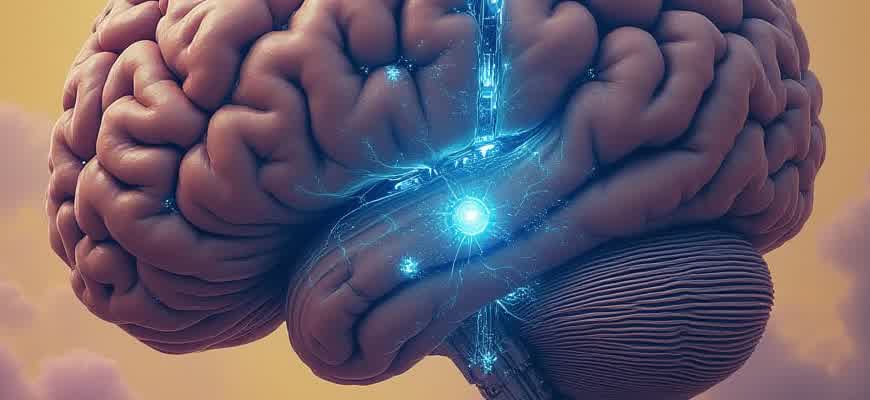
The Analytics Engagement Model outlines the structured approach organizations use to leverage data analytics for decision-making and strategic planning. It focuses on the interactions between data scientists, analysts, and key stakeholders, ensuring that data-driven insights align with business objectives. This framework supports organizations in optimizing their data processes, enhancing collaboration, and driving measurable outcomes.
At its core, the model consists of several key components that define the flow of analytics engagement:
- Data Collection and Preparation: Gathering, cleaning, and structuring relevant data for analysis.
- Analysis and Insights: Employing various techniques to extract actionable insights from the data.
- Collaboration and Communication: Sharing findings with stakeholders to inform decisions and strategies.
Key Benefits of the Analytics Engagement Model:
Benefit | Description |
---|---|
Improved Decision Making | Enables evidence-based decisions that drive business success. |
Efficiency Gains | Optimizes resource allocation and reduces operational inefficiencies. |
Enhanced Collaboration | Fosters better communication between data teams and business units. |
"Data-driven decision-making is no longer a luxury; it's a necessity for businesses aiming to stay competitive in the modern market."
Identifying Key Metrics for Your Analytics Engagement Strategy
Defining the right metrics is critical for evaluating the effectiveness of your analytics engagement model. The goal is to pinpoint which performance indicators will offer clear insights into user behavior, engagement levels, and overall impact. Understanding these metrics will enable businesses to optimize data-driven strategies and make informed decisions for continuous improvement.
To build a successful analytics framework, you must start by identifying metrics that align with your business goals and objectives. Focusing on the right data points ensures that the team is working towards a common purpose and can easily track progress over time. Without clarity on key metrics, tracking and improving engagement becomes challenging.
Key Metrics to Consider
- User Retention: Measures the ability to keep users engaged over time. This can be tracked by monitoring repeat visits or logins.
- Engagement Rate: Indicates how often users interact with your content or platform, helping to gauge the level of interest and satisfaction.
- Conversion Rate: Tracks the percentage of visitors who take a desired action, such as making a purchase or signing up for a service.
- Customer Lifetime Value (CLV): Estimates the total revenue a business can expect from a customer during their relationship.
Steps to Identify the Right Metrics
- Understand Business Goals: Start by clarifying the key business outcomes you're aiming for, whether it's increasing sales, improving customer retention, or enhancing product usability.
- Define Engagement Objectives: Determine the specific actions or behaviors that signify engagement, such as content consumption, interaction, or feedback.
- Align Metrics with Value: Choose metrics that directly relate to the value your business offers to users, ensuring they can be linked to tangible outcomes.
“Choosing the right metrics is not about having the most data, but about having the data that matters to your strategy.”
Example of Key Metrics in Action
Metric | Purpose | Frequency |
---|---|---|
User Retention | Measures the ability to keep users engaged | Monthly |
Engagement Rate | Tracks how actively users engage with content | Weekly |
Conversion Rate | Measures the success of turning visitors into customers | Daily |
Customer Lifetime Value | Estimates long-term revenue from a customer | Quarterly |
Building Data Collection Processes for Accurate Insights
Effective data collection is a cornerstone of any analytics strategy. To derive meaningful insights, organizations must establish clear processes for gathering, validating, and analyzing data. Without a structured approach, data can become fragmented, leading to inaccurate conclusions and missed opportunities. This process involves setting up systematic mechanisms for capturing data, ensuring its quality, and organizing it in a way that aligns with business objectives.
The goal is to ensure that the data collected is both reliable and relevant. This starts by identifying key data sources, defining what metrics matter most, and selecting appropriate tools and technologies to support the collection process. As organizations scale, having an efficient data collection framework becomes increasingly important to avoid bottlenecks and maintain data integrity.
Key Steps in Building a Robust Data Collection System
- Identify Data Sources: Determine where the data will come from, whether it's internal systems, external partners, or third-party services.
- Set Clear Data Objectives: Establish what specific insights you want to derive from the data, ensuring alignment with business goals.
- Select Appropriate Tools: Choose tools and platforms that enable easy data capture, storage, and analysis.
- Ensure Data Quality: Implement processes for data validation and error-checking to avoid issues in the analysis phase.
- Standardize Data Formats: Standardization ensures that all data collected can be easily compared and analyzed across different sources.
Challenges in Data Collection
- Data Overload: Accumulating large volumes of data without a clear purpose can lead to analysis paralysis.
- Data Inaccuracy: Incorrect data can stem from manual entry errors, misconfigured tools, or inconsistent formats.
- Integration Issues: Combining data from multiple sources can be challenging without a unified system in place.
Building a structured, transparent data collection framework is critical for ensuring that insights derived from analytics are actionable and aligned with strategic objectives.
Data Validation Process
Validation Step | Description |
---|---|
Data Cleansing | Removing inaccuracies, inconsistencies, and irrelevant data entries. |
Data Transformation | Converting raw data into a usable format for analysis. |
Data Enrichment | Enhancing data with external sources to add context and value. |
Integrating Analytics Tools into Existing Systems
To successfully integrate analytics tools into an existing system, it is essential to understand the current infrastructure and ensure that the new tools align with business objectives. The integration should enhance data visibility, streamline decision-making processes, and not disrupt the daily operations of the business. A step-by-step approach is often the best way to ensure smooth adoption and functionality.
Effective integration requires a clear understanding of the available data sources, how analytics tools interact with existing software, and what customizations may be needed to provide relevant insights. Below is a guide to help businesses plan and execute the integration of analytics tools into their existing systems.
Key Steps for Integrating Analytics Tools
- Evaluate Current System Requirements: Before integrating any analytics solution, assess the data structures, formats, and compatibility with the existing system architecture. Make sure the tools can interact seamlessly with databases and APIs.
- Choose the Right Analytics Platform: Select a platform that offers the functionality required for your business, such as reporting, forecasting, or machine learning. Consider scalability and flexibility for future growth.
- Data Integration: Utilize APIs or ETL (Extract, Transform, Load) processes to integrate data from various sources into the analytics platform. Ensure that data flows without bottlenecks or losses.
- Testing and Validation: Run test cases to validate the integration and ensure that data is being processed accurately and consistently. Regular testing also helps detect potential errors early.
- Monitoring and Optimization: Continuously monitor the performance of the integrated system and make adjustments to improve speed, efficiency, or accuracy. Use feedback loops to enhance data collection processes.
Important: Successful integration requires ongoing maintenance and a collaborative approach between technical teams and business stakeholders.
Considerations for Smooth Integration
- Customization: Ensure the analytics tools are tailored to specific business needs, whether it’s through custom dashboards, reports, or data filters.
- Security: Implement strong security protocols to protect sensitive data throughout the integration process.
- Training: Offer training for team members to help them understand how to use new tools and interpret data effectively.
Comparison of Analytics Tools Integration Methods
Integration Type | Advantages | Disadvantages |
---|---|---|
API-based | Real-time data exchange, flexible integration | Requires advanced technical expertise, potential latency issues |
ETL Process | Can handle large volumes of data, allows for more structured transformations | Potential delays in data refresh, complex setup |
Embedded Analytics | Seamless user experience, ready-made reporting | Limited customization, higher initial cost |
Measuring User Interaction with Analytics Reports
Understanding how users engage with analytics reports is crucial for improving the relevance and impact of data insights. Effective measurement of user interactions can help optimize report design, ensuring it meets the specific needs of stakeholders. This process goes beyond simply tracking page visits, as it involves capturing detailed information on how users navigate, filter, and interpret the data presented in the reports.
Key metrics such as time spent on a report, number of interactions, and specific actions taken during the session offer valuable insights. By analyzing these metrics, organizations can identify areas where users may face difficulties or lose interest, leading to targeted improvements in report usability and content presentation.
Key Metrics for Measuring Interaction
- Time Spent on Report: Indicates how engaging or useful the content is, and whether users are investing enough time to fully understand the insights.
- Click-through Rates (CTR): Shows how often users interact with specific elements such as links, charts, and buttons within the report.
- Scroll Depth: Reveals how far users scroll through a report, indicating whether they are exploring all available sections or only viewing the most visible data.
- Actions Taken: Measures specific interactions like applying filters, selecting data points, or downloading reports, which can help assess report effectiveness in guiding decision-making.
Techniques for Tracking User Engagement
- Heatmaps: Visual representation of where users click, scroll, or hover within the report, providing a clear picture of user attention areas.
- Session Recording: Captures the user’s journey through the report, allowing for detailed analysis of interaction patterns and pain points.
- Surveys and Feedback Forms: Direct feedback from users can provide qualitative insights into their experience with the report.
Data Collection Methods
Method | Description | Benefits |
---|---|---|
Event Tracking | Monitors user actions such as clicks, filters, and report downloads. | Provides quantitative data for assessing user engagement and pinpointing areas for improvement. |
Session Analytics | Tracks the user’s session time, scroll behavior, and page transitions. | Helps understand how long users interact with specific sections of the report and where they drop off. |
Surveys | Collects user feedback on the usefulness and clarity of the report. | Offers qualitative insights into user satisfaction and areas for enhancing the report’s content and design. |
Measuring how users interact with analytics reports is not just about collecting data, but about transforming that data into actionable insights to improve user experience and report efficacy.
Understanding Customer Behavior through Engagement Analysis
Engagement analysis allows businesses to gain deep insights into customer behavior by examining interactions across various platforms. By tracking and interpreting engagement data, companies can identify trends, preferences, and potential areas for improvement in customer experiences. This process involves monitoring how customers interact with content, products, or services, and understanding the triggers that drive these actions.
Through detailed engagement tracking, businesses can segment their audience based on behavior patterns and customize their strategies accordingly. The analysis of these patterns helps companies predict future behaviors and optimize their engagement efforts. Key metrics like response rates, time spent on platforms, and click-through rates provide valuable data for refining marketing and customer service strategies.
Key Engagement Metrics
- Click-Through Rate (CTR): Measures how often users click on a link, indicating the effectiveness of content or ads.
- Time on Site: Reveals how long customers engage with a website or app, giving insights into their level of interest.
- Interaction Frequency: Tracks how often users engage with content, showcasing the consistency of customer engagement.
- Conversion Rate: Measures the percentage of visitors who complete desired actions, like purchases or form submissions.
Analyzing Engagement through Segmentation
- Behavioral Segmentation: Grouping customers based on their actions, such as frequent buyers or occasional browsers.
- Demographic Segmentation: Categorizing customers by demographic factors, such as age, gender, or location, to understand engagement trends.
- Psychographic Segmentation: Analyzing customer interests and lifestyles to predict future engagement patterns.
Understanding customer behavior is not just about collecting data; it’s about interpreting that data to drive more personalized and effective engagement strategies.
Engagement Impact on Customer Loyalty
Engagement Level | Loyalty Impact |
---|---|
High Engagement | Strong emotional connection, higher retention rates, and repeat purchases. |
Moderate Engagement | Occasional interaction, but lower overall commitment and potential for churn. |
Low Engagement | Minimal connection, high risk of abandonment, and low brand advocacy. |
Optimizing Content Delivery Based on Engagement Insights
In today's digital landscape, delivering relevant content to users is crucial for maintaining high engagement rates. By leveraging data on how users interact with various content formats, platforms can optimize their delivery strategies. The ability to make real-time adjustments based on these insights ensures that content is delivered in a way that resonates with the target audience, ultimately improving user retention and experience. Data-driven content optimization isn't just about personalizing content, but about understanding when and how to present it for maximum impact.
Engagement data provides a wealth of information, such as which types of content lead to longer interaction times, higher conversion rates, or increased social sharing. By interpreting these metrics, content strategies can be refined to address audience preferences more effectively. This approach minimizes content fatigue while enhancing overall satisfaction, ensuring that users consistently find value in the content they consume.
Key Strategies for Optimizing Content Delivery
- Personalized Recommendations: Use engagement data to suggest content tailored to individual preferences, increasing the likelihood of user interaction.
- Dynamic Scheduling: Adjust content delivery times based on when users are most active, improving visibility and engagement.
- Content Format Optimization: Analyze which formats (e.g., videos, articles, infographics) generate the most engagement and prioritize their use.
“Understanding user behavior is the foundation of delivering content that resonates and drives action.”
Evaluating Content Performance
Continuous evaluation of content performance through engagement metrics is essential for long-term success. Key indicators such as click-through rates (CTR), average time spent on content, and interaction rates provide a clear picture of what resonates with the audience. By tracking these metrics over time, content strategies can be fine-tuned for better results.
Engagement Metric | Performance Indicator |
---|---|
Click-Through Rate (CTR) | Measures the effectiveness of content in prompting further interaction. |
Average Time Spent | Indicates how engaging and relevant the content is to users. |
Social Shares | Shows how likely users are to share content, expanding reach. |
By consistently adapting based on these insights, businesses can ensure their content delivery remains relevant, timely, and impactful, fostering greater engagement and user satisfaction in the long run.
Expanding Your Analytics Framework for Business Advancement
As organizations grow, so must their data infrastructure. Scaling analytics is crucial to ensure that decision-making becomes more informed and aligned with evolving business needs. A robust strategy requires integrating analytics across all departments and enhancing data processing capabilities. However, this growth can only happen if businesses refine their processes and adjust their tools as their data needs expand.
The process of scaling analytics involves more than just increasing the amount of data processed. It requires a shift towards more sophisticated models and tools that can handle higher complexity. Effective scaling means improving data access, refining data governance, and increasing the responsiveness of analytic capabilities across various functions.
Key Steps to Scaling Your Analytics Strategy
- Establish a Clear Data Governance Framework - As data volumes grow, managing its quality and accessibility becomes critical. Implementing clear rules for data collection, storage, and sharing ensures consistency and trustworthiness.
- Invest in Scalable Technology - Invest in tools that can handle large datasets and adapt to new demands. Cloud-based platforms and machine learning tools are great choices for flexibility and scalability.
- Develop Cross-Functional Collaboration - Analytics should not be confined to a single department. Encourage collaboration between marketing, finance, operations, and other units to create a more holistic approach to data-driven decisions.
Considerations for Long-Term Growth
- Data Infrastructure Flexibility - Building scalable infrastructure will allow your company to adjust as needs change.
- Employee Training and Engagement - Equip employees with the skills necessary to understand and leverage analytics, ensuring that data insights translate into action.
- Automating Analytics Processes - Automation speeds up the process, allowing analytics teams to focus on higher-value tasks, reducing operational bottlenecks.
"A successful analytics strategy isn’t just about collecting more data – it's about turning that data into actionable insights that drive sustainable growth."
Evaluating Analytics Growth Using Metrics
Metric | Purpose | How to Measure |
---|---|---|
Data Access Speed | How quickly users can access needed data | Measure load times and data retrieval efficiency |
Decision Accuracy | Impact of analytics on business decisions | Track decision success rates before and after analytics implementation |
Employee Adoption Rate | Extent to which employees use analytics tools | Survey employees or track tool usage frequency |