Content Ai Ghl
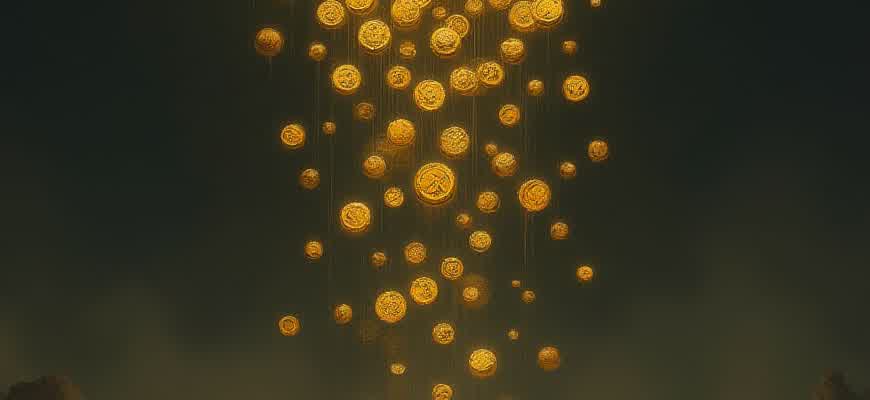
Modern customer acquisition strategies increasingly rely on intelligent automation. Within the GoHighLevel ecosystem, AI-enhanced copywriting tools streamline funnel creation and client outreach, reducing manual workload and increasing personalization efficiency.
- Dynamic content generation for landing pages and email campaigns
- Automated A/B testing with real-time performance insights
- AI-based segmentation for hyper-targeted messaging
Note: Incorporating machine-generated content reduces campaign setup time by up to 60%, significantly boosting productivity in agency workflows.
These capabilities are not standalone. They integrate with CRM features, allowing seamless alignment between client engagement and sales pipeline progression.
- Content personalization based on behavioral data
- Trigger-based automation sequences using AI input
- In-depth analytics for content optimization
Feature | Benefit |
---|---|
Smart Text Generation | Custom copy tailored to target audience personas |
Predictive Scheduling | Optimized delivery time for maximum engagement |
Customizing AI-Generated Copy to Match Your Brand Voice
Automated content tools can draft text at scale, but aligning that output with a specific tone and messaging style requires a structured approach. Precision in terminology, sentence cadence, and emotional tone defines whether AI-generated material fits seamlessly into your brand ecosystem.
To adapt generated text effectively, start by mapping out the verbal identity of your brand: sentence structure, preferred terminology, emotional undertone, and rhythm. These parameters serve as the foundation for guiding AI output through prompt engineering, post-processing, or automated filters.
Steps to Tailor Machine-Written Text to Your Voice
- Define key tonal elements: Is the voice formal or conversational? Minimalist or descriptive?
- Assemble a vocabulary list: Highlight terms to use and words to avoid.
- Use content templates: Create sentence models to maintain structural consistency.
- Review and refine: Post-edit AI drafts to match the desired nuance.
Consistency in brand voice is not a stylistic choice–it's a trust signal for your audience.
- Emotional tone: confident, empathetic, authoritative
- Grammar preferences: use of contractions, sentence length, punctuation norms
- Point of view: first person ("we") or third person ("the company")
Element | Brand A Example | Brand B Example |
---|---|---|
Tone | Direct & Assertive | Friendly & Encouraging |
Vocabulary | Technical, industry-specific | Simple, accessible |
Sentence Structure | Short, action-driven | Flowing, explanatory |
Analyzing Engagement Metrics of AI-Created Content
Monitoring user interactions with machine-generated materials is essential for evaluating their performance. Click-through rates, bounce rates, and average session durations are core indicators that show how well these assets retain attention and drive action. Unlike traditional copy, AI-driven outputs require distinct benchmarking due to their dynamic and often templated nature.
Quantitative metrics must be complemented by qualitative feedback to measure how users perceive the relevance and authenticity of the content. AI can produce large volumes rapidly, but without meaningful engagement, scale becomes irrelevant. Therefore, precision tracking is necessary for identifying which formats, tones, and topics resonate best.
Key Interaction Indicators to Track
- Scroll Depth: Shows how far users consume content before leaving.
- Heatmaps: Identify zones of most frequent interaction.
- Social Sharing: Indicates perceived value and relevance of the content.
High bounce rates on AI-generated pages may indicate a mismatch between search intent and the output's structure or tone.
- Segment content types (listicles, how-tos, narratives).
- Compare performance across distribution channels.
- Integrate user session recordings for context.
Metric | Benchmark | AI Content Goal |
---|---|---|
CTR (Click-Through Rate) | 2-3% | Above 3.5% |
Average Time on Page | 1:30 min | Over 2 min |
Bounce Rate | <60% | <50% |
Optimizing Conversion Paths With AI-Powered A/B Testing Suggestions
Improving the efficiency of user journeys requires more than intuition. Machine learning models integrated with smart testing mechanisms can now detect underperforming segments within funnels and provide actionable variation ideas based on historical data patterns and real-time user behavior.
These intelligent testing systems analyze visitor interactions across landing pages, CTAs, and form sequences, automatically prioritizing which elements should be tested first for maximum impact on lead generation or sales conversion.
Key Applications of AI-Driven Experimentation
- Pinpointing friction points by comparing drop-off data across funnel stages
- Generating hypothesis variations based on user intent clustering
- Continuously refining content blocks based on real-world engagement signals
Note: AI suggestions outperform manual A/B test planning by reducing time-to-insight by over 40%, according to internal benchmarking from conversion analytics platforms.
- Feed user interaction data into the AI model
- Receive prioritized suggestions for layout, copy, or CTA adjustments
- Deploy automatically generated test variants
- Review statistically significant uplift data
Element | AI Suggestion Type | Expected Impact |
---|---|---|
Headline | Sentiment and clarity optimization | +12% clickthrough |
CTA Button | Color and wording variants | +18% engagement |
Form Fields | Field count reduction | +22% completion rate |