Campaign Intelligence
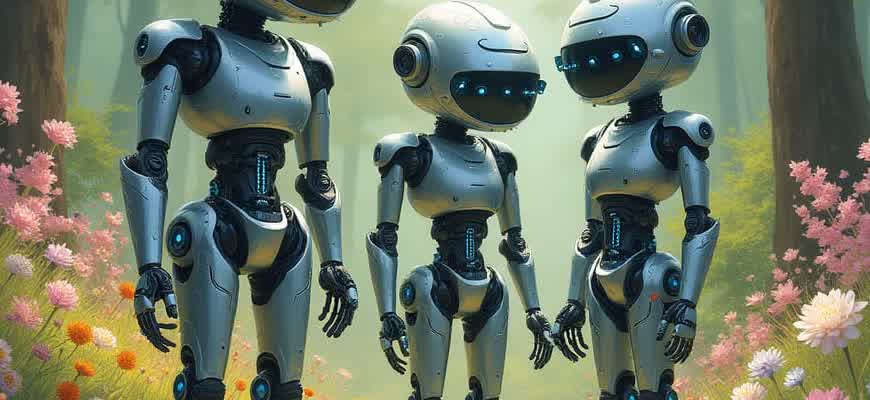
Campaign Intelligence refers to the data-driven strategies and tools used to enhance the effectiveness of marketing campaigns. It integrates various metrics and insights to optimize decision-making processes, improve targeting, and maximize overall campaign performance. By leveraging advanced analytics, businesses can better understand consumer behavior and anticipate trends.
Key Elements of Campaign Intelligence
- Data Collection: Gathering information from various channels such as social media, email, and website interactions.
- Analytics: Using statistical models to assess campaign performance and identify areas for improvement.
- Consumer Insights: Understanding the preferences and actions of the target audience to adjust strategies effectively.
- Real-time Monitoring: Continuously tracking campaign performance to make timely adjustments.
"Effective campaign intelligence enables businesses to adapt in real-time, ensuring that every marketing dollar spent brings optimal returns."
Important Metrics to Track
Metric | Purpose |
---|---|
Click-through Rate (CTR) | Measures the effectiveness of an ad or email in driving traffic. |
Conversion Rate | Indicates how well a campaign turns visitors into customers. |
Engagement Rate | Assesses how actively users interact with content, reflecting interest and relevancy. |
How to Leverage Data for Real-Time Campaign Adjustments
In today’s fast-paced marketing landscape, campaign success depends on the ability to make swift and data-driven adjustments. Real-time monitoring allows marketers to optimize campaigns on the fly, ensuring that they stay relevant and effective. By analyzing live data, teams can identify underperforming elements and pivot quickly to maintain optimal engagement levels.
Effective campaign optimization hinges on the timely collection and interpretation of key metrics. Utilizing tools that provide instant insights into audience behavior, conversion rates, and engagement can dramatically impact decision-making. Data analysis, when applied in real time, can refine targeting, messaging, and channel strategy to improve overall campaign performance.
Key Steps to Utilize Data for Real-Time Adjustments
- Monitor Engagement Metrics: Track clicks, conversions, and interactions to assess which elements resonate with the audience.
- Analyze Audience Segments: Use data to segment audiences based on behavior and demographics for tailored messaging.
- Test and Optimize Creatives: A/B testing in real time can determine which visuals and copy yield the best results.
Real-Time Data Sources for Optimization
- Website Analytics: Metrics such as page views, bounce rates, and conversion funnels offer critical insights.
- Social Media Insights: Engagement, reach, and sentiment analysis provide a snapshot of campaign impact.
- Ad Platforms: Platforms like Google Ads and Facebook Ads offer live performance data, enabling quick budget reallocations.
Key Insight: Real-time data is invaluable for adjusting campaign strategies and ensuring that marketing efforts are maximized to achieve immediate goals.
Example of Real-Time Data-Driven Decisions
Metric | Action |
---|---|
Low Click-Through Rate (CTR) | Revise Ad Copy and Visuals for better engagement |
High Bounce Rate | Optimize landing page to increase relevance and improve UX |
Underperforming Audience Segment | Adjust targeting to reach a more responsive demographic |
Establishing Effective Metrics for Campaign Performance
Setting precise performance metrics is crucial for tracking the success of any marketing campaign. Key Performance Indicators (KPIs) should reflect the specific objectives and desired outcomes of the campaign, allowing teams to measure progress and adjust strategies accordingly. When creating these indicators, it's essential to focus on both quantitative and qualitative data that align with business goals. The selection of KPIs will depend on factors such as target audience, marketing channels, and overall campaign strategy.
Effective KPIs must be SMART–Specific, Measurable, Achievable, Relevant, and Time-bound. This approach ensures that each metric is clear, actionable, and aligned with campaign objectives. Below are common metrics used to measure campaign success, broken down into categories based on focus areas.
Types of KPIs for Campaign Success
- Traffic Metrics: Focus on the volume and source of traffic to evaluate how well the campaign is generating awareness.
- Conversion Metrics: Measures the effectiveness of turning potential customers into actual ones.
- Engagement Metrics: Assesses the level of interaction and interest generated by the campaign.
- Revenue Metrics: Tracks the financial impact of the campaign, including ROI and cost-per-conversion.
Setting Up and Tracking KPIs
- Define Clear Objectives: Understand what success looks like for the campaign. Whether it’s increasing brand awareness, generating leads, or boosting sales, define what you want to achieve.
- Select Relevant KPIs: Choose metrics that directly correlate with the campaign’s goals. For example, if the objective is lead generation, focus on conversion rate and cost-per-lead.
- Implement Tracking Tools: Use analytics tools such as Google Analytics or CRM software to monitor KPI performance in real-time.
- Review and Optimize: Regularly assess performance data to identify areas for improvement and make necessary adjustments.
"KPIs are not just about measuring success, but also about identifying areas where improvements can be made to enhance campaign performance."
Sample KPI Table
Metric | Goal | Measurement Tool |
---|---|---|
Traffic Volume | Increase website visits by 30% | Google Analytics |
Conversion Rate | Achieve 5% conversion rate | CRM, Google Analytics |
Social Media Engagement | Boost engagement by 20% | Social Media Analytics |
Cost per Lead | Reduce cost per lead by 10% | CRM, Marketing Software |
Leveraging Predictive Analytics for Campaign Outcome Forecasting
Predictive analytics offers powerful tools for forecasting the success of marketing campaigns by analyzing historical data and identifying patterns that can influence future outcomes. By incorporating machine learning models and statistical techniques, campaign managers can predict various metrics, such as conversion rates, customer engagement, and ROI, with a higher degree of accuracy. This approach allows brands to optimize their strategies in real time and make data-driven decisions that can significantly improve the effectiveness of campaigns.
To implement predictive analytics effectively, campaign teams need to collect and analyze key performance indicators (KPIs) from previous campaigns. These insights can be used to forecast potential challenges, identify opportunities, and tailor future strategies. The ability to anticipate campaign results not only saves time but also enhances overall resource allocation and improves customer targeting.
Key Components of Predictive Analytics for Campaigns
- Data Collection: Gathering data from various touchpoints to create a robust dataset.
- Pattern Recognition: Identifying trends and correlations within the historical data.
- Modeling and Simulation: Building models to simulate potential outcomes under different scenarios.
- Optimization: Fine-tuning campaign strategies based on forecasted insights.
Predictive Models in Action
- Customer Segmentation: Identifying target audiences and predicting how different segments will respond to specific messages.
- Budget Allocation: Predicting which channels and tactics will yield the highest returns, allowing for more efficient resource distribution.
- Campaign Timing: Analyzing when to launch a campaign for maximum impact based on historical data and external factors.
Impact of Predictive Analytics on Campaign Success
By leveraging predictive analytics, companies can make informed decisions about campaign strategies, ultimately reducing risks and increasing the likelihood of success. This data-driven approach helps refine marketing tactics and fosters a more personalized experience for customers.
Example of Predictive Model Outcomes
Campaign Metric | Predicted Outcome | Actual Outcome |
---|---|---|
Customer Engagement | 15% increase | 17% increase |
Conversion Rate | 10% increase | 8% increase |
ROI | 25% growth | 27% growth |
Improving Customer Segmentation with Advanced Insights
In the modern digital landscape, understanding customer behavior and preferences has become increasingly complex. Traditional segmentation methods often rely on basic demographics, which may not fully capture the nuances of customer needs. By integrating advanced analytical tools and data sources, companies can gain a deeper, more actionable understanding of their customers. This enhanced approach allows businesses to segment their audience based on real-time, behavioral, and predictive data, moving beyond simple categorization to more personalized engagement.
Advanced insights unlock new dimensions of customer segmentation, enabling more precise targeting strategies. By leveraging machine learning, AI algorithms, and big data analytics, businesses can identify patterns and trends that would otherwise be difficult to detect. These insights not only help in creating refined customer segments but also allow for dynamic adjustments in marketing strategies, ensuring greater relevance and effectiveness in every interaction.
Key Techniques for Enhanced Segmentation
- Behavioral Data Analysis: Analyzing how customers interact with digital platforms, including browsing patterns, purchase history, and social media activity, provides a richer understanding of their preferences.
- Predictive Analytics: Using historical data and machine learning models, businesses can forecast future behavior, enabling proactive targeting of customers who are most likely to engage or convert.
- Sentiment Analysis: Assessing customer sentiment through text mining or social listening helps businesses understand how customers feel about their products or services.
- Dynamic Segmentation: Moving away from static, one-time segments to dynamic ones that update regularly based on evolving customer behavior.
"Advanced segmentation not only identifies who your customers are, but also predicts what they will do next, allowing businesses to tailor their marketing strategies effectively."
Table: Customer Segmentation Methods Comparison
Method | Description | Advantages |
---|---|---|
Demographic Segmentation | Grouping customers based on age, gender, income, etc. | Simplicity, easy to implement |
Behavioral Segmentation | Focusing on customer actions, like purchase behavior or website interactions. | Highly actionable, relevant for personalized marketing |
Psychographic Segmentation | Based on customer values, interests, or lifestyle. | Deep insights into motivations, ideal for loyalty programs |
Predictive Segmentation | Uses past behavior and machine learning to predict future actions. | Forward-looking, enables proactive engagement |
"Predictive segmentation is revolutionizing how companies understand their customers, enabling more anticipatory, relevant interactions."
Maximizing ROI Through Data-Driven Decision Making
To achieve higher returns on investment (ROI) in marketing campaigns, organizations must move beyond intuition-based strategies. The foundation of maximizing ROI lies in leveraging data insights to guide decisions, ensuring that resources are allocated efficiently and effectively. By adopting a data-centric approach, businesses can identify high-performing channels, optimize messaging, and ultimately reduce wastage in marketing spend.
Data-driven decision making provides a structured framework for analyzing campaign performance. By monitoring real-time metrics and aligning strategies with consumer behavior, brands can make more informed choices, thereby improving the impact of each marketing dollar spent. The goal is to transform raw data into actionable insights that directly contribute to better performance and measurable financial returns.
Key Strategies for Maximizing ROI
- Targeted Audience Segmentation: Analyzing customer data allows for segmentation, which helps to deliver personalized content, improving engagement and conversion rates.
- Predictive Analytics: Using historical data to forecast trends and behaviors ensures that marketing efforts are not reactive, but proactive and aligned with future consumer actions.
- Continuous Optimization: Regularly testing and adjusting campaign components based on real-time performance metrics maximizes the overall efficiency of campaigns.
Steps to Implement a Data-Driven Approach
- Data Collection: Gather relevant data from multiple sources, including CRM systems, social media, and web analytics platforms.
- Data Analysis: Analyze patterns and insights that inform decisions, focusing on conversion rates, cost per acquisition, and lifetime value.
- Test and Optimize: Continuously run A/B tests, monitor KPIs, and adjust campaigns based on findings to enhance ROI.
Example of ROI Maximization
Campaign Type | Cost per Click (CPC) | Conversion Rate | ROI |
---|---|---|---|
Social Media Ads | $1.50 | 3% | 4x |
Email Marketing | $0.75 | 5% | 6x |
Search Engine Ads | $2.00 | 4% | 5x |
"By aligning marketing strategies with data insights, organizations can ensure that each decision is backed by quantifiable evidence, ultimately improving ROI and overall performance."
Building a Scalable Framework for Ongoing Campaign Analysis
To ensure sustained success in marketing efforts, developing a scalable framework for continuous campaign evaluation is critical. The framework must not only accommodate current needs but also adapt to future requirements as the campaign evolves and new insights emerge. This requires a structured approach to data collection, analysis, and decision-making. The goal is to enable marketers to optimize campaigns in real time and adjust strategies based on meaningful metrics.
The foundation of such a framework lies in clear objectives, integrated tools, and streamlined processes for interpreting campaign performance. A successful structure will support ongoing data monitoring, identify trends quickly, and highlight areas for improvement. It allows for quick iteration while maintaining focus on long-term goals, ensuring that each campaign builds upon past learnings and improves over time.
Key Elements of a Scalable Framework
- Data Integration: Consolidate data from all touchpoints (social media, email, web analytics) into a unified dashboard for a clear overview.
- Performance Metrics: Establish key performance indicators (KPIs) to measure success against defined objectives.
- Real-Time Analysis: Implement tools that enable immediate analysis and adjustments during campaigns, preventing wasted resources.
- Feedback Loops: Create systems to gather feedback from all stakeholders and use this to improve future campaigns.
Steps to Implement the Framework
- Set Clear Goals: Begin with defining specific campaign objectives that are aligned with broader business targets.
- Choose the Right Tools: Select appropriate analytics and automation platforms that can handle data across multiple channels.
- Regular Data Evaluation: Schedule regular intervals for assessing campaign performance and tweaking strategies accordingly.
- Collaborative Feedback: Involve team members from different departments to ensure a holistic view of the campaign’s effectiveness.
Key Considerations
Aspect | Consideration |
---|---|
Scalability | Ensure that the framework can handle increased complexity as more campaigns are added. |
Automation | Incorporate automated processes for recurring analysis tasks to save time and reduce errors. |
Consistency | Maintain consistency in data collection and reporting to allow for accurate long-term comparisons. |
Building a scalable campaign analysis framework requires a balance between real-time agility and long-term strategy. It’s not just about reacting quickly, but using past data to forecast future needs and enhance overall effectiveness.