Integration in Nmr
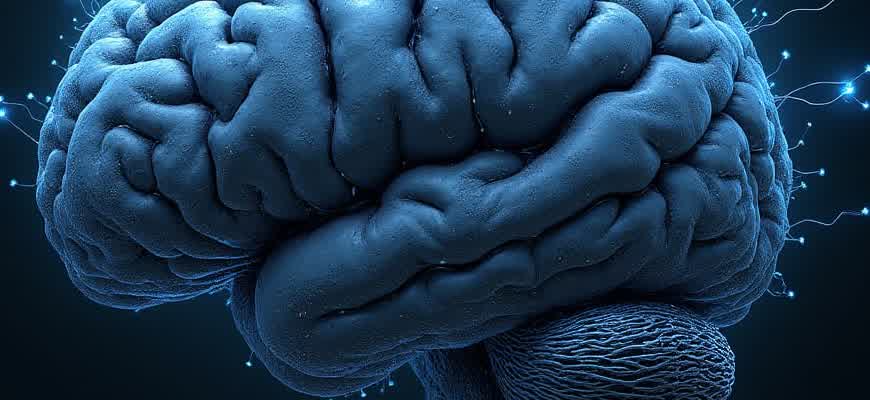
Proton signal analysis in magnetic resonance spectroscopy enables precise determination of molecular structure through relative area assessment under resonance peaks. This method provides critical insight into the number of nuclei contributing to each signal, thereby supporting structural elucidation and compound verification.
- Peak area directly correlates with the number of protons generating the signal.
- Integration values must be normalized against known reference signals.
- Consistent baseline and resolution are essential for reliable quantification.
Precise area calculation under resonance curves allows for direct comparison between different proton environments in a molecule.
To interpret proton signal contributions, the process involves numeric comparison of integrated values. These values are commonly represented as ratios reflecting proton quantities in different chemical environments.
- Acquire high-resolution spectrum with flat baseline.
- Select non-overlapping peaks for reference.
- Calculate relative intensities based on integrated area units.
Signal Position (ppm) | Integrated Value | Relative Proton Count |
---|---|---|
7.2 | 1.00 | 5 H (aromatic) |
3.5 | 0.40 | 2 H (methylene) |
1.2 | 0.60 | 3 H (methyl) |
Signal Area Quantification in Nuclear Magnetic Spectroscopy
In proton NMR spectroscopy, the area under each resonance peak is directly related to the number of hydrogen nuclei (protons) contributing to that signal. This property allows precise determination of the relative quantities of chemically distinct proton environments within a molecule. The process involves comparing the integrated signal intensities, which reflect the proportional presence of specific proton types.
The electronic integration output is displayed as step curves or numerical values, enabling analysts to derive molecular structures and confirm compound purity. When handled correctly, integration facilitates clear assignment of spectral components to specific structural fragments.
Integration Characteristics and Interpretation
Note: Integration reflects the relative number of equivalent protons, not their absolute count unless normalized.
- The height of the integration trace correlates with proton count.
- Accuracy requires fully relaxed nuclei; delays between scans must be sufficient.
- Solvent signals and impurities can distort the integration baseline.
- Acquire a fully relaxed 1H NMR spectrum.
- Select baseline regions free from overlapping peaks.
- Normalize integration to a known signal (e.g., methyl group = 3H).
Signal (ppm) | Integration Value | Proton Assignment |
---|---|---|
0.90 | 3.0 | CH₃ group |
2.45 | 2.0 | CH₂ adjacent to carbonyl |
7.25 | 5.0 | Aromatic protons |
Selecting Optimal Strategies for Accurate NMR Signal Quantification
Precise determination of component concentrations in NMR spectroscopy depends heavily on the integration technique applied to the acquired spectra. The chosen approach must consider baseline stability, peak overlap, and relaxation effects to avoid skewed results. Errors in signal area estimation directly affect the accuracy of quantitative analysis, especially in complex mixtures or low-concentration samples.
To achieve reliable integration, careful evaluation of spectral conditions and experimental parameters is essential. Different methods may yield varying levels of accuracy and reproducibility depending on the type of sample, resolution of the peaks, and software tools available. Below are key aspects to guide the selection process for the most appropriate integration routine.
Criteria for Method Selection
Note: Manual integration may offer precision in complex spectra but introduces subjectivity; automated methods are faster but require stringent calibration.
- Baseline Correction: Imperfect baselines distort peak area. Apply polynomial or spline corrections before integration.
- Peak Deconvolution: In overlapping multiplets, Gaussian or Lorentzian fitting provides more accurate individual areas than summation.
- Relaxation Delays: For nuclei with slow T1, ensure adequate delay times between scans to reach quantitative conditions.
- Calibrate with a known internal standard.
- Use full relaxation for all quantifiable nuclei.
- Select integration regions avoiding noise and sidebands.
Integration Method | Best Use Case | Limitations |
---|---|---|
Manual Integration | Complex, overlapping spectra | Time-consuming, user bias |
Automated Integration | Simple, well-resolved peaks | Prone to baseline errors |
Fitting-Based Integration | Highly overlapped peaks | Requires model assumptions |
Precision Techniques for Defining Integration Boundaries in NMR Analysis
In nuclear magnetic resonance spectroscopy, accurate quantification of proton environments depends on precisely defining the regions over which signal areas are measured. Misplaced boundaries can result in incorrect integrals, skewing the calculated ratios and misleading structural interpretations. Therefore, careful placement of integration limits is essential for reliable data analysis.
High-resolution spectra often present overlapping peaks, baseline noise, and artifacts that complicate boundary setting. Analysts must differentiate true signal from noise, isolate multiplets, and avoid including adjacent signals or shoulders. This process requires both spectral familiarity and methodical adjustment of integration cursors.
Steps to Optimize Integration Region Selection
- Zoom into the signal of interest to clearly view individual peak shapes.
- Set the left and right integration cursors just beyond the baseline return points of the multiplet.
- Check for adjacent or overlapping signals that may require deconvolution or selective integration.
- Adjust cursors to exclude sloping baselines or noise-induced distortions.
Tip: Always confirm that the integrated region returns to baseline on both sides. If it does not, re-evaluate the signal or use baseline correction tools.
When comparing multiple signals for relative quantification, ensure consistent application of boundary-setting criteria. Avoid integrating truncated peaks or asymmetrical multiplets unless applying corrections.
Signal Feature | Integration Limit Recommendation |
---|---|
Isolated singlet | Place cursors at full baseline return points on both sides |
Overlapping multiplet | Use peak deconvolution or manual integration with visual confirmation |
Broad signals (e.g., exchangeable protons) | Set broader limits, but verify baseline stability |
- Recalibrate the integral scale after each boundary adjustment.
- Validate integration results against known proton counts.
- Use internal standards when absolute quantification is required.
Managing Signal Overlap in Quantitative NMR Analysis
In proton NMR spectroscopy, the accurate quantification of proton environments becomes challenging when resonances are closely spaced or superimposed. This situation often arises in aromatic systems, complex aliphatic regions, or when sample purity is compromised. Without clear separation, direct area measurement under each signal can lead to significant integration errors.
To resolve this, analysts apply a combination of deconvolution techniques, spectral editing, and peak fitting algorithms. Manual strategies also involve strategic use of 2D NMR or selective decoupling experiments to isolate contributions from individual nuclei. Each method is chosen based on the specific overlap characteristics and the resolution capabilities of the spectrometer.
Common Strategies to Handle Overlapping Resonances
- Peak fitting software: Mathematical modeling to separate composite signals.
- Increased spectral resolution: Using higher field strength or optimized acquisition parameters.
- Selective experiments: TOCSY or COSY to spread signals over two dimensions.
When peaks are partially merged, a simple area-based integration will not reflect actual proton counts. Software-assisted resolution is essential to avoid misinterpretation.
- Identify the region with suspected overlap.
- Apply Gaussian or Lorentzian fitting to decompose the signal.
- Validate against known chemical structure and expected multiplicity.
Technique | Use Case | Limitations |
---|---|---|
Peak Deconvolution | Quantifying overlapping methyl and methylene signals | Requires accurate initial parameters |
2D NMR (e.g., COSY) | Separating J-coupled systems in complex spectra | More time-intensive, less quantitative |
High-field NMR | Improves resolution in crowded regions | Not always accessible |
Impact of Baseline Correction on NMR Signal Integration
Accurate quantification of signal areas in NMR spectra requires precise adjustment of the spectral baseline. When the baseline is distorted–due to factors like instrumental drift or solvent suppression artifacts–it directly affects the numerical integration, leading to unreliable concentration ratios among analytes.
Baseline distortions can lead to overestimation or underestimation of peak areas, especially in regions with low-intensity signals or overlapping multiplets. The application of mathematical correction algorithms is therefore critical to ensure consistent and reproducible integrals across the entire spectral range.
Common Correction Approaches and Their Effects
- Polynomial fitting removes broad baseline undulations.
- Whittaker smoothing filters out low-frequency baseline noise.
- Rolling ball or rubber band algorithms adapt to local variations in signal-free regions.
Note: Inconsistent baseline correction across different spectra may introduce systematic errors in quantitative analysis.
- Apply baseline correction before any peak integration.
- Validate correction by checking flatness in signal-free regions.
- Use control samples to verify quantitative reproducibility.
Correction Method | Suitable For | Risk |
---|---|---|
Polynomial Fitting | Simple baselines | Overfitting can distort signals |
Whittaker Smoother | Gradual baseline drift | Parameter-sensitive |
Rubber Band | Complex spectral backgrounds | May miss broad signal tails |
Using Software Tools for Automated NMR Integration
Modern NMR data analysis heavily relies on specialized programs that streamline signal quantification. These tools detect signal areas, correct baselines, and assign multiplets without manual input. Automation minimizes human error and significantly reduces processing time.
Applications such as MestReNova, TopSpin, and NUTS support automated peak integration by applying consistent algorithms across datasets. Their capabilities include digital Fourier transform, solvent suppression, and auto phase correction, ensuring reliable quantitative interpretation.
Core Features of Automated NMR Tools
- Baseline correction algorithms to eliminate drift
- Peak deconvolution and multiplet recognition
- Batch processing for multi-sample analysis
- Export of integration data in structured formats (CSV, XML)
Note: Accurate integration depends on high signal-to-noise ratios and proper referencing; automation cannot compensate for poor experimental setup.
Software | Integration Method | Output Format |
---|---|---|
MestReNova | Adaptive binning | CSV, PDF |
TopSpin | Region-specific auto-integration | XML, TXT |
NUTS | Manual and semi-auto | CSV |
- Import raw FID data
- Apply phase and baseline correction
- Run automatic integration routine
- Review and adjust integration limits if needed
Normalization Approaches for Signal Integration in NMR
Nuclear Magnetic Resonance (NMR) spectroscopy requires precise signal integration for accurate structural interpretation. However, signal intensity can vary due to several factors such as sample concentration, experimental conditions, or the physical properties of the sample. Normalization techniques are used to account for these variations and to facilitate direct comparisons between integrated signals in different spectra. These methods allow researchers to assess relative abundances and chemical environments of various nuclei in the sample.
Normalization plays a critical role in making integrated signals comparable by adjusting for discrepancies in signal intensity. The most common approaches involve mathematical scaling of the data to ensure that integration values reflect actual concentration or relative quantity accurately. The choice of normalization technique depends on the specific needs of the experiment and the type of comparison required.
Common Normalization Techniques
- Peak Height Normalization: This approach normalizes the signal by the height of the highest peak in the spectrum.
- Area Normalization: Signals are scaled based on the area under the curve of the integrated peaks, which accounts for the total signal intensity.
- Internal Standard Normalization: An internal reference compound with a known concentration is used to adjust the intensity of other signals.
Important Considerations for Signal Comparison
It is essential to ensure that the chosen normalization technique reflects the experimental conditions and accurately represents the relative quantities of different components in the sample.
Example Comparison of Different Normalization Techniques
Normalization Method | Advantages | Limitations |
---|---|---|
Peak Height Normalization | Simple and quick to apply. | Does not account for signal broadening or variations in peak shape. |
Area Normalization | Accurate for broad signals and varying peak shapes. | Can be computationally intensive for complex spectra. |
Internal Standard Normalization | Highly reliable if the standard is well-chosen. | Requires the presence of a consistent, well-defined internal standard. |
Integrating NMR Signals in Complex Mixtures
Nuclear Magnetic Resonance (NMR) spectroscopy is a powerful analytical tool used to study the composition of complex mixtures, including biological samples, natural products, and synthetic compounds. The main challenge when analyzing these mixtures is the effective integration of overlapping signals from various components, which can lead to incorrect quantification and interpretation of data. Accurate signal integration is crucial for obtaining reliable information about the relative concentrations of different compounds within a mixture.
The process of integrating NMR signals involves carefully distinguishing between peaks corresponding to individual components, even when they appear overlapped. This can be particularly challenging in mixtures where signals from different compounds resonate in similar frequency ranges. Advanced data processing techniques and software tools are often employed to resolve overlapping peaks and improve the accuracy of signal integration.
Approaches for Signal Integration in Complex Mixtures
- Peak Deconvolution: This technique uses algorithms to break down overlapping signals into individual peaks, making it easier to integrate each signal separately.
- Two-Dimensional NMR: 2D NMR techniques, such as COSY or HSQC, provide more detailed information by correlating signals from different nuclei, helping to resolve complex mixtures.
- Baseline Correction: Removing baseline distortions ensures that the integrated area of each signal reflects its true intensity, avoiding errors in quantification.
Common Challenges in Integration
- Signal Overlap: Compounds with similar chemical shifts can lead to peak overlap, which complicates the integration process.
- Conformational Variability: Some compounds may exhibit multiple conformations, causing broadening of peaks and further complicating signal integration.
- Matrix Effects: The presence of solvents or other components in the sample can affect the NMR signal and make integration difficult.
Important Considerations
Note: The choice of NMR technique and the conditions used for data acquisition significantly impact the quality of signal integration in complex mixtures. Proper experimental setup and sample preparation are essential to minimize complications during analysis.
Comparison of Integration Methods
Method | Advantages | Disadvantages |
---|---|---|
Peak Deconvolution | Accurate signal separation in complex mixtures | Requires advanced software and computational time |
Two-Dimensional NMR | Improved resolution and correlation of signals | Longer acquisition times |
Baseline Correction | Helps in accurate quantification | May not fully eliminate baseline distortions in all cases |
Validation of NMR Integration Results Using Internal Standards
In Nuclear Magnetic Resonance (NMR) spectroscopy, accurate quantification of peaks is crucial for structural and compositional analysis. One effective method for validating integration results is the use of internal standards. An internal standard is a compound that is added to the sample in a known concentration before the NMR experiment. This compound should be chemically similar to the analyte, ensuring that it behaves similarly under the same experimental conditions.
The primary benefit of using an internal standard is that it compensates for variations in instrument settings, sample preparation, and other experimental factors that can affect the integration results. By comparing the peak area or intensity of the analyte with that of the internal standard, it becomes possible to validate and correct the quantification process.
Steps for Validation
- Select a suitable internal standard: The compound should be inert and not interfere with the analyte's peaks.
- Ensure proper concentration: The concentration of the internal standard should be in the same order of magnitude as the analyte.
- Compare integration ratios: The ratio of peak areas or intensities between the analyte and internal standard is used to determine the sample’s composition.
Key Considerations
- Choice of internal standard: Select a compound that does not overlap with any signals of the target analyte.
- Quantitative accuracy: The internal standard method helps correct for systematic errors in integration.
- Reproducibility: Repeating the experiment with the same internal standard ensures consistent results.
Using an internal standard enhances the reliability of NMR quantification, especially when dealing with complex mixtures or samples where signal overlap is a concern.
Example of Calculation
Peak Area (Analyte) | Peak Area (Internal Standard) | Calculated Concentration |
---|---|---|
1200 | 1500 | 0.8 |
2500 | 2000 | 1.25 |